LigVoxel: Inpainting binding pockets using 3D-convolutional neural networks.
BIOINFORMATICS(2019)
摘要
Motivation: Structure-based drug discovery methods exploit protein structural information to design small molecules binding to given protein pockets. This work proposes a purely data driven, structure-based approach for imaging ligands as spatial fields in target protein pockets. We use an end-to-end deep learning framework trained on experimental protein-ligand complexes with the intention of mimicking a chemist's intuition at manually placing atoms when designing a new compound. We show that these models can generate spatial images of ligand chemical properties like occupancy, aromaticity and donor-acceptor matching the protein pocket. Results: The predicted fields considerably overlap with those of unseen ligands bound to the target pocket. Maximization of the overlap between the predicted fields and a given ligand on the Astex diverse set recovers the original ligand crystal poses in 70 out of 85 cases within a threshold of 2 angstrom RMSD. We expect that these models can be used for guiding structure-based drug discovery approaches.
更多查看译文
关键词
binding,ligvoxel,pockets,d-convolutional
AI 理解论文
溯源树
样例
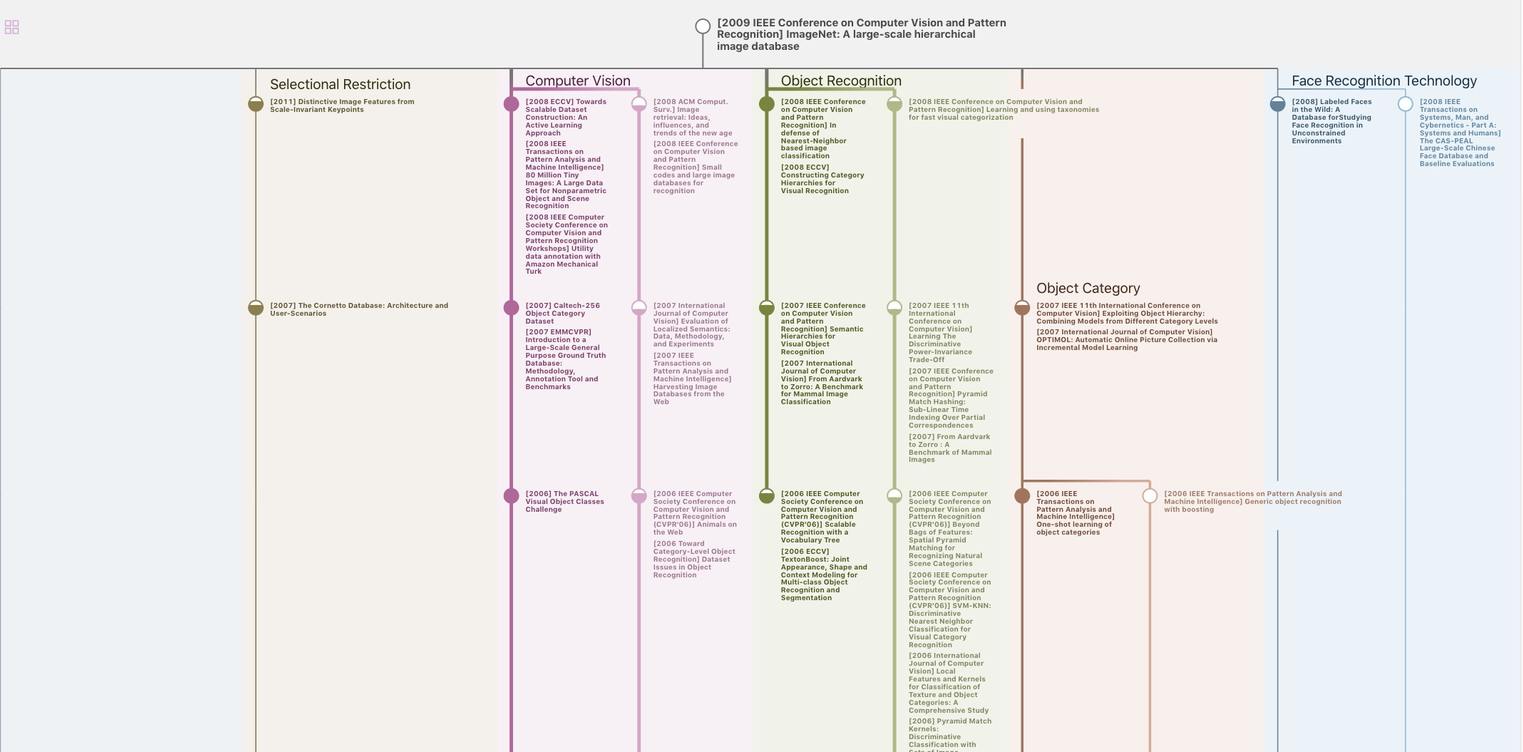
生成溯源树,研究论文发展脉络
Chat Paper
正在生成论文摘要