Successful Implementation of a Combined Learning Collaborative and Mentoring Intervention to Improve Neonatal Quality of Care in Rural Rwanda
BMC Health Services Research(2018)SCI 3区
Partners In HealthInshuti Mu Buzima | Widener University Center for Human and Sexuality Studies | Northwestern University Feinberg School of Medicine
Abstract
BackgroundGlobally, neonatal mortality remains high despite interventions known to reduce neonatal deaths. The All Babies Count (ABC) initiative was a comprehensive health systems strengthening intervention designed by Partners In Health in collaboration with the Rwanda Ministry of Health to improve neonatal care in rural public facilities. ABC included provision of training, essential equipment, and a quality improvement (QI) initiative which combined clinical and QI mentorship within a learning collaborative. We describe ABC implementation outcomes, including development of a QI change package.MethodsABC was implemented over 18months from 2013 to 2015 in two Rwandan districts of Kirehe and Southern Kayonza, serving approximately 500,000 people with 24 nurse-led health centers and 2 district hospitals. A process evaluation of ABC implementation and its impact on healthcare worker (HCW) attitudes and QI practice was done using program documents, standardized surveys and focus groups with facility QI team members attending ABC Learning Sessions. The Change Package was developed using mixed methods to identify projects with significant change according to quantitative indicators and qualitative feedback obtained during focus group discussions. Outcome measures included ABC implementation process measures, HCW-reported impact on attitudes and practice of QI, and resulting change package developed for antenatal care, delivery management and postnatal care.ResultsABC was implemented across all 26 facilities with an average of 0.76 mentorship visits/facility/month and 118 tested QI change ideas. HCWs reported a reduction in barriers to quality care delivery related to training (p=0.018); increased QI capacity (knowledge 37 to 89%, p<0.001); confidence (47 to 89%, p<0.001), QI leadership (59 to 91%, p<0.001); and peer-to-peer learning (37 to 66%, p=0.024). The final change package included 46 change ideas. Themes associated with higher impact changes included provision of mentorship and facility readiness support through equipment provision.ConclusionsABC provides a feasible model of an integrated approach to QI in rural Rwanda. This model resulted in increases in HCW and facility capacity to design and implement effective QI projects and facilitated peer-to-peer learning. ABC and the change package are being scaled to accelerate improvement in neonatal outcomes.
MoreTranslated text
Key words
Quality improvement,Health care system,Children,Quality culture,Developing countries
PDF
View via Publisher
AI Read Science
AI Summary
AI Summary is the key point extracted automatically understanding the full text of the paper, including the background, methods, results, conclusions, icons and other key content, so that you can get the outline of the paper at a glance.
Example
Background
Key content
Introduction
Methods
Results
Related work
Fund
Key content
- Pretraining has recently greatly promoted the development of natural language processing (NLP)
- We show that M6 outperforms the baselines in multimodal downstream tasks, and the large M6 with 10 parameters can reach a better performance
- We propose a method called M6 that is able to process information of multiple modalities and perform both single-modal and cross-modal understanding and generation
- The model is scaled to large model with 10 billion parameters with sophisticated deployment, and the 10 -parameter M6-large is the largest pretrained model in Chinese
- Experimental results show that our proposed M6 outperforms the baseline in a number of downstream tasks concerning both single modality and multiple modalities We will continue the pretraining of extremely large models by increasing data to explore the limit of its performance
Try using models to generate summary,it takes about 60s
Must-Reading Tree
Example
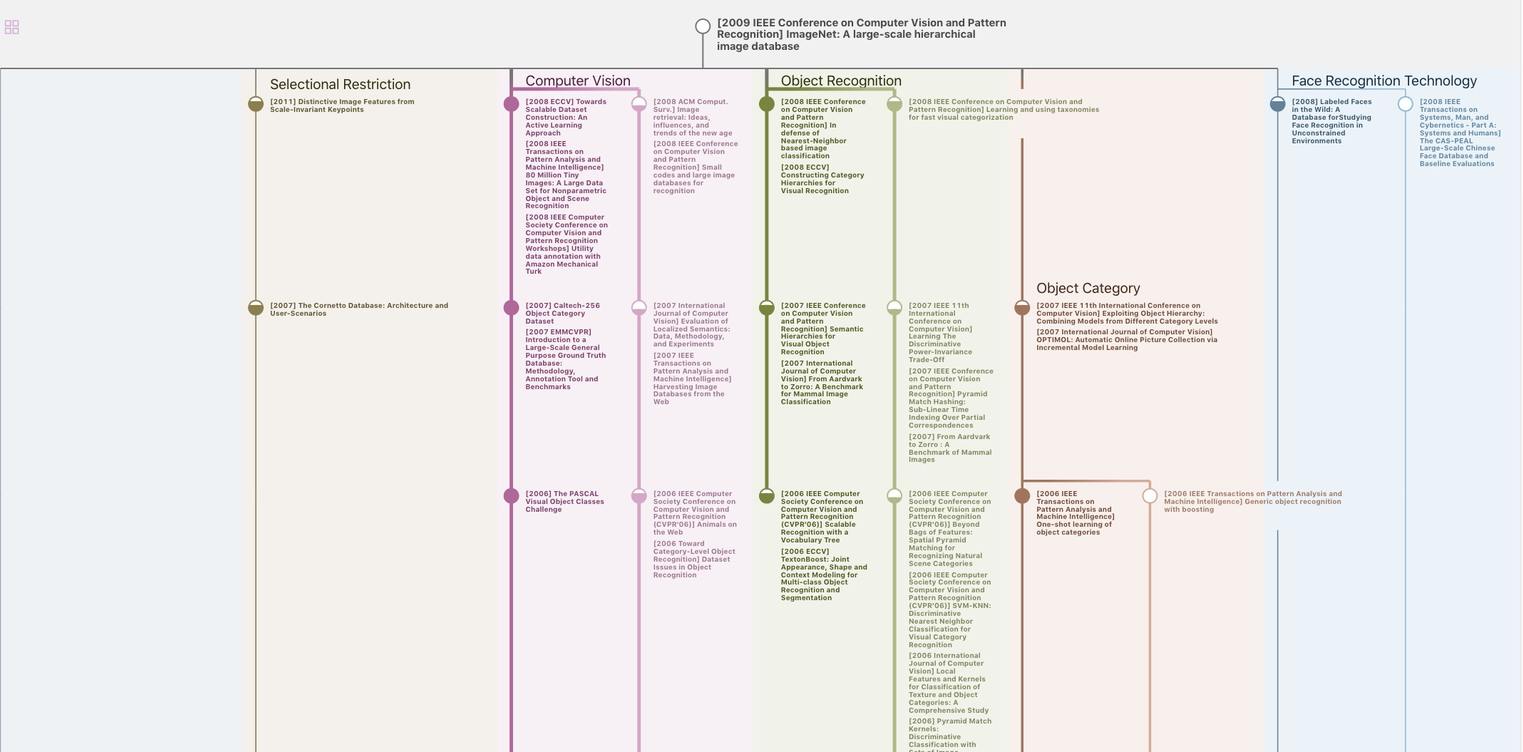
Generate MRT to find the research sequence of this paper
Related Papers
2011
被引用419 | 浏览
2011
被引用110 | 浏览
2012
被引用11 | 浏览
2008
被引用88 | 浏览
2008
被引用137 | 浏览
2012
被引用330 | 浏览
2013
被引用141 | 浏览
2014
被引用65 | 浏览
2016
被引用303 | 浏览
2015
被引用13 | 浏览
2017
被引用26 | 浏览
Data Disclaimer
The page data are from open Internet sources, cooperative publishers and automatic analysis results through AI technology. We do not make any commitments and guarantees for the validity, accuracy, correctness, reliability, completeness and timeliness of the page data. If you have any questions, please contact us by email: report@aminer.cn
Chat Paper
GPU is busy, summary generation fails
Rerequest