Regularizing Feature Distribution Using Sliced Wasserstein Distance for Semi-supervised Learning.
MIWAI(2018)
摘要
We propose a novel consistency based regularization method for semi-supervised image classification, called feature distribution matching (FDM), which is designed to induce smoothness of feature space by reducing sliced Wasserstein distance between feature distributions of labeled and unlabeled set. Unlike previous perturbation based methods, FDM does not require extra computational cost except one regularization loss. Our result shows that FDM combined with entropy minimization improves classification accuracy compared to supervised-only baseline and some previous methods. We also analyze our method by visualizing feature embeddings which shows that FDM lead smooth data manifold on feature space.
更多查看译文
关键词
Semi-supervised learning, Deep neural network, Image classification
AI 理解论文
溯源树
样例
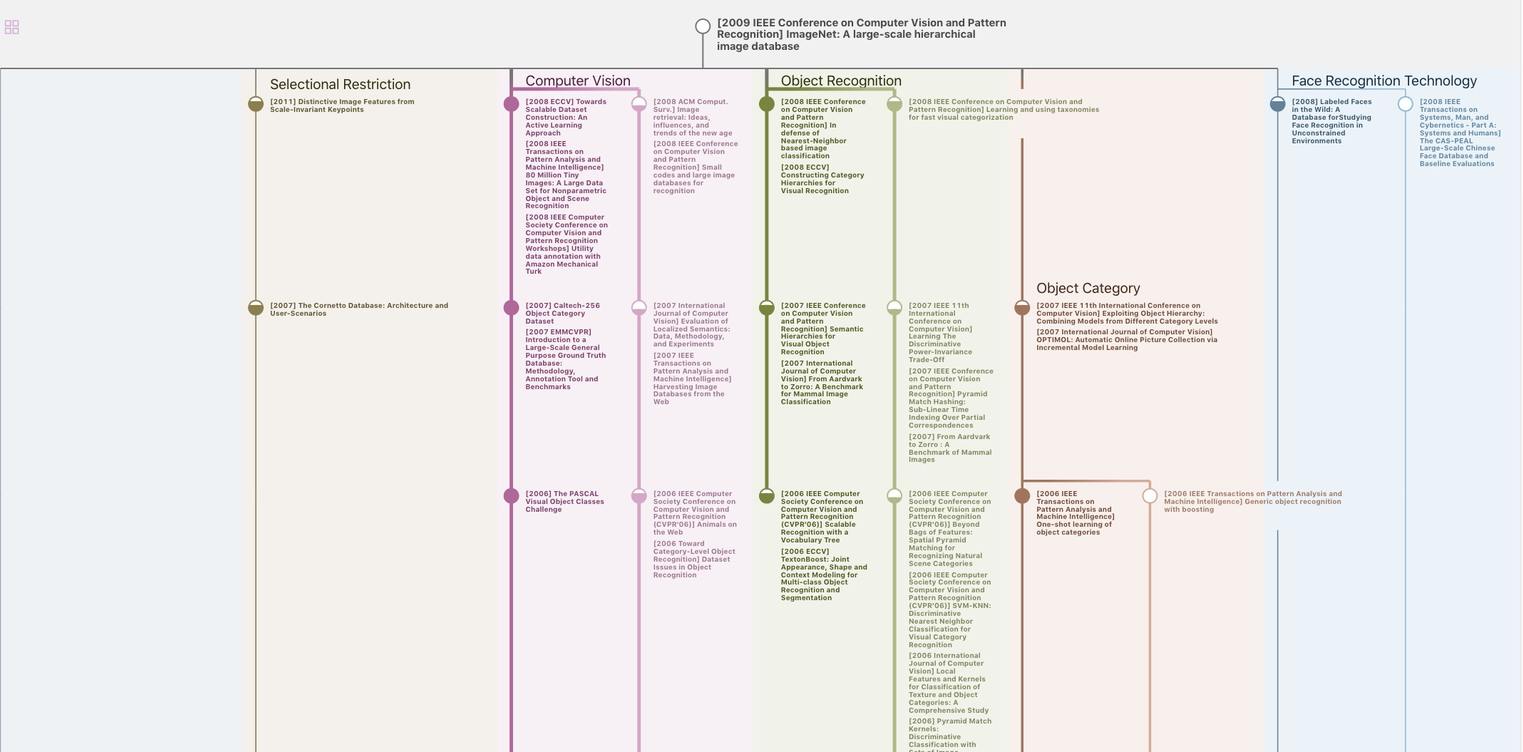
生成溯源树,研究论文发展脉络
Chat Paper
正在生成论文摘要