Constructing Priors That Penalize the Complexity of Gaussian Random Fields
Journal of the American Statistical Association(2018)SCI 1区
NTNU | Univ Toronto | Univ Edinburgh | King Abdullah Univ Sci & Technol
Abstract
Priors are important for achieving proper posteriors with physicallymeaningful covariance structures for Gaussian random fields (GRFs) since thelikelihood typically only provides limited information about the covariancestructure under in-fill asymptotics. We extend the recent Penalised Complexityprior framework and develop a principled joint prior for the range and themarginal variance of one-dimensional, two-dimensional and three-dimensionalMatérn GRFs with fixed smoothness. The prior is weakly informative andpenalises complexity by shrinking the range towards infinity and the marginalvariance towards zero. We propose guidelines for selecting the hyperparameters,and a simulation study shows that the new prior provides a principledalternative to reference priors that can leverage prior knowledge to achieveshorter credible intervals while maintaining good coverage. We extend the prior to a non-stationary GRF parametrized through local rangesand marginal standard deviations, and introduce a scheme for selecting thehyperparameters based on the coverage of the parameters when fitting simulatedstationary data. The approach is applied to a dataset of annual precipitationin southern Norway and the scheme for selecting the hyperparameters leads toconcervative estimates of non-stationarity and improved predictive performanceover the stationary model.
MoreTranslated text
Key words
Convective Parameterization
PDF
View via Publisher
AI Read Science
AI Summary
AI Summary is the key point extracted automatically understanding the full text of the paper, including the background, methods, results, conclusions, icons and other key content, so that you can get the outline of the paper at a glance.
Example
Background
Key content
Introduction
Methods
Results
Related work
Fund
Key content
- Pretraining has recently greatly promoted the development of natural language processing (NLP)
- We show that M6 outperforms the baselines in multimodal downstream tasks, and the large M6 with 10 parameters can reach a better performance
- We propose a method called M6 that is able to process information of multiple modalities and perform both single-modal and cross-modal understanding and generation
- The model is scaled to large model with 10 billion parameters with sophisticated deployment, and the 10 -parameter M6-large is the largest pretrained model in Chinese
- Experimental results show that our proposed M6 outperforms the baseline in a number of downstream tasks concerning both single modality and multiple modalities We will continue the pretraining of extremely large models by increasing data to explore the limit of its performance
Try using models to generate summary,it takes about 60s
Must-Reading Tree
Example
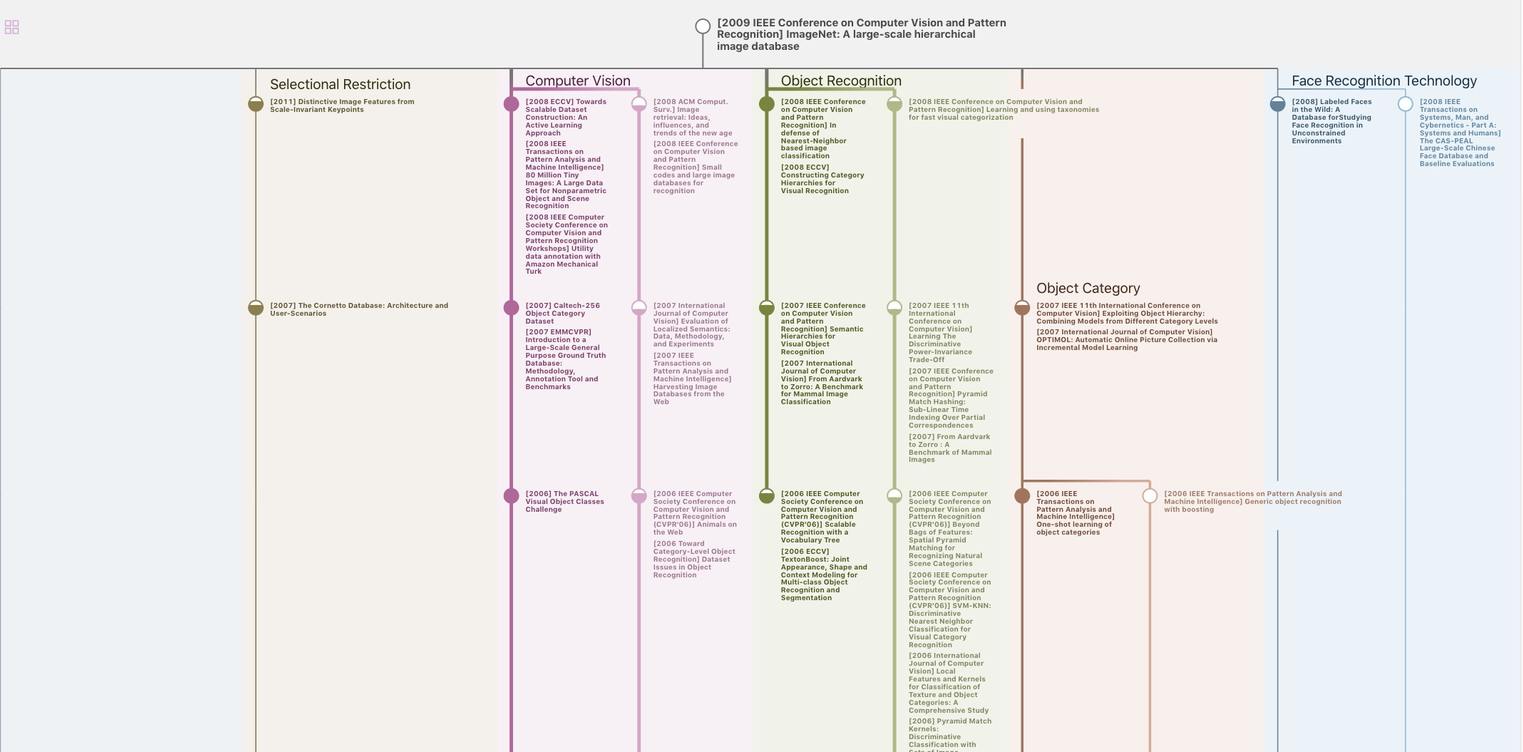
Generate MRT to find the research sequence of this paper
Related Papers
1991
被引用92 | 浏览
2009
被引用155 | 浏览
1987
被引用110 | 浏览
2009
被引用5888 | 浏览
2004
被引用449 | 浏览
2017
被引用657 | 浏览
2011
被引用1916 | 浏览
2016
被引用44 | 浏览
2012
被引用35 | 浏览
Data Disclaimer
The page data are from open Internet sources, cooperative publishers and automatic analysis results through AI technology. We do not make any commitments and guarantees for the validity, accuracy, correctness, reliability, completeness and timeliness of the page data. If you have any questions, please contact us by email: report@aminer.cn
Chat Paper
去 AI 文献库 对话