A Comparison Of Supervised Approaches For Process Pattern Recognition In Analog Semiconductor Wafer Test Data
2018 17TH IEEE INTERNATIONAL CONFERENCE ON MACHINE LEARNING AND APPLICATIONS (ICMLA)(2018)
摘要
The semiconductor industry is currently leveraging to exploit machine learning techniques to improve and automate the manufacturing process. An essential step is the wafer test, where each single device is measured electrically, resulting in an image of the wafer. Our work is based on the hypothesis that deviations of production processes can be detected via spatial patterns on these wafermaps. Supervised learning methods are one possibility to recognize such patterns in an automated way - however, the training sample size is very low. In our work, we present and compare several methods for multiclass classification, which can deal with this limitation: multiclass decision trees, as well as decomposition methods like round robin and error-correcting output coding (ECOC). As elementary classifiers, we compare binary decision trees and logistic regression using an elastic net regularization. The evaluation shows that the decomposition methods outperform the multiclass decision tree regarding both, accuracy and practical demands.
更多查看译文
关键词
multiclass classification, small training data, process pattern recognition, wafer test data
AI 理解论文
溯源树
样例
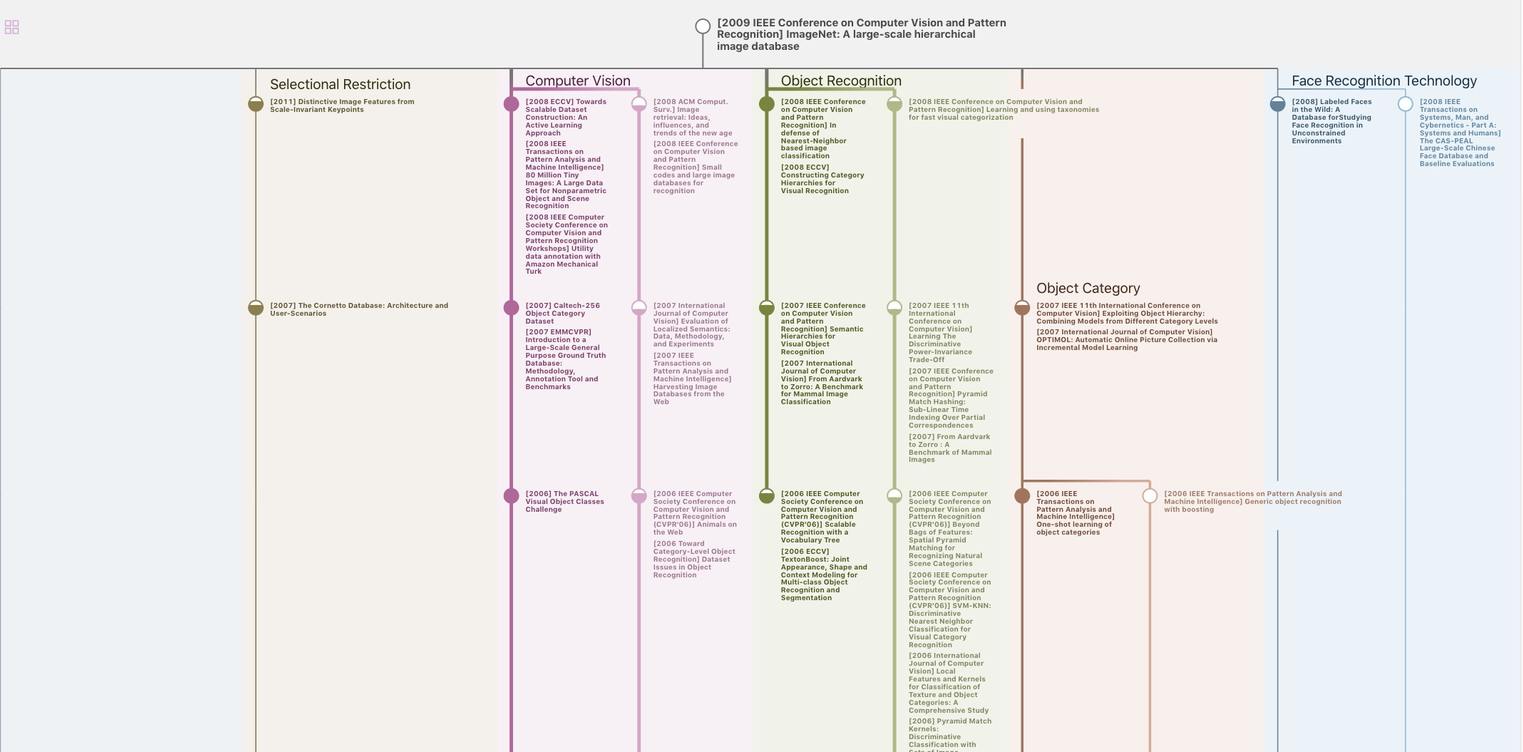
生成溯源树,研究论文发展脉络
Chat Paper
正在生成论文摘要