Degree Approximation-Based Fuzzy Partitioning Algorithm and Applications in Wheat Production Prediction
Symmetry(2018)
摘要
Recently, prediction modelling has become important in data analysis. In this paper, we propose a novel algorithm to analyze the past dataset of crop yields and predict future yields using regression-based approximation of time series fuzzy data. A framework-based algorithm, which we named DAbFP (data algorithm for degree approximation-based fuzzy partitioning), is proposed to forecast wheat yield production with fuzzy time series data. Specifically, time series data were fuzzified by the simple maximum-based generalized mean function. Different cases for prediction values were evaluated based on two-set interval-based partitioning to get accurate results. The novelty of the method lies in its ability to approximate a fuzzy relation for forecasting that provides lesser complexity and higher accuracy in linear, cubic, and quadratic order than the existing methods. A lesser complexity as compared to dynamic data approximation makes it easier to find the suitable de-fuzzification process and obtain accurate predicted values. The proposed algorithm is compared with the latest existing frameworks in terms of mean square error (MSE) and average forecasting error rate (AFER).
更多查看译文
关键词
wheat production prediction,fuzzy rules,time series,fuzzy regression
AI 理解论文
溯源树
样例
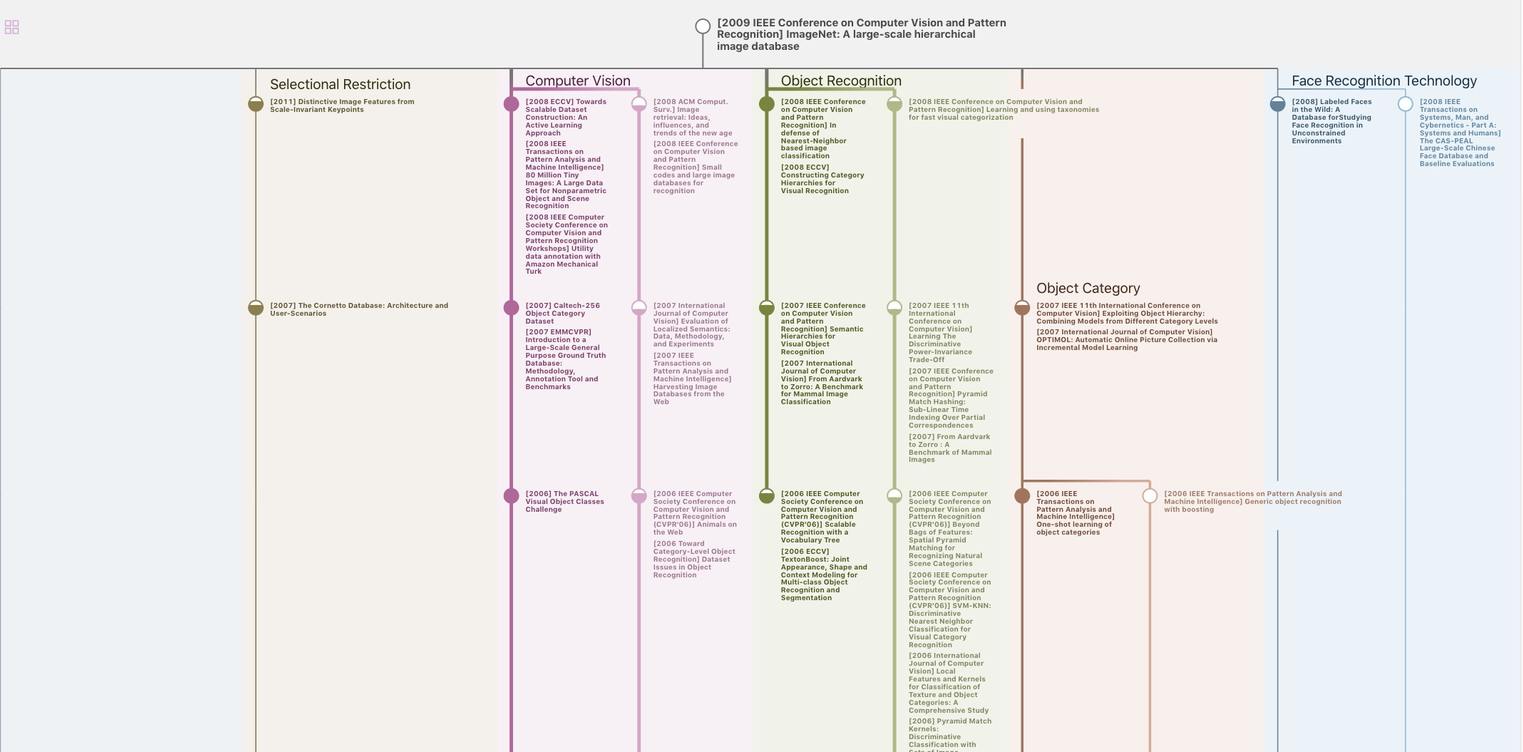
生成溯源树,研究论文发展脉络
Chat Paper
正在生成论文摘要