Disease Resistance and the Definition of Genetic Enhancement
Frontiers in genetics(2017)SCI 3区
Abstract
Recent gene editing experiments carried out in human embryos have raised the question of whether interventions like the introduction of a CCR5-Δ32 deletion, which could provide heritable resistance to HIV infection, ought to be considered enhancements. Many authors have used the term “enhancement” in different ways, some based on patients’ biomedical outcomes and others on their social context. These classifications are often considered overly imprecise. Nevertheless, the concept of “enhancement” could affect the ways in which these applications are regulated in different jurisdictions, the availability of coverage by insurers or public health care, and the force of public opinion in shaping future policy on gene editing. In order to ethically situate resistance to communicable disease with reference to other techniques, this article provides an overview of its similarities and differences with disease gene therapy in embryos, gene therapy in consenting adults, and vaccination. In discussing key ethical features of CCR5-Δ32 deletion (including its frequency in various populations, biological mechanism, benefits for individuals, and use in previous clinical trials) we offer some potential guideposts for the continuing discussion on how to classify “enhancements” in the age of CRISPR gene editing.
MoreTranslated text
Key words
enhancement,gene editing,gene therapy,CRISPR/Cas9,CCR5,HIV,disease resistance
PDF
View via Publisher
AI Read Science
AI Summary
AI Summary is the key point extracted automatically understanding the full text of the paper, including the background, methods, results, conclusions, icons and other key content, so that you can get the outline of the paper at a glance.
Example
Background
Key content
Introduction
Methods
Results
Related work
Fund
Key content
- Pretraining has recently greatly promoted the development of natural language processing (NLP)
- We show that M6 outperforms the baselines in multimodal downstream tasks, and the large M6 with 10 parameters can reach a better performance
- We propose a method called M6 that is able to process information of multiple modalities and perform both single-modal and cross-modal understanding and generation
- The model is scaled to large model with 10 billion parameters with sophisticated deployment, and the 10 -parameter M6-large is the largest pretrained model in Chinese
- Experimental results show that our proposed M6 outperforms the baseline in a number of downstream tasks concerning both single modality and multiple modalities We will continue the pretraining of extremely large models by increasing data to explore the limit of its performance
Try using models to generate summary,it takes about 60s
Must-Reading Tree
Example
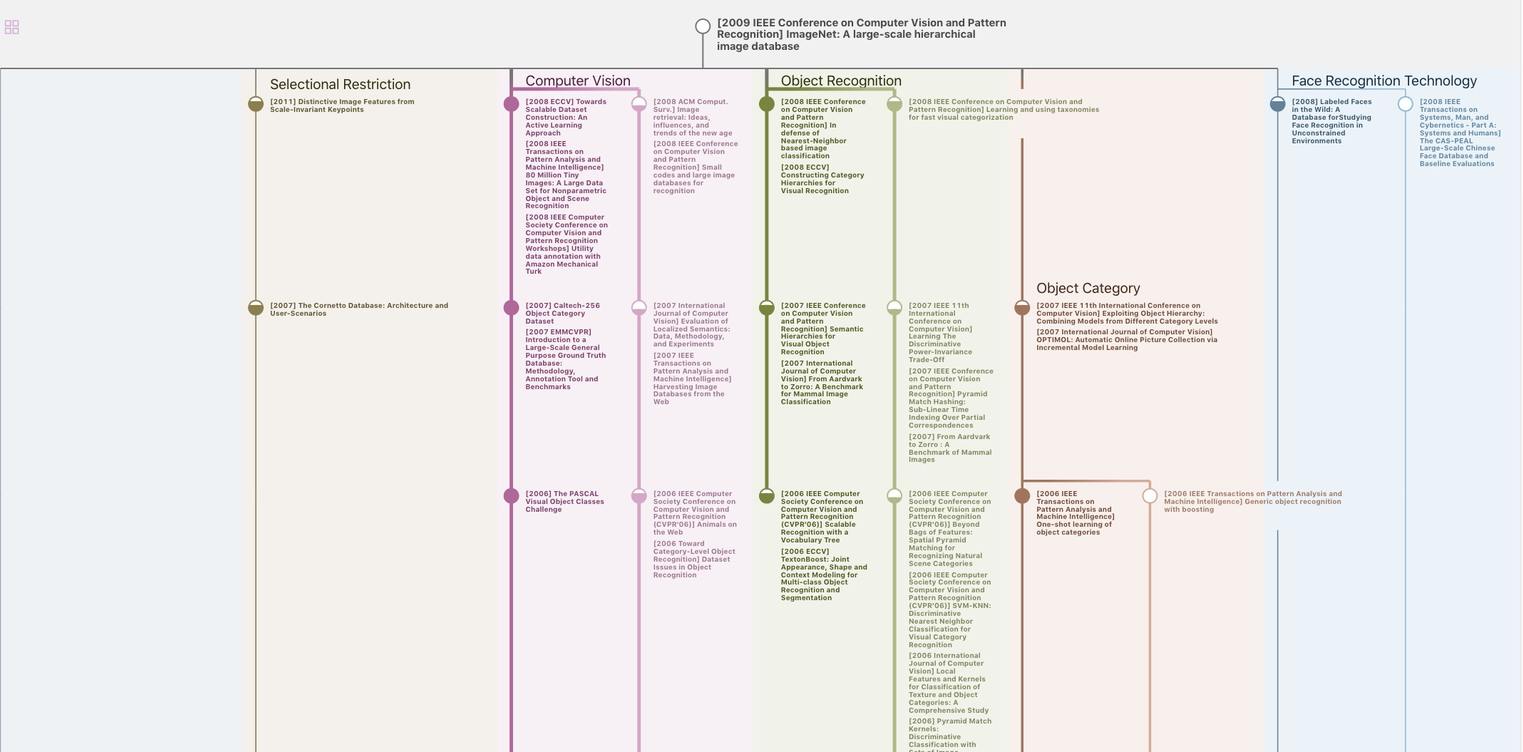
Generate MRT to find the research sequence of this paper
Related Papers
2003
被引用182 | 浏览
1998
被引用556 | 浏览
2017
被引用125 | 浏览
2009
被引用100 | 浏览
2014
被引用119 | 浏览
2014
被引用4 | 浏览
2017
被引用21 | 浏览
2007
被引用376 | 浏览
Data Disclaimer
The page data are from open Internet sources, cooperative publishers and automatic analysis results through AI technology. We do not make any commitments and guarantees for the validity, accuracy, correctness, reliability, completeness and timeliness of the page data. If you have any questions, please contact us by email: report@aminer.cn
Chat Paper
GPU is busy, summary generation fails
Rerequest