A Grouping Particle Swarm Optimizer with Personal-Best-Position Guidance for Large Scale Optimization.
IEEE/ACM Transactions on Computational Biology and Bioinformatics(2017)
摘要
Particle Swarm Optimization (PSO) is a popular algorithm which is widely investigated and well implemented in many areas. However, the canonical PSO does not perform well in population diversity maintenance so that usually leads to a premature convergence or local optima. To address this issue, we propose a variant of PSO named Grouping PSO with Personal-Best-Position ( Pbest) Guidance (GPSO-PG) which maintains the population diversity by preserving the diversity of exemplars. On one hand, we adopt uniform random allocation strategy to assign particles into different groups and in each group the losers will learn from the winner. On the other hand, we employ personal historical best position of each particle in social learning rather than the current global best particle. In this way, the exemplars diversity increases and the effect from the global best particle is eliminated. We test the proposed algorithm to the benchmarks in CEC 2008 and CEC 2010, which concern the large scale optimization problems (LSOPs). By comparing several current peer algorithms, GPSO-PG exhibits a competitive performance to maintain population diversity and obtains a satisfactory performance to the problems.
更多查看译文
关键词
Large-scale systems,Random processes,Statistics,Maintenance engineering,Particle swarm optimization,Optimization,Atmospheric measurements
AI 理解论文
溯源树
样例
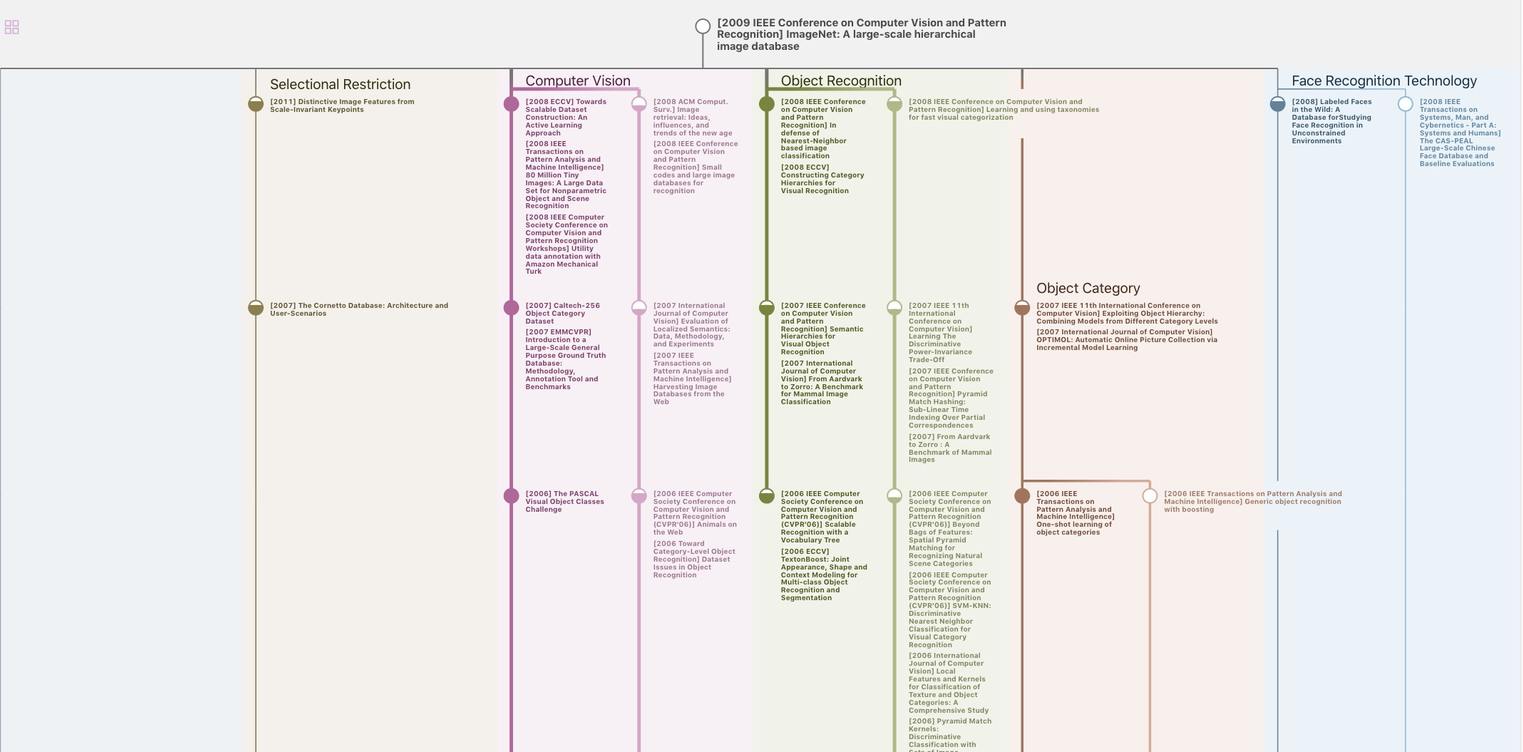
生成溯源树,研究论文发展脉络
Chat Paper
正在生成论文摘要