EFFICIENT SEPARABLE FILTER ESTIMATION USING RANK-1 CONVOLUTIONAL DICTIONARY LEARNING
2018 IEEE 28TH INTERNATIONAL WORKSHOP ON MACHINE LEARNING FOR SIGNAL PROCESSING (MLSP)(2018)
摘要
Natively learned separable filters for Convolutional Sparse Coding (CSC) have recently been shown to provide equivalent reconstruction performance to their non-separable counterparts (as opposed to approximated separable filters), while reducing computational cost. Furthermore, multiple approaches to optimize the Dictionary Update stage of Convolutional Dictionary Learning (CDL) methods based on the Accelerated Proximal Gradient (APG) framework have recently been proposed. In this paper, we propose a novel separable filter learning method based on the rank-1 decomposition, and test its performance against the existing separable approaches. In adittion, we evaluate how APG-based variations couple with our proposed method in order to improve computational runtime. Our results show that the filters learned through our proposed method match the performance of other natively-learned separable filters, while providing a significant runtime improvement in the learning process through our APG-based implementation.
更多查看译文
关键词
Separable Filter learning,Convolutional Sparse Representation,Convolutional Sparse Coding
AI 理解论文
溯源树
样例
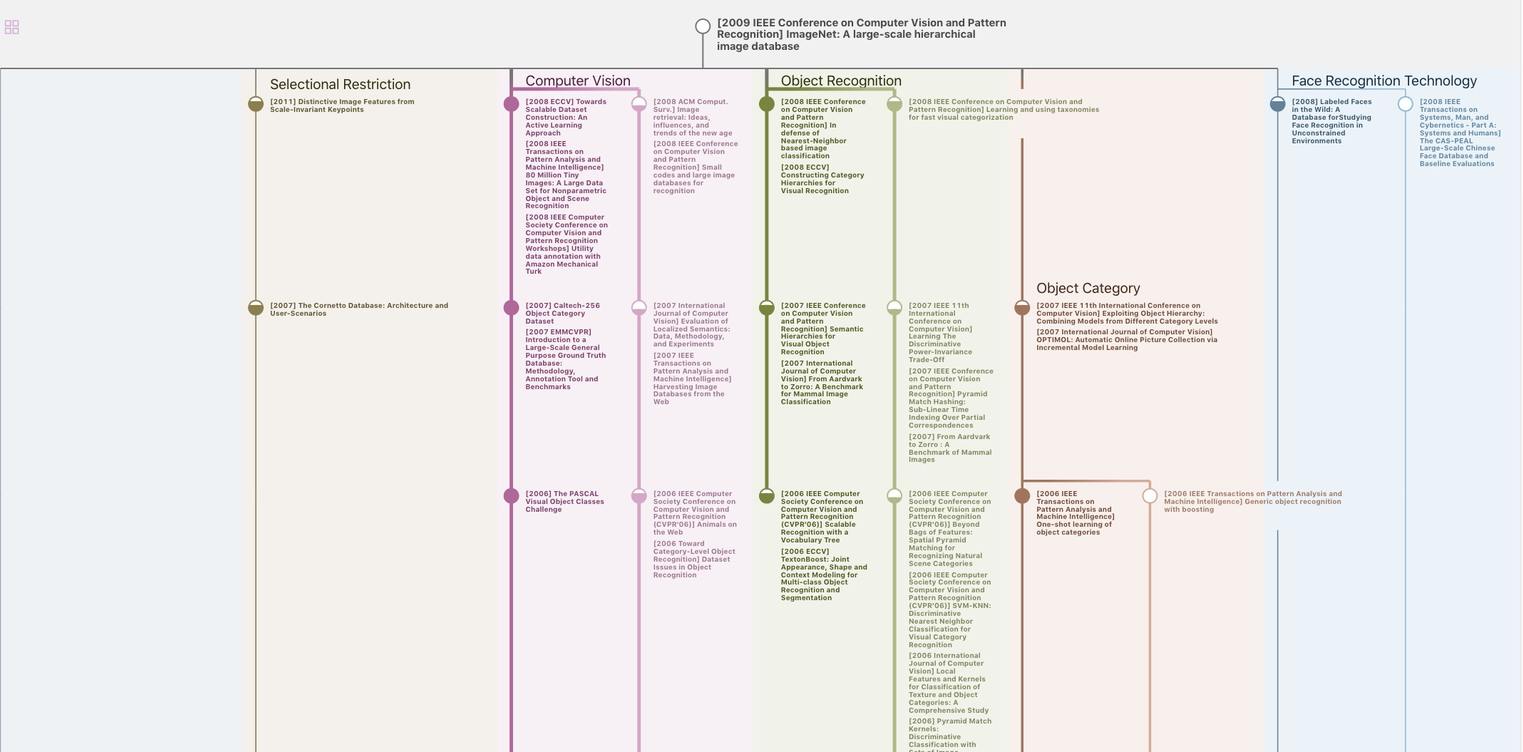
生成溯源树,研究论文发展脉络
Chat Paper
正在生成论文摘要