A Deep Learning Approach for the Classification of Neuronal Cell Types.
Annual International Conference of the IEEE Engineering in Medicine and Biology Society(2018)
摘要
Classification of neurons from extracellular recordings is mainly limited to putatively excitatory or inhibitory units based on the spike shape and firing patterns. Narrow waveforms are considered to be fast spiking inhibitory neurons and broad waveforms excitatory neurons. The aim of this work is twofold. First, we intend to use the rich spatial information from high-density Multi-Electrode Arrays (MEAs) to make classification more robust; second, we hope to be able to classify sub-types of excitatory and inhibitory neurons. We first built, in simulation, a large dataset of action potentials from detailed neural models. Then, we extracted spike features from the simulated recordings on a high-density Multi-Electrode Array model. Finally, we used a Convolutional Neural Networks (CNN), to classify the different cell types. Compared with the ground truth data from the simulated dataset, the results show that this forward modelling/machine learning approach is very robust in recognizing excitatory and inhibitory spikes (accuracy $\ge 92.15$%). Additionally, the approach can, to a certain extent, correctly classify different cell sub-types. As the detail and fidelity of neural models increase and high-density recordings become available, this approach could become a viable and robust alternative for classification of neural cell types from in-vivo extracellular recordings.
更多查看译文
关键词
Action Potentials,Deep Learning,Machine Learning,Models, Neurological,Neural Networks, Computer,Neurons
AI 理解论文
溯源树
样例
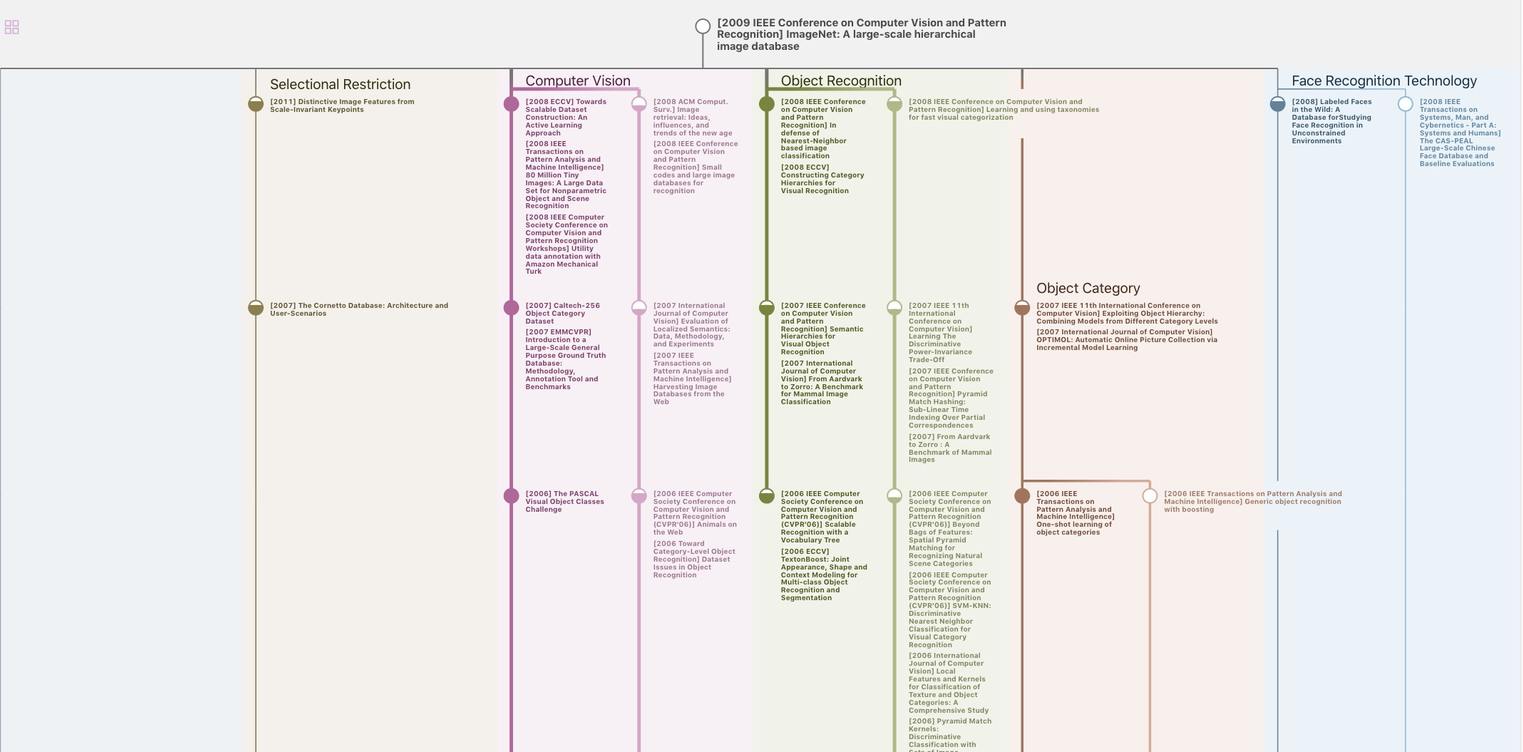
生成溯源树,研究论文发展脉络
Chat Paper
正在生成论文摘要