Driving-PASS: an Automatic Driving Performance Assessment System for Stroke Drivers Based on ANN and SVM
ICARCV(2018)
摘要
Although many stroke survivors are not fully capable of driving, they drive again without any formal assessment due to an absence of valid screening tools. This leads to an elevated risk of accidents. Although an on-road test is considered a standard assessment method for items relevant to actual driving, it may be dangerous to evaluate all stroke drivers with the on-road test. For safe pre-screening of unsuitable stroke drivers, we propose an automatic Driving Performance Assessment System for Stroke drivers (Driving-PASS). Driving-PASS aims to provide not only information about problematic driving assessment items but also a decision about fitness to drive. The problematic driving items are classified by abnormal classifiers while the decision item is determined by a decision classifier in Driving-PASS. For designing the system, we firstly propose a subjective assessment method consisting of ten assessment items and one decision item. And then, we propose an automated method of the subjective assessment method with a machine learning approach (i.e., ANN and SVM) by using assessment criteria from five expert's judgments. Evaluation results demonstrate that Driving-PASS automatically assess not only the ten assessment items (total average Accuracy of 90% and F1-score of 88%) but also the decision item (Accuracy of 93% and F1-score of 92%). We expect Driving-PASS provides analytical assessment results that can be used in driving rehabilitation programs and contributes to reducing the risk of vehicle accidents by pre-screening unsuitable stroke drivers with high accuracy and reliability.
更多查看译文
关键词
Driving-PASS,standard assessment method,actual driving,problematic driving assessment items,decision item,subjective assessment method,unsuitable stroke drivers,ANN,SVM,automatic driving performance assessment system for stroke drivers
AI 理解论文
溯源树
样例
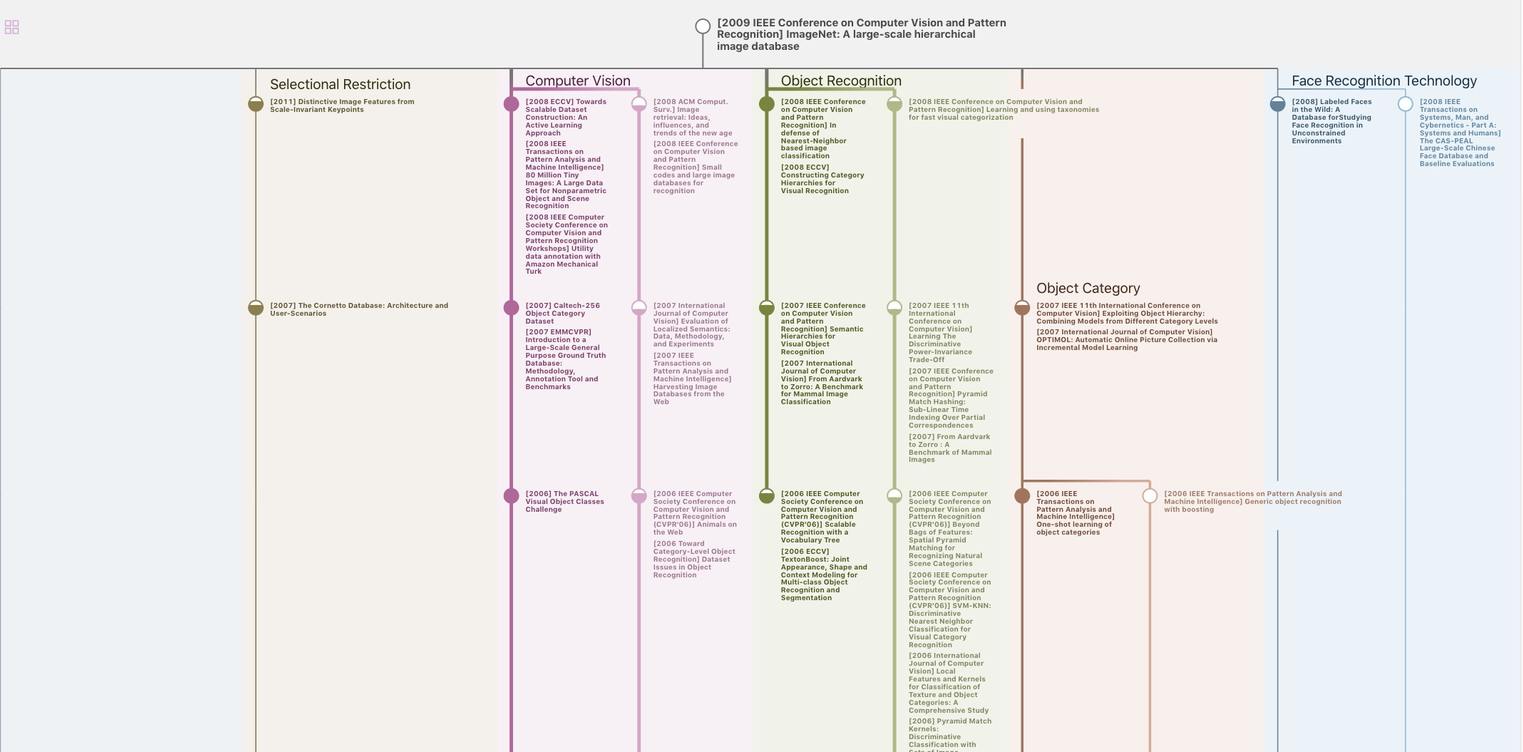
生成溯源树,研究论文发展脉络
Chat Paper
正在生成论文摘要