Feasibility analysis of genetically-encoded calcium indicators as a neural signal source for all-optical brain-machine interfaces
2017 8th International IEEE/EMBS Conference on Neural Engineering (NER)(2017)
摘要
Optical techniques such as two-photon (2p) calcium imaging have the potential to transform the way we interrogate neural circuits, both in the realm of basic neuroscience and in the development of brain-machine interfaces (BMIs). This may be possible by overcoming some of the limitations of electrophysiological methods. Here we ask if optical imaging signals, in particular 2p GCaMP6 calcium imaging signals, can benefit BMIs despite their relatively long activity-response time constants, low signal-to-noise ratios (SNRs), and slow acquisition frame rates. We employed motor cortical electrode array recordings as the basis for generating synthetic 2p GCaMP signals. We then decoded movement kinematics from these surrogate data using a state-of-the-art BMI decoder algorithm. We found that it was possible to decode the position and velocity of the hand from synthetic imaging signals. We quantified the decoder performance using standard mean squared errors (MSEs) and Pearson's correlation coefficient (r) measures. Decode quality varied considerably as a function of SNR and the frame rate of data acquisition. Future computational and experimental research is required to quantify SNR more accurately and to increase the imaging frame rate while maintaining high SNR, in order to improve all-optical BMI (o-BMI) performance. This study should help establish the feasibility and design space of o-BMIs.
更多查看译文
关键词
genetically-encoded calcium indicators,neural signal source,all-optical brain-machine interfaces,two-photon calcium imaging,neural circuits,electrophysiological methods,optical imaging signals,activity-response time constants,signal-to-noise ratios,acquisition frame rates,motor cortical electrode array recordings,movement kinematics,BMI decoder algorithm,mean squared errors,Pearson correlation coefficient,data acquisition,Ca
AI 理解论文
溯源树
样例
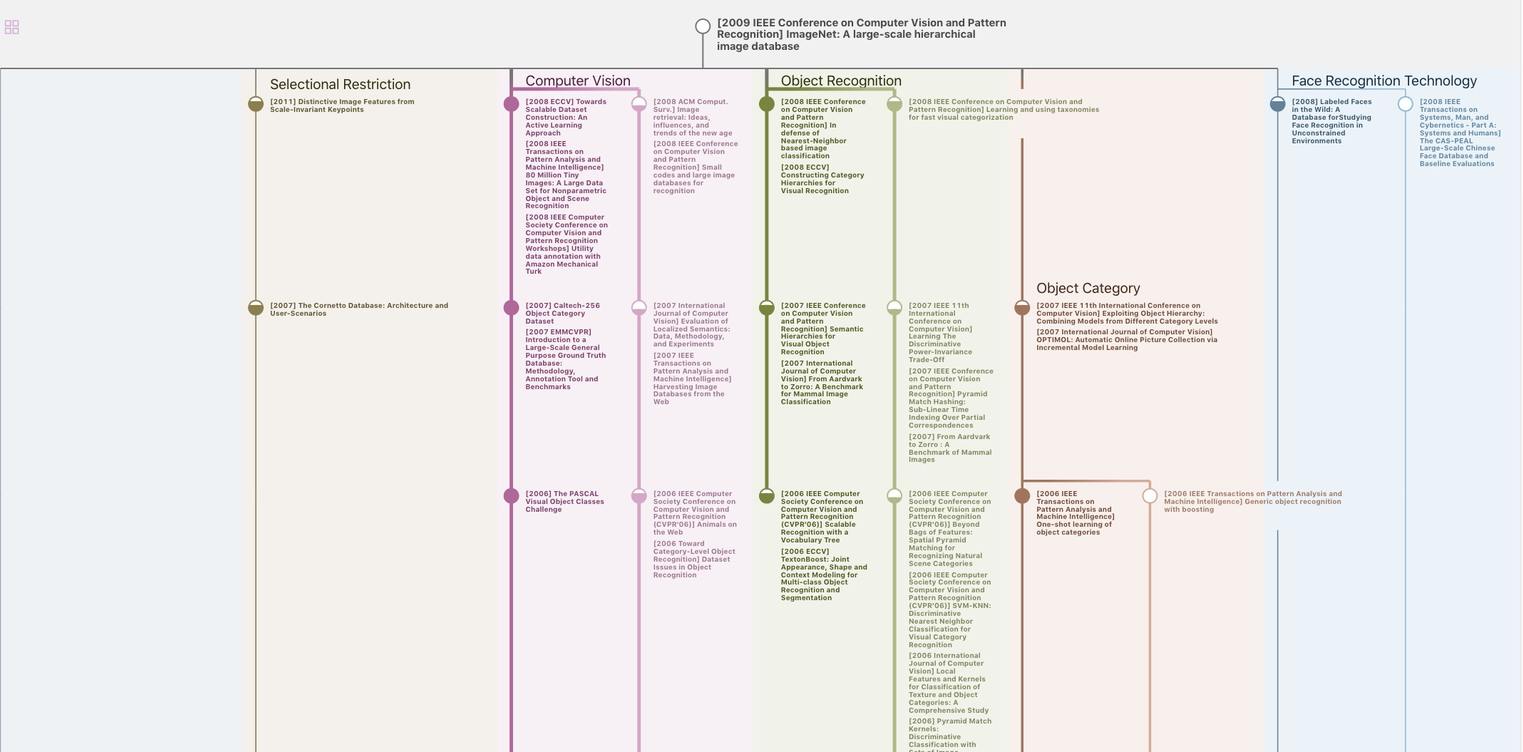
生成溯源树,研究论文发展脉络
Chat Paper
正在生成论文摘要