Rat Cortical Layers Classification Extracting Evoked Local Field Potential Images with Implanted Multi-Electrode Sensor.
2018 IEEE 20th International Conference on e-Health Networking, Applications and Services (Healthcom)(2018)
摘要
One of the most ambitious goals of neuroscience and its neuroprosthetic applications is to interface intelligent electronic devices with the biological brain to cure neurological diseases. This emerging research field builds on our growing understanding of brain circuits and on recent technological advances in miniaturization of implantable multi-electrode-arrays (MEAs) to record brain signals at high spatiotemporal resolution. Data processing is needed to extract useful information from the recorded neural activity to better understand the function of underlying neural circuits and, in perspective, to operate neuroprosthetic devices. In this context, machine learning approaches are increasingly used in many application scenarios. This paper focuses on processing data of evoked local field potentials (LFPs) recorded from the rat barrel cortex using a miniaturized 16×16 MEA. We evaluated machine learning algorithms and trained an optimized classifier to detect at which cortical depth the neural activity is measured. We demonstrate with experimental results that machine learning can be applied successfully to noisy single-trial LFPs offering up to 99.11% of test accuracy in classifying signals acquired from different cortical layers. As such, the method is a very promising starting point toward real-time decoding of cerebral activities with low power consumption digital processors for brain-machine interfacing and neuroprosthetic applications.
更多查看译文
关键词
neuroscience,machine learning,brain-chip interface,image processing,bio-sensors,implantable sensors
AI 理解论文
溯源树
样例
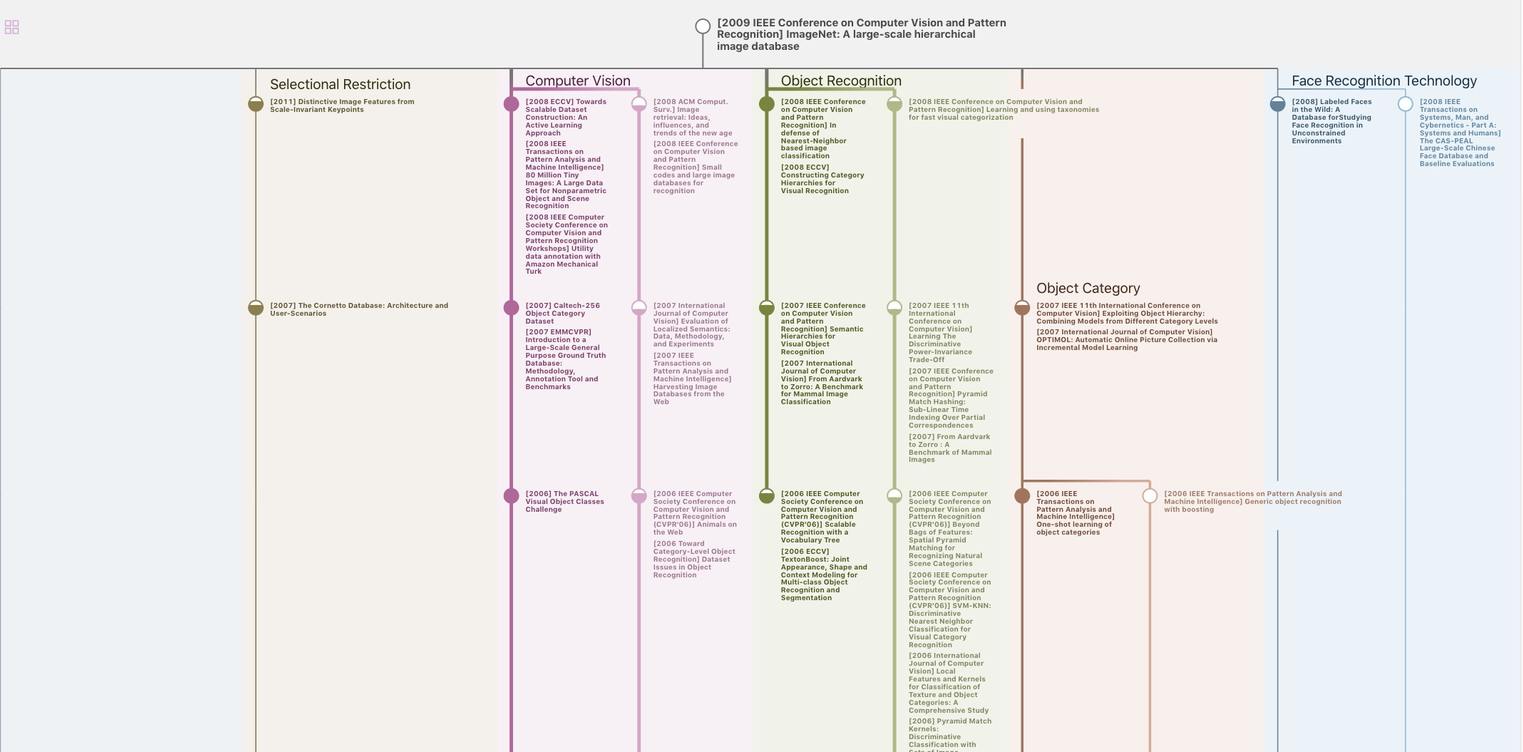
生成溯源树,研究论文发展脉络
Chat Paper
正在生成论文摘要