A Novel Robust Principal Component Analysis Method for Image and Video Processing
Applied Mathematics(2016)
摘要
The research on the robust principal component analysis has been attracting much attention recently. Generally, the model assumes sparse noise and characterizes the error term by the λ 1 -norm. However, the sparse noise has clustering effect in practice so using a certain λ p -norm simply is not appropriate for modeling. In this paper, we propose a novel method based on sparse Bayesian learning principles and Markov random fields. The method is proved to be very effective for low-rank matrix recovery and contiguous outliers detection, by enforcing the low-rank constraint in a matrix factorization formulation and incorporating the contiguity prior as a sparsity constraint. The experiments on both synthetic data and some practical computer vision applications show that the novel method proposed in this paper is competitive when compared with other state-of-the-art methods.
更多查看译文
关键词
robust principal component analysis,sparse Bayesian learning,Markov random fields,matrix factorization,contiguity prior
AI 理解论文
溯源树
样例
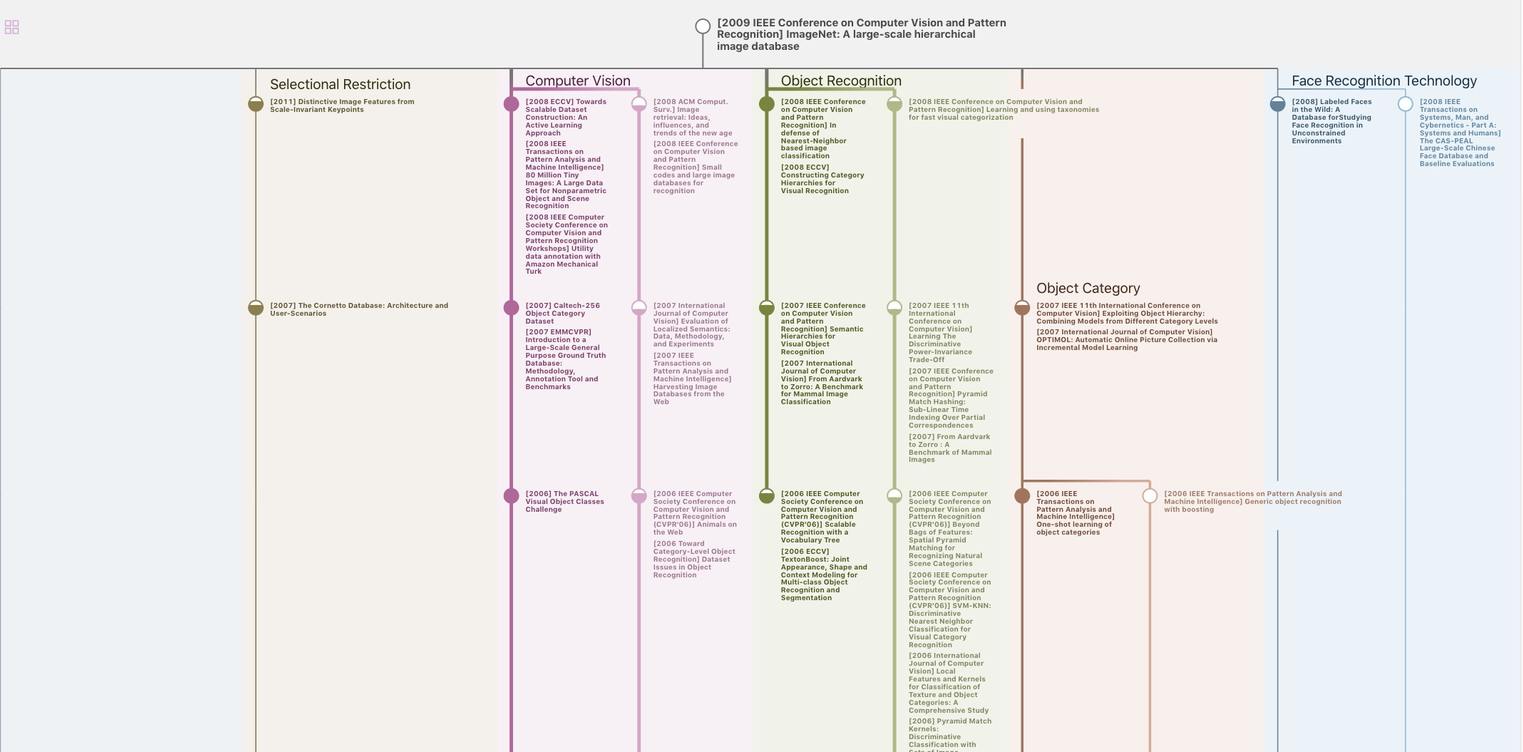
生成溯源树,研究论文发展脉络
Chat Paper
正在生成论文摘要