The VISCACHA Survey - I. Overview and First Results
Monthly Notices of the Royal Astronomical Society(2019)SCI 2区
Univ Sao Paulo | European Southern Observ | Instituto de Ciências Exatas | Univ Fed Rio Grande do Sul | Consejo Nacl Invest Cient & Tecn | AURA | Lab Nacl Astrofis | Ctr Fed Educ Tecnol Minas Gerais | Departamento de Ciências Exatas e Tecnológicas | Univ Fed ABC
Abstract
The VISCACHA(VIsible Soar photometry of star Clusters in tApii and Coxi HuguA) Survey is an ongoing project based on deep photometric observations of Magellanic Cloud star clusters, collected using the SOuthern Astrophysical Research (SOAR) telescope together with the SOAR Adaptive Module Imager. Since 2015 more than 200 h of telescope time were used to observe about 130 stellar clusters, most of them with low mass (M < 10(4) M-circle dot) and/or located in the outermost regions of the Large Magellanic Cloud and the Small Magellanic Cloud. With this high-quality data set, we homogeneously determine physical properties from statistical analysis of colour-magnitude diagrams, radial density profiles, luminosity functions, and mass functions. Ages, metallicities, reddening, distances, present-day masses, mass function slopes, and structural parameters for these clusters are derived and used as a proxy to investigate the interplay between the environment in the Magellanic Clouds and the evolution of such systems. In this first paper we present the VISCACHA Survey and its initial results, concerning the SMC clusters AM3, K37, HW20, and NGC 796 and the LMC ones KMHK228, OHSC3, SL576, SL61, and SL897, chosen to compose a representative subset of our cluster sample. The project's long-term goals and legacy to the community are also addressed.
MoreTranslated text
Key words
surveys,galaxies: interactions,Magellanic Clouds,galaxies: photometry,galaxies: star clusters: general
PDF
View via Publisher
AI Read Science
AI Summary
AI Summary is the key point extracted automatically understanding the full text of the paper, including the background, methods, results, conclusions, icons and other key content, so that you can get the outline of the paper at a glance.
Example
Background
Key content
Introduction
Methods
Results
Related work
Fund
Key content
- Pretraining has recently greatly promoted the development of natural language processing (NLP)
- We show that M6 outperforms the baselines in multimodal downstream tasks, and the large M6 with 10 parameters can reach a better performance
- We propose a method called M6 that is able to process information of multiple modalities and perform both single-modal and cross-modal understanding and generation
- The model is scaled to large model with 10 billion parameters with sophisticated deployment, and the 10 -parameter M6-large is the largest pretrained model in Chinese
- Experimental results show that our proposed M6 outperforms the baseline in a number of downstream tasks concerning both single modality and multiple modalities We will continue the pretraining of extremely large models by increasing data to explore the limit of its performance
Try using models to generate summary,it takes about 60s
Must-Reading Tree
Example
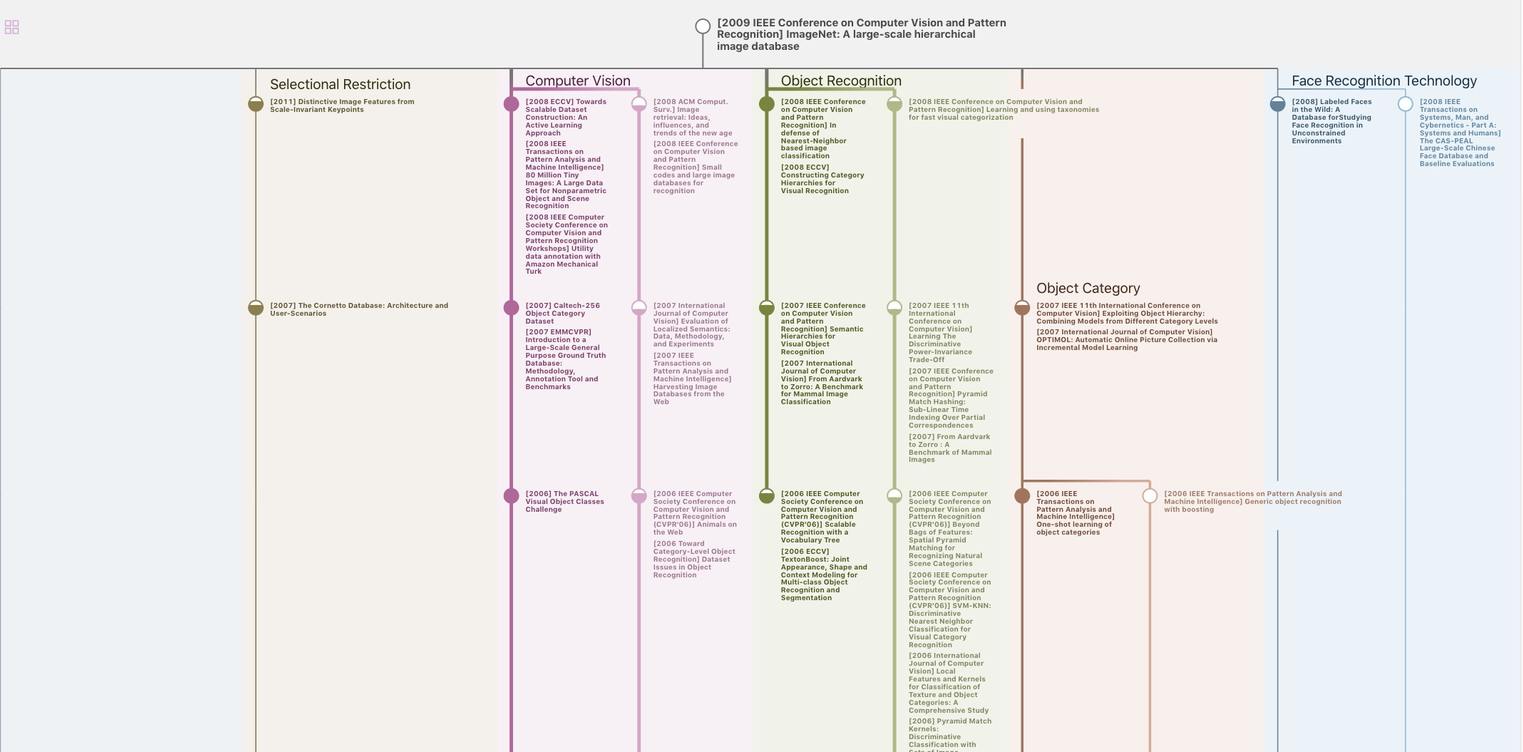
Generate MRT to find the research sequence of this paper
Related Papers
1991
被引用297 | 浏览
1998
被引用17913 | 浏览
2006
被引用124 | 浏览
2007
被引用40 | 浏览
2000
被引用566 | 浏览
1988
被引用126 | 浏览
2012
被引用146 | 浏览
2013
被引用395 | 浏览
2015
被引用21 | 浏览
2011
被引用46 | 浏览
2015
被引用31 | 浏览
2013
被引用26 | 浏览
2016
被引用16 | 浏览
Data Disclaimer
The page data are from open Internet sources, cooperative publishers and automatic analysis results through AI technology. We do not make any commitments and guarantees for the validity, accuracy, correctness, reliability, completeness and timeliness of the page data. If you have any questions, please contact us by email: report@aminer.cn
Chat Paper
GPU is busy, summary generation fails
Rerequest