Bounded Real Lemma for Discrete-Time Stochastic Systems with Infinite Markov Jumps
Chinese Control Conference (CCC)(2016)
Shandong Univ Sci & Technol
Abstract
This paper is concerned with the disturbance attenuation property of discrete-time stochastic systems with infinite Markov jump and multiplicative noises. Above all, the finite horizon H ∞ performance is analyzed by means of a group of coupled difference Riccati equations, and a finite horizon bounded real lemma is obtained. Further, two versions of infinite horizon bounded real lemmas are presented for the considered systems in terms of coupled algebraic Riccati equations and linear matrix inequalities (LMI), respectively. As an application of the proposed bounded real lemma, a state-feedback H ∞ control design is provided.
MoreTranslated text
Key words
infinite Markov chain,bounded real lemma,H-infinity control
PDF
View via Publisher
AI Read Science
AI Summary
AI Summary is the key point extracted automatically understanding the full text of the paper, including the background, methods, results, conclusions, icons and other key content, so that you can get the outline of the paper at a glance.
Example
Background
Key content
Introduction
Methods
Results
Related work
Fund
Key content
- Pretraining has recently greatly promoted the development of natural language processing (NLP)
- We show that M6 outperforms the baselines in multimodal downstream tasks, and the large M6 with 10 parameters can reach a better performance
- We propose a method called M6 that is able to process information of multiple modalities and perform both single-modal and cross-modal understanding and generation
- The model is scaled to large model with 10 billion parameters with sophisticated deployment, and the 10 -parameter M6-large is the largest pretrained model in Chinese
- Experimental results show that our proposed M6 outperforms the baseline in a number of downstream tasks concerning both single modality and multiple modalities We will continue the pretraining of extremely large models by increasing data to explore the limit of its performance
Try using models to generate summary,it takes about 60s
Must-Reading Tree
Example
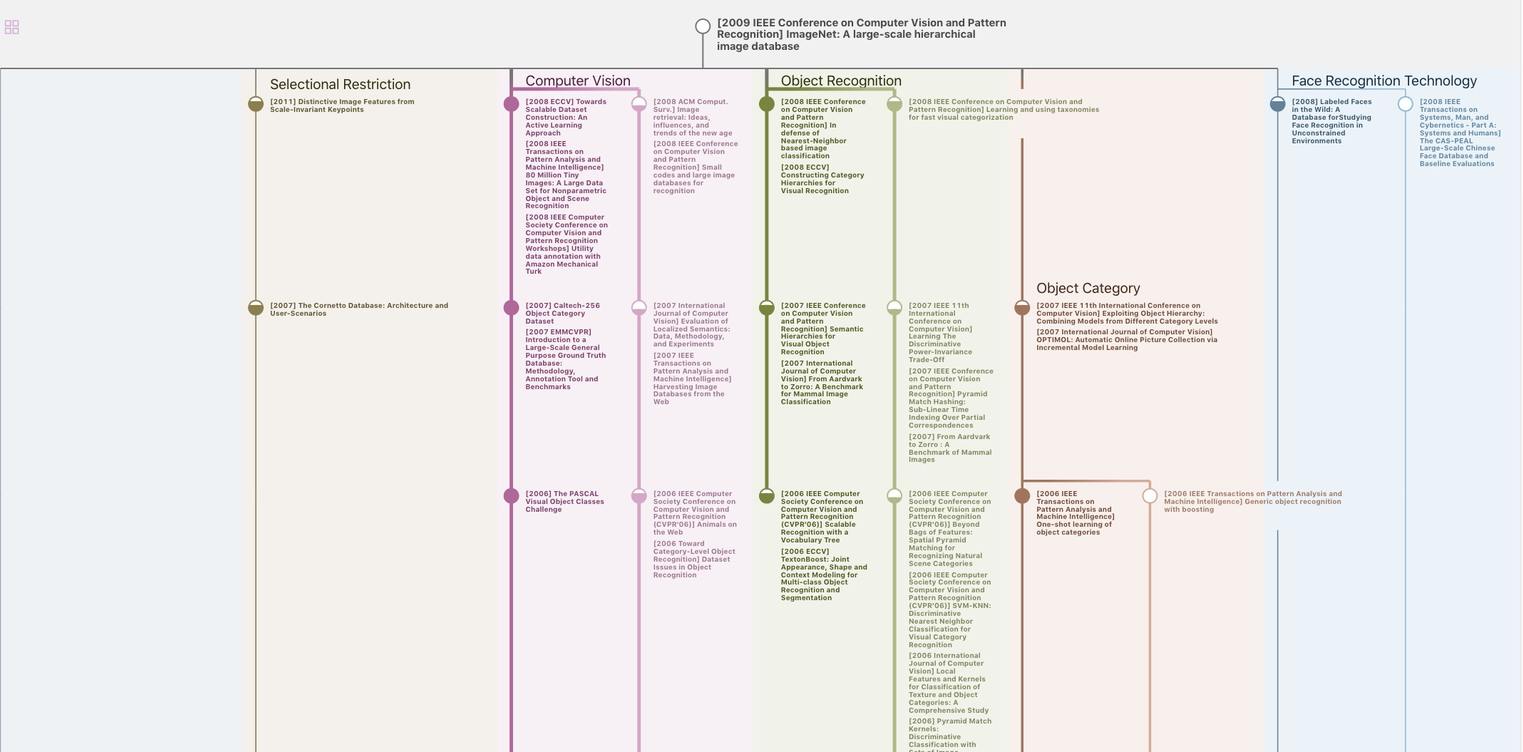
Generate MRT to find the research sequence of this paper
Related Papers
2018 37TH CHINESE CONTROL CONFERENCE (CCC) 2018
被引用0
$H_{2}/h_{\infty}$ Control for Continuous-Time Stochastic Systems with Infinite Markovian Jumps
2018 37th Chinese Control Conference (CCC) 2018
被引用0
PROCEEDINGS OF THE 36TH CHINESE CONTROL CONFERENCE (CCC 2017) 2017
被引用1
PROCEEDINGS OF THE 36TH CHINESE CONTROL CONFERENCE (CCC 2017) 2017
被引用21
Finite Horizon H2∕H∞ Control for SDEs with Infinite Markovian Jumps
Nonlinear Analysis Hybrid Systems 2019
被引用16
Robust Filtering for Nonlinear Stochastic Delay Systems with Multiplicative Noise and Jumps
PROCEEDINGS OF THE 2019 31ST CHINESE CONTROL AND DECISION CONFERENCE (CCDC 2019) 2019
被引用0
H∞ Consensus Control for Discrete-Time Stochastic Multi-agent Systems with Infinite Markov Jumps
IECON 2020 The 46th Annual Conference of the IEEE Industrial Electronics Society 2020
被引用2
2018 37th Chinese Control Conference (CCC) 2018
被引用0
International Journal of Control Automation and Systems 2022
被引用2
2018 37th Chinese Control Conference (CCC) 2018
被引用0
H∞ Control for a Class of Discrete-Time Infinite State Markov Jump Systems
Dynamical Systems and Control 2023
被引用0
Data Disclaimer
The page data are from open Internet sources, cooperative publishers and automatic analysis results through AI technology. We do not make any commitments and guarantees for the validity, accuracy, correctness, reliability, completeness and timeliness of the page data. If you have any questions, please contact us by email: report@aminer.cn
Chat Paper
GPU is busy, summary generation fails
Rerequest