Associative memory framework for speech recognition: Adaptation of Hopfield network
2016 IEEE ANNUAL INDIA CONFERENCE (INDICON)(2016)
摘要
We examine the problem of how the Hopfield net associative memory framework can be adapted for speech recognition, by first identifying specific issues in such an adaptation, such as i) the problem of representing spectral feature vector sequences in the form of time normalized time-frequency (t-f) constellations, ii) the issue of limited capacity of the network when the temporal patterns are highly correlated as in the case of such temporal speech patterns and how it can be enhanced using a principal component transform with Hebbian learning or with the Pseudo-inverse learning rule, and iii) speaker-independent word recognition performance on a large number of test speakers (up to 400) by making the unsupervised Hopfield learning / retrieval perform as a classifier. We present early results on these issues and show the basic feasibility and merits of the Hopfield network formulation for speech recognition.
更多查看译文
关键词
Hopfield network,associative memory,speech recognition,t-f constellation,principal component based decorrelation,Hebbian rule,pseudo-inverse rule
AI 理解论文
溯源树
样例
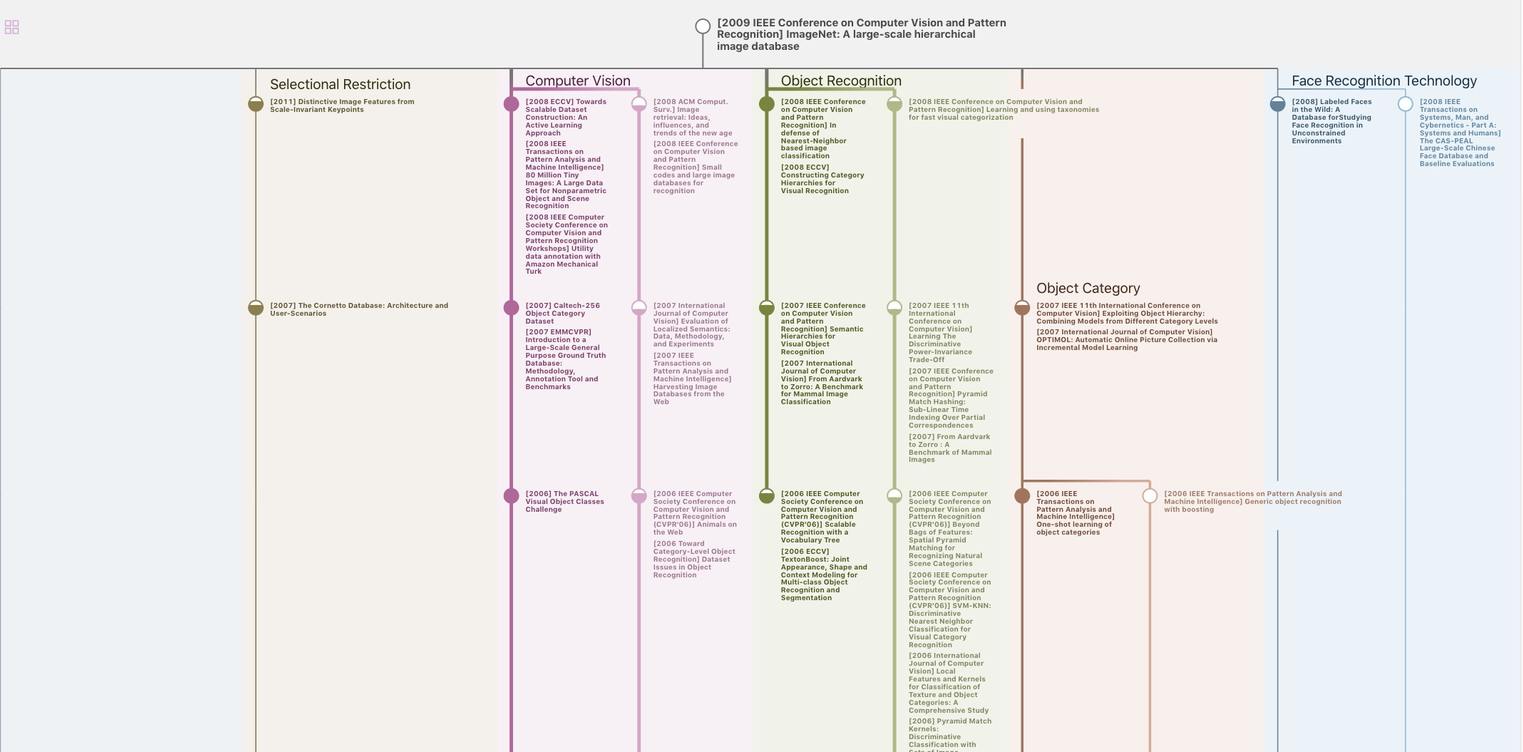
生成溯源树,研究论文发展脉络
Chat Paper
正在生成论文摘要