The Gene Ontology Resource: 20 Years and Still GOing Strong.
Nucleic Acids Research(2018)SCI 2区
Northwestern Univ | Univ Southern Calif | Texas A&M Univ | Univ Cambridge | Harvard Univ | Indiana Univ | GO EMBL EBI | Norwegian Univ Sci & Technol | Radboud Univ Nijmegen | UCL | Univ Maryland | EMBL EBI | Jackson Lab | Francis Crick Inst | Med Coll Wisconsin | NYU | Univ N Carolina | Stanford Univ | SIB | Phoenix Bioinformat | CALTECH | Univ Oregon
- Pretraining has recently greatly promoted the development of natural language processing (NLP)
- We show that M6 outperforms the baselines in multimodal downstream tasks, and the large M6 with 10 parameters can reach a better performance
- We propose a method called M6 that is able to process information of multiple modalities and perform both single-modal and cross-modal understanding and generation
- The model is scaled to large model with 10 billion parameters with sophisticated deployment, and the 10 -parameter M6-large is the largest pretrained model in Chinese
- Experimental results show that our proposed M6 outperforms the baseline in a number of downstream tasks concerning both single modality and multiple modalities We will continue the pretraining of extremely large models by increasing data to explore the limit of its performance
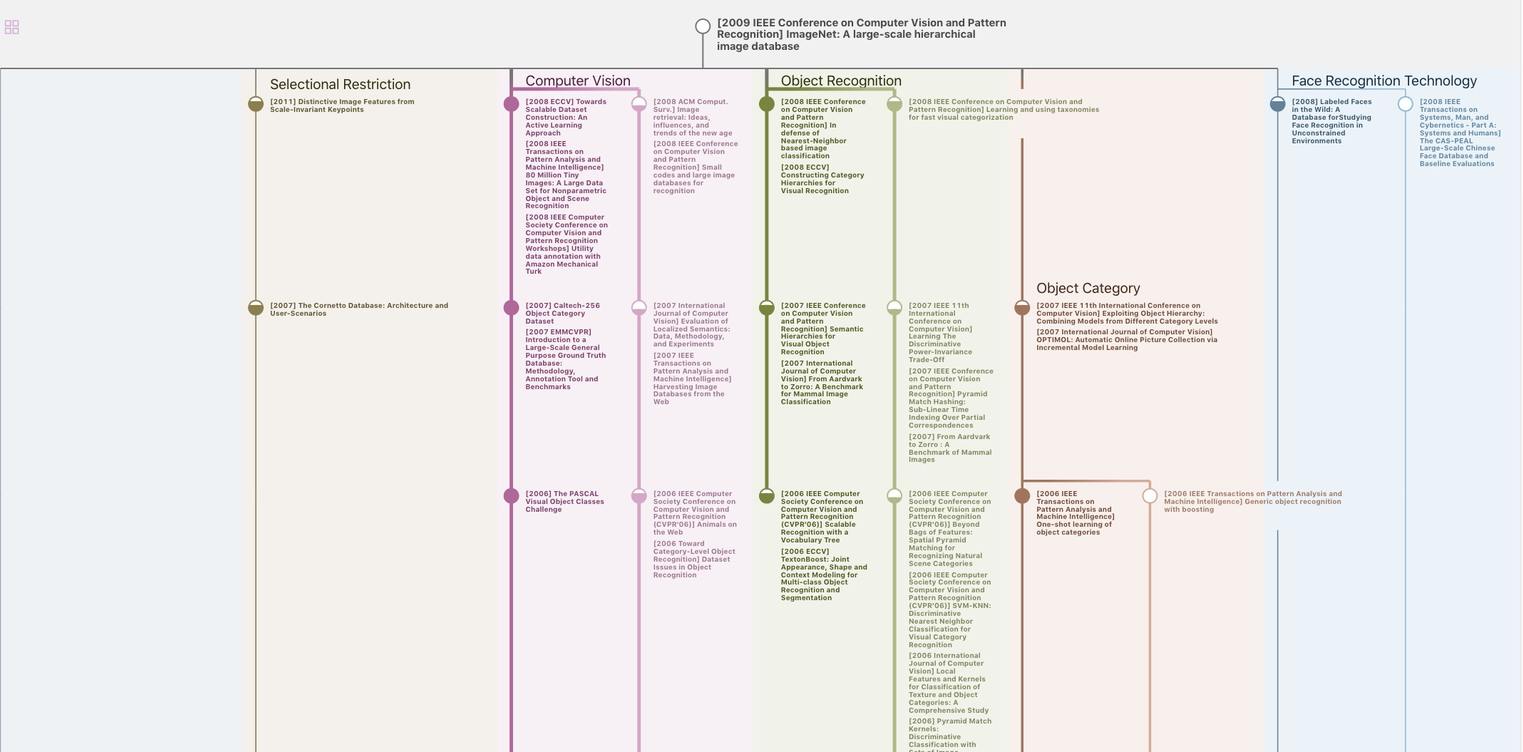
被引用18
A Genetic Interaction of NRXN2 with GABRE, SYT1 and CASK in Migraine Patients: a Case-Control Study
被引用5
被引用9
The Association of Immunosurveillance and Distant Metastases in Colorectal Cancer.
被引用8
Global Effects of Heroin Self-Administration on Microrna Expression Profiles in Rat Brain
被引用0
被引用7
Evolution of Protein Functional Annotation: Text Mining Study
被引用1
被引用9
被引用2
Predicted Mouse Interactome and Network-Based Interpretation of Differentially Expressed Genes.
被引用0
The Cerna Crosstalk Between Mrnas and Lncrnas in Diabetes Myocardial Infarction.
被引用2
被引用0
An Automatic Integrative Method for Learning Interpretable Communities of Biological Pathways
被引用1
被引用6
被引用1
被引用14
被引用19
被引用1
被引用4
被引用5
被引用2
被引用5
被引用3