Nanostructured Germanium Synthesized by High-Pressure Chemical Vapor Deposition in Mesoporous Silica Templates
JOURNAL OF MATERIALS SCIENCE-MATERIALS IN ELECTRONICS(2023)
The Pennsylvania State University
Abstract
Nanostructured semiconductors are interesting because of their varied electronic and optical properties compared to the bulk. Using ordered porous materials as templates is an appealing approach to prepare nanostructured materials. However, the very small pore sizes (< 10 nm) of many mesoporous silicas make traditional deposition methods for germanium difficult, resulting in aggregated particles or voids in the deposited material. To overcome this challenge, high-pressure chemical vapor deposition (HPCVD) has been used to deposit germanium within the pore network of KIT-5 mesoporous silica. This technique allows for smooth, continuous deposition within small, tortuous pore networks. Both crystalline and amorphous materials can be produced, expanding the applicability of the resulting materials for various uses. The resulting nanocrystalline germanium has 5-nm features derived from the parent KIT-5 and is the smallest templated material prepared using HPCVD to date. This work represents the first time a three-dimensional mesoporous silica, with features ≤ 5 nm, has been uniformly filled with a semiconductor.
MoreTranslated text
Key words
Nanocomposite Materials,Ordered Nanoporous Arrays,Pore Structure Characterization,Porous Silicon,Mesoporous Materials
求助PDF
上传PDF
View via Publisher
AI Read Science
AI Summary
AI Summary is the key point extracted automatically understanding the full text of the paper, including the background, methods, results, conclusions, icons and other key content, so that you can get the outline of the paper at a glance.
Example
Background
Key content
Introduction
Methods
Results
Related work
Fund
Key content
- Pretraining has recently greatly promoted the development of natural language processing (NLP)
- We show that M6 outperforms the baselines in multimodal downstream tasks, and the large M6 with 10 parameters can reach a better performance
- We propose a method called M6 that is able to process information of multiple modalities and perform both single-modal and cross-modal understanding and generation
- The model is scaled to large model with 10 billion parameters with sophisticated deployment, and the 10 -parameter M6-large is the largest pretrained model in Chinese
- Experimental results show that our proposed M6 outperforms the baseline in a number of downstream tasks concerning both single modality and multiple modalities We will continue the pretraining of extremely large models by increasing data to explore the limit of its performance
Upload PDF to Generate Summary
Must-Reading Tree
Example
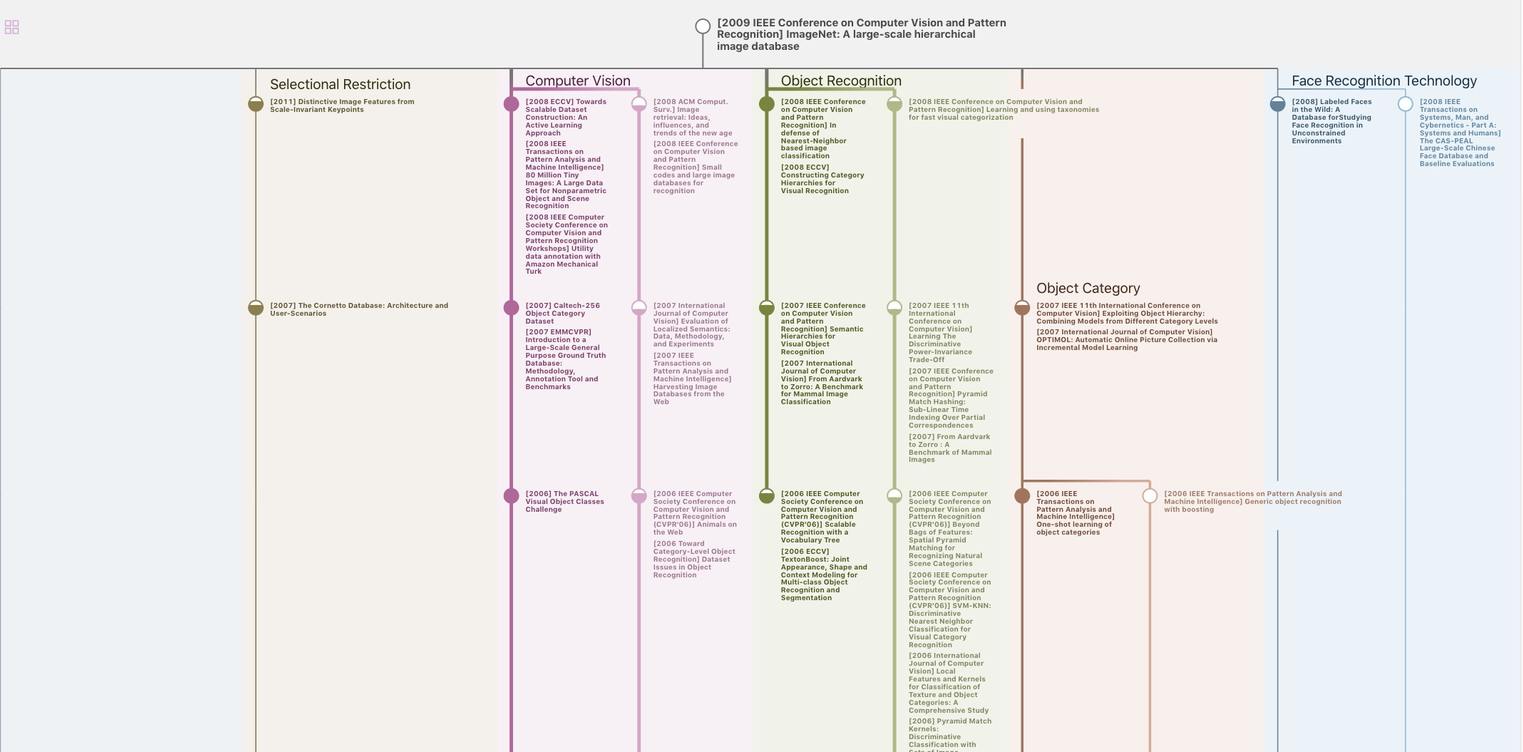
Generate MRT to find the research sequence of this paper
Related Papers
2006
被引用261 | 浏览
2003
被引用63 | 浏览
2009
被引用28 | 浏览
2010
被引用26 | 浏览
2012
被引用107 | 浏览
1983
被引用1385 | 浏览
2020
被引用11 | 浏览
2020
被引用7 | 浏览
Data Disclaimer
The page data are from open Internet sources, cooperative publishers and automatic analysis results through AI technology. We do not make any commitments and guarantees for the validity, accuracy, correctness, reliability, completeness and timeliness of the page data. If you have any questions, please contact us by email: report@aminer.cn
Chat Paper