Generalized DFTB repulsive potentials from unsupervised machine learning.
JOURNAL OF CHEMICAL THEORY AND COMPUTATION(2018)
Abstract
We combine the approximate density-functional tight-binding (DFTB) method with unsupervised machine learning. This allows us to improve transferability and accuracy, make use of large quantum chemical data sets for the parametrization, and efficiently automatize the parametrization process of DFTB. For this purpose, generalized pair-potentials are introduced, where the chemical environment is included during the learning process, leading to more specific effective two-body potentials. We train on energies and forces of equilibrium and nonequilibrium structures of 2100 molecules, and test on similar to 130 000 organic molecules containing O, N, C, H, and F atoms. Atomization energies of the reference method can be reproduced within an error of similar to 2.6 kcal/mol, indicating drastic improvement over standard DFTB.
MoreTranslated text
Key words
machine learning,density-functional,tight-binding
AI Read Science
Must-Reading Tree
Example
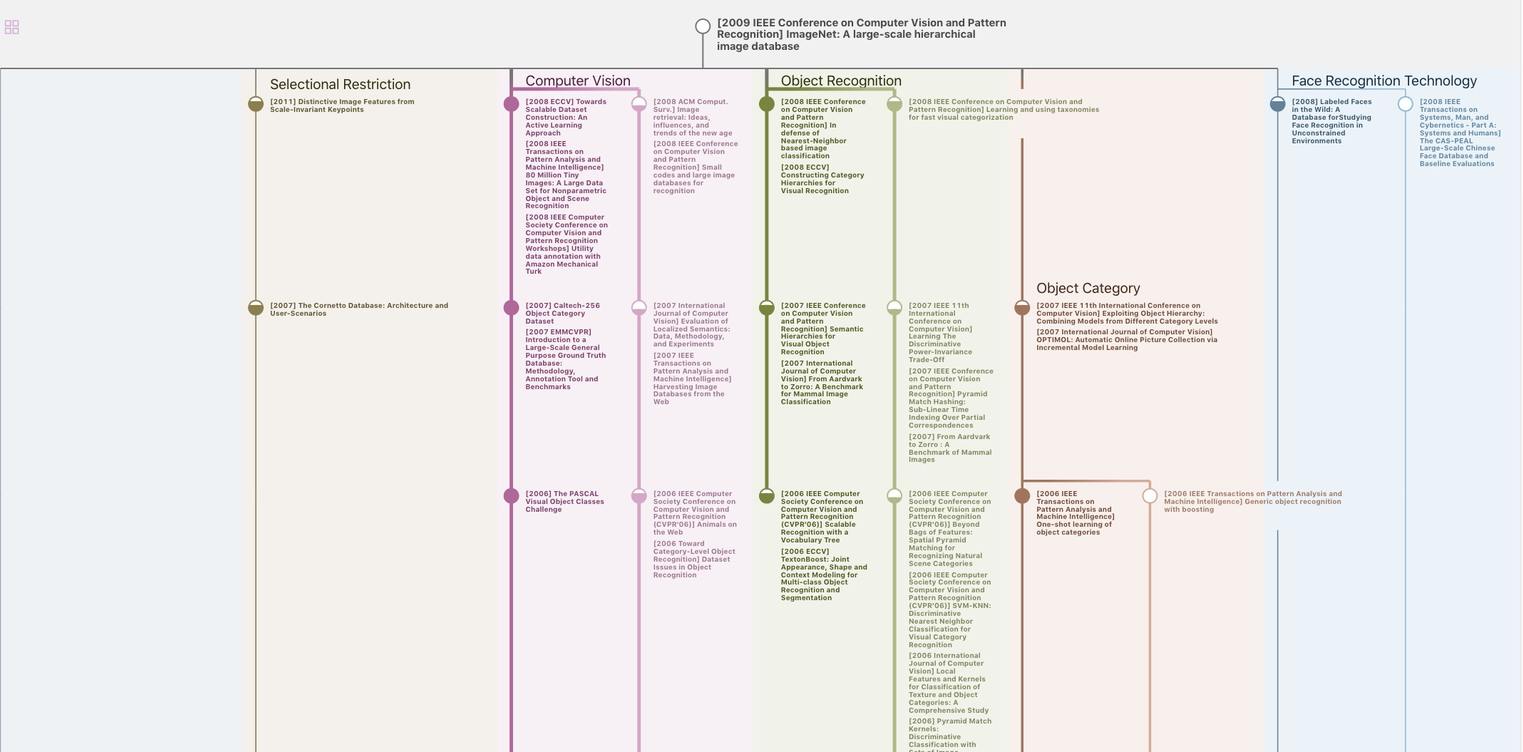
Generate MRT to find the research sequence of this paper
Chat Paper
Summary is being generated by the instructions you defined