Scale-Transferrable Object Detection.
2018 IEEE/CVF Conference on Computer Vision and Pattern Recognition(2018)
摘要
Scale problem lies in the heart of object detection. In this work, we develop a novel Scale-Transferrable Detection Network (STDN) for detecting multi-scale objects in images. In contrast to previous methods that simply combine object predictions from multiple feature maps from different network depths, the proposed network is equipped with embedded super-resolution layers (named as scale-transfer layer/module in this work) to explicitly explore the inter scale consistency nature across multiple detection scales. Scale-transfer module naturally fits the base network with little computational cost. This module is further integrated with a dense convolutional network (DenseNet) to yield a one-stage object detector. We evaluate our proposed architecture on PASCAL VOC 2007 and MS COCO benchmark tasks and STDN obtains significant improvements over the comparable state-of-the-art detection models.
更多查看译文
关键词
STDN,multiscale objects,object predictions,multiple feature maps,super-resolution layers,multiple detection scales,dense convolutional network,network depths,scale-transferrable detection network,scale-transferrable object detection,DenseNet
AI 理解论文
溯源树
样例
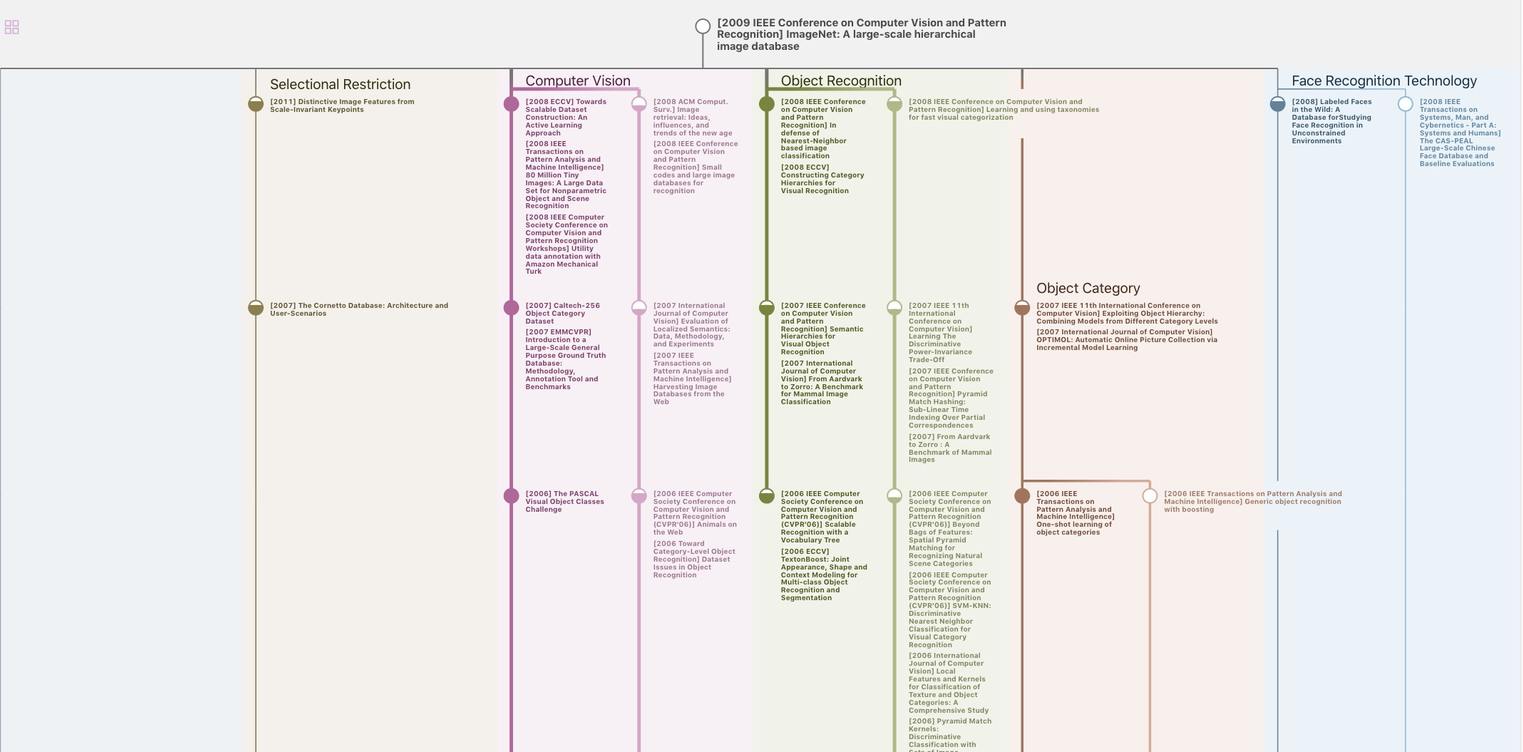
生成溯源树,研究论文发展脉络
Chat Paper
正在生成论文摘要