Detecting Autism by Analyzing a Simulated Social Interaction
ECML/PKDD (1)(2018)
摘要
Diagnosing autism spectrum conditions takes several hours by well-trained practitioners; therefore, standardized questionnaires are widely used for first-level screening. Questionnaires as a diagnostic tool, however, rely on self-reflection-which is typically impaired in individuals with autism spectrum condition. We develop an alternative screening mechanism in which subjects engage in a simulated social interaction. During this interaction, the subjects' voice, eye gaze, and facial expression are tracked, and features are extracted that serve as input to a predictive model. We find that a random-forest classifier on these features can detect autism spectrum condition accurately and functionally independently of diagnostic questionnaires. We also find that a regression model estimates the severity of the condition more accurately than the reference screening method.
更多查看译文
AI 理解论文
溯源树
样例
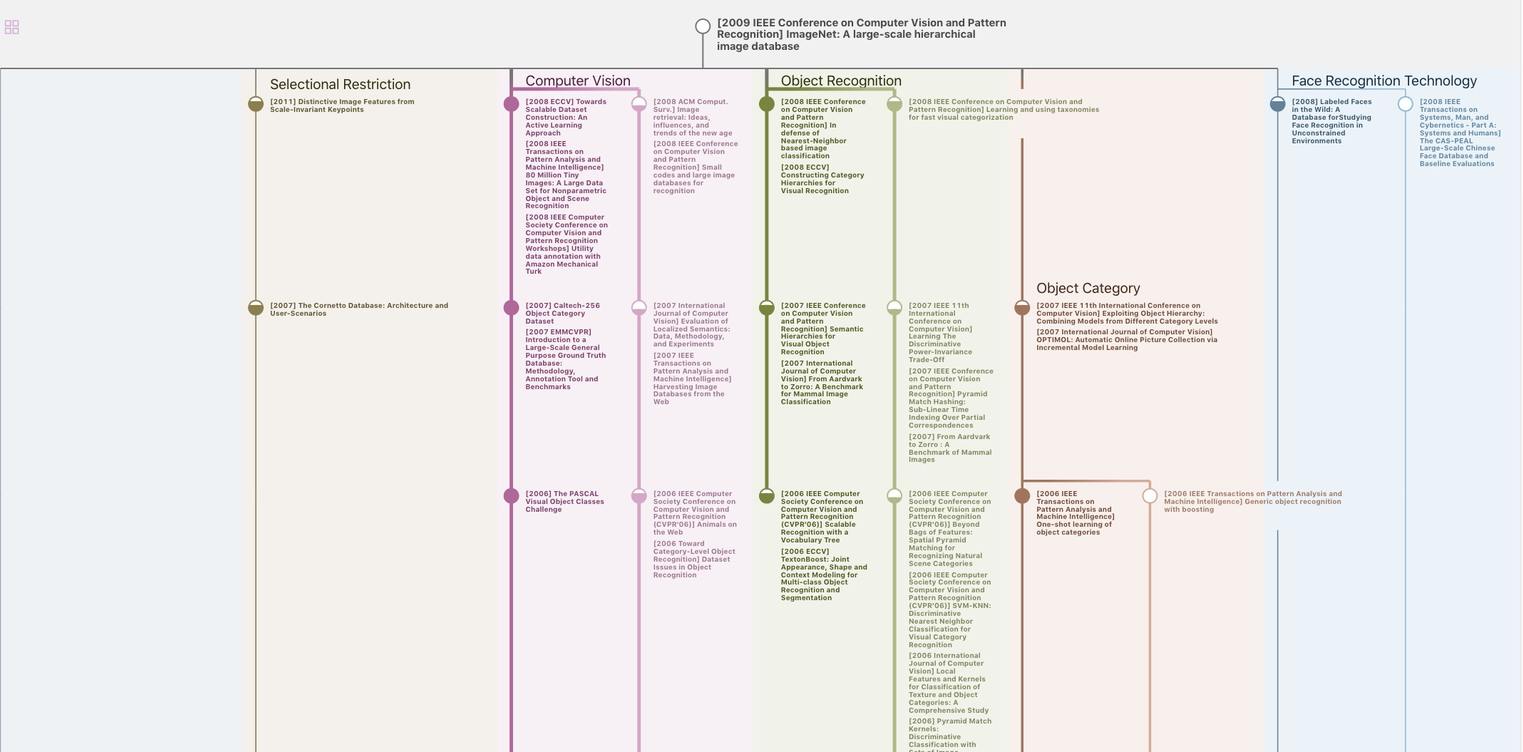
生成溯源树,研究论文发展脉络
Chat Paper
正在生成论文摘要