A Hybrid Instance-based Transfer Learning Method.
CoRR(2018)
摘要
In recent years, supervised machine learning models have demonstrated tremendous success in a variety of application domains. Despite the promising results, these successful models are data hungry and their performance relies heavily on the size of training data. However, in many healthcare applications it is difficult to collect sufficiently large training datasets. Transfer learning can help overcome this issue by transferring the knowledge from readily available datasets (source) to a new dataset (target). In this work, we propose a hybrid instance-based transfer learning method that outperforms a set of baselines including state-of-the-art instance-based transfer learning approaches. Our method uses a probabilistic weighting strategy to fuse information from the source domain to the model learned in the target domain. Our method is generic, applicable to multiple source domains, and robust with respect to negative transfer. We demonstrate the effectiveness of our approach through extensive experiments for two different applications.
更多查看译文
关键词
Transfer Learning,Meta-Learning,Semi-Supervised Learning,Representation Learning,Few-Shot Learning
AI 理解论文
溯源树
样例
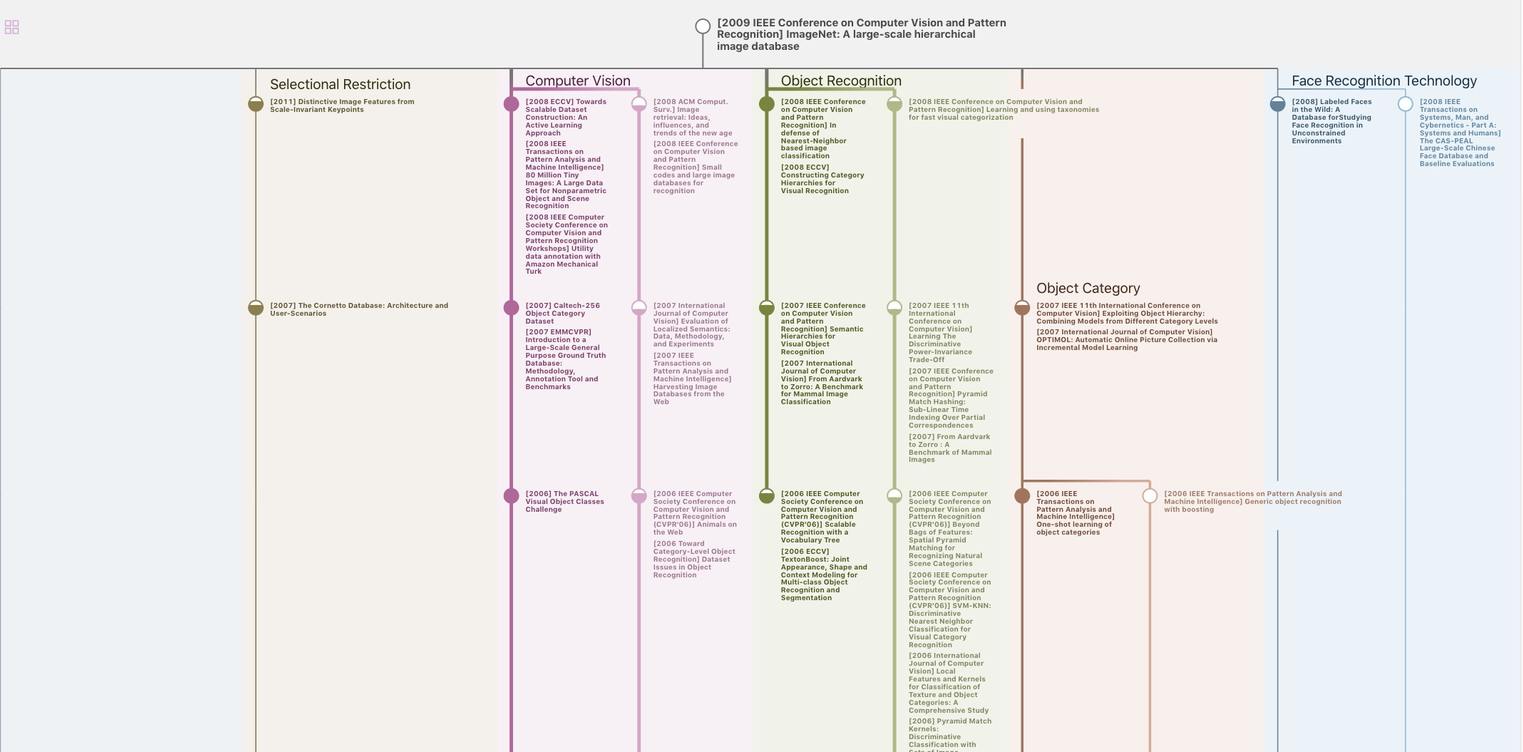
生成溯源树,研究论文发展脉络
Chat Paper
正在生成论文摘要