DMOEA- $\varepsilon \text{C}$ : Decomposition-Based Multiobjective Evolutionary Algorithm With the $\varepsilon $ -Constraint Framework
IEEE Transactions on Evolutionary Computation(2017)
摘要
Decomposition is an efficient and prevailing strategy for solving multiobjective optimization problems (MOPs). Its success has been witnessed by the multiobjective evolutionary algorithm MOEA/D and its variants. In decomposition-based methods, an MOP is decomposed into a number of scalar subproblems by using various scalarizing functions. Most decomposition schemes adopt the weighting method to construct scalarizing functions. In this paper, another classical generation method in the field of mathematical programming, that is the $ {varepsilon }$ -constraint method, is adopted for the multiobjective optimization. It selects one of the objectives as the main objective and converts other objectives into constraints. We incorporate the $ {varepsilon }$ -constraint method into the decomposition strategy and propose a new decomposition-based multiobjective evolutionary algorithm with the $ {varepsilon }$ -constraint framework (DMOEA- $ {varepsilon }text{C}$ ). It decomposes an MOP into a series of scalar constrained optimization subproblems by assigning each subproblem with an upper bound vector. These subproblems are optimized simultaneously by using information from neighboring subproblems. Besides, a main objective alternation strategy, a solution-to-subproblem matching procedure, and a subproblem-to-solution matching procedure are proposed to strike a balance between convergence and diversity. DMOEA- $ {varepsilon }text{C}$ is compared with a number of state-of-the-art multiobjective evolutionary algorithms. Experimental studies demonstrate that DMOEA- $ {varepsilon }text{C}$ outperforms or performs competitively against these algorithms on the majority of 34 continuous benchmark problems, and it also shows obvious advantages in solving multiobjective 0-1 knapsack problems.
更多查看译文
关键词
Linear programming,Upper bound,Pareto optimization,Evolutionary computation,Sociology
AI 理解论文
溯源树
样例
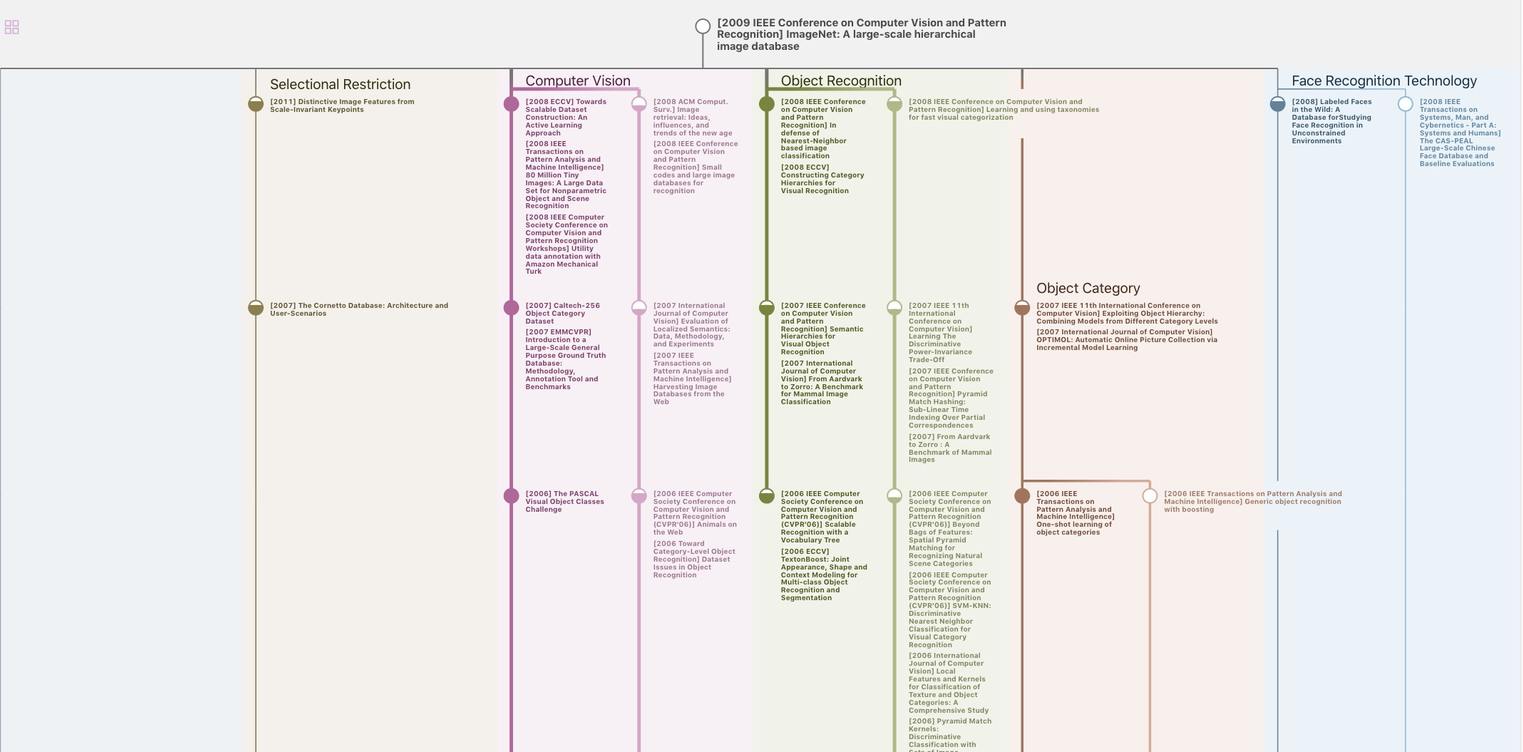
生成溯源树,研究论文发展脉络
Chat Paper
正在生成论文摘要