Prediction Of Hemorrhagic Growth Using Textural Pattern Analysis.
Stroke(2016)
摘要
Introduction: The subjective characterization of non-contrast CT “swirl sign” along with hematoma volume have been used to predict intracerebral hemorrhage (ICH) growth. As early assessment of patients with acute stroke symptoms can be used to optimize treatment, a more robust, quantitative biomarker set could improve patient care.Hypothesis: Quantification of ICH lesion texture from CT scans taken at less than 3 hours from onset could be used to predict hemorrhagic growth at 24 hours.Methods: Baseline and 24-hour CT scans on 71 ICH stroke subjects were obtained from the Virtual International Stroke Trials Archive (VISTA). Semi-automated segmentation of ICH was used to determine lesion volume. Based on treatment effects seen with hemostatic agents, a 6mL absolute increase was initially selected for the growth threshold. First order statistics of CT pixel values within the lesions along with spatial parameters extracted from grey-level co-occurrence matrices (GLCM) were computed, as well as baseline lesion volume, location (deep/lobar), number of connected components, and information about intraventricular hemorrhage. Linear support vector machines were used for classification with 10-fold cross-validation.Results: By the 24-hour scan, 19 subjects showed ICH growth and 52 were stable. In predicting growth based on the initial CT scan, the coefficient of variation and GLCM contrast had the highest sensitivities for discrimination. A combination of textural features provided a classification accuracy of 68% (sensitivity 65%, specificity 68%). Results for classifying “any growth” were comparable.Conclusions: Patterns noted in standard-of-care CT are indicative of an increased risk of hematoma expansion. Imaging biomarkers based on texture analysis can be used to classify patients and may aid in understanding mechanisms of ICH enlargement and acute treatment selection.
更多查看译文
关键词
hemorrhagic growth,textural pattern analysis
AI 理解论文
溯源树
样例
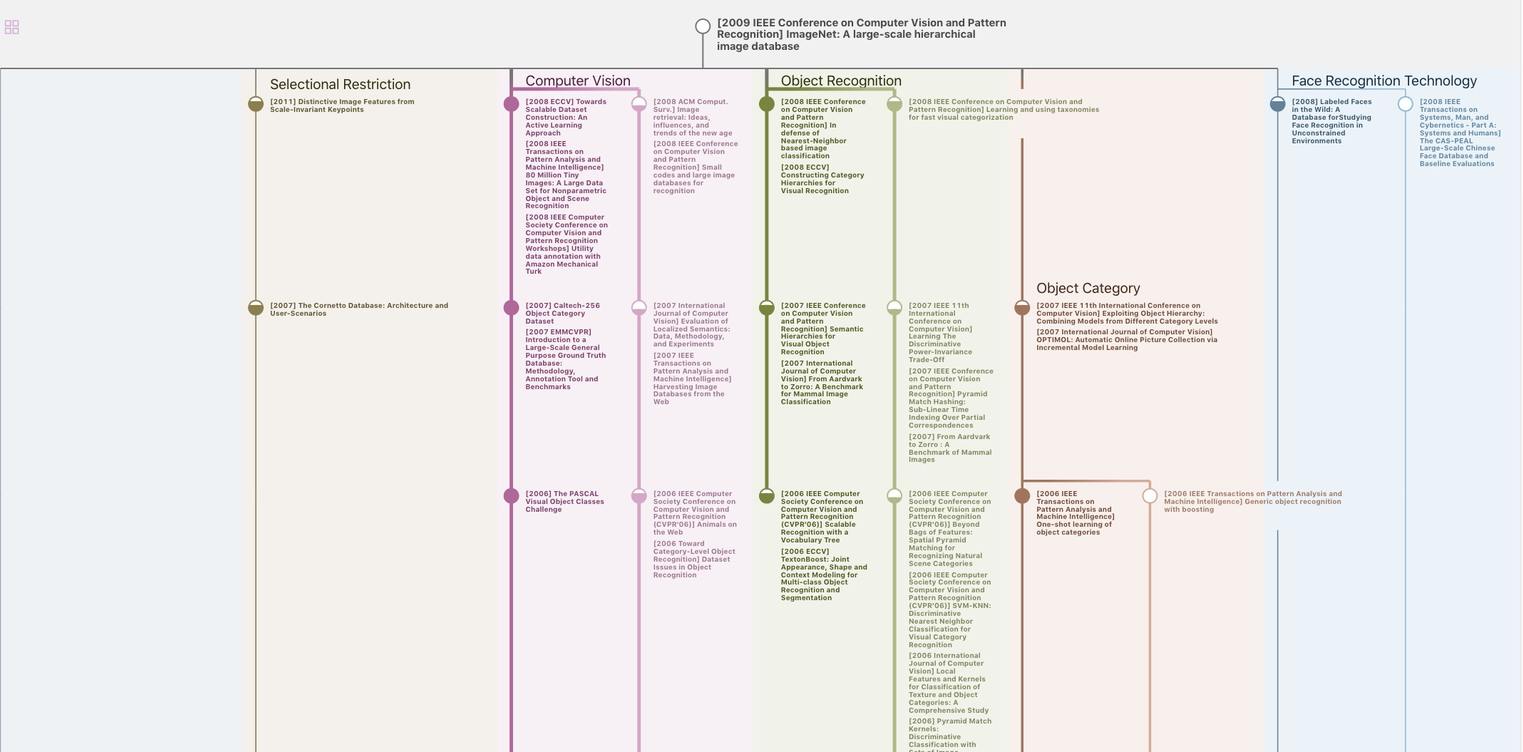
生成溯源树,研究论文发展脉络
Chat Paper
正在生成论文摘要