PartsNet: A Unified Deep Network for Automotive Engine Precision Parts Defect Detection
CSAI '18: Proceedings of the 2018 2nd International Conference on Computer Science and Artificial Intelligence(2018)
摘要
Defect detection is a basic and essential task in automatic parts production, especially for automotive engine precision parts. In this paper, we propose a new idea to construct a deep convolutional network combining related knowledge of feature processing and the representation ability of deep learning. Our algorithm consists of a pixel-wise segmentation Deep Neural Network (DNN) and a feature refining network. The fully convolutional DNN is presented to learn basic features of parts defects. After that, several typical traditional methods which are used to refine the segmentation results are transformed into convolutional manners and integrated. We assemble these methods as a shallow network with fixed weights and empirical thresholds. These thresholds are then released to enhance its adaptation ability and realize end-to-end training. Testing results on different datasets show that the proposed method has good portability and outperforms the state-of-the-art algorithms.
更多查看译文
关键词
Defect detection,PartsNet,fully convolutional DNN,result refinement
AI 理解论文
溯源树
样例
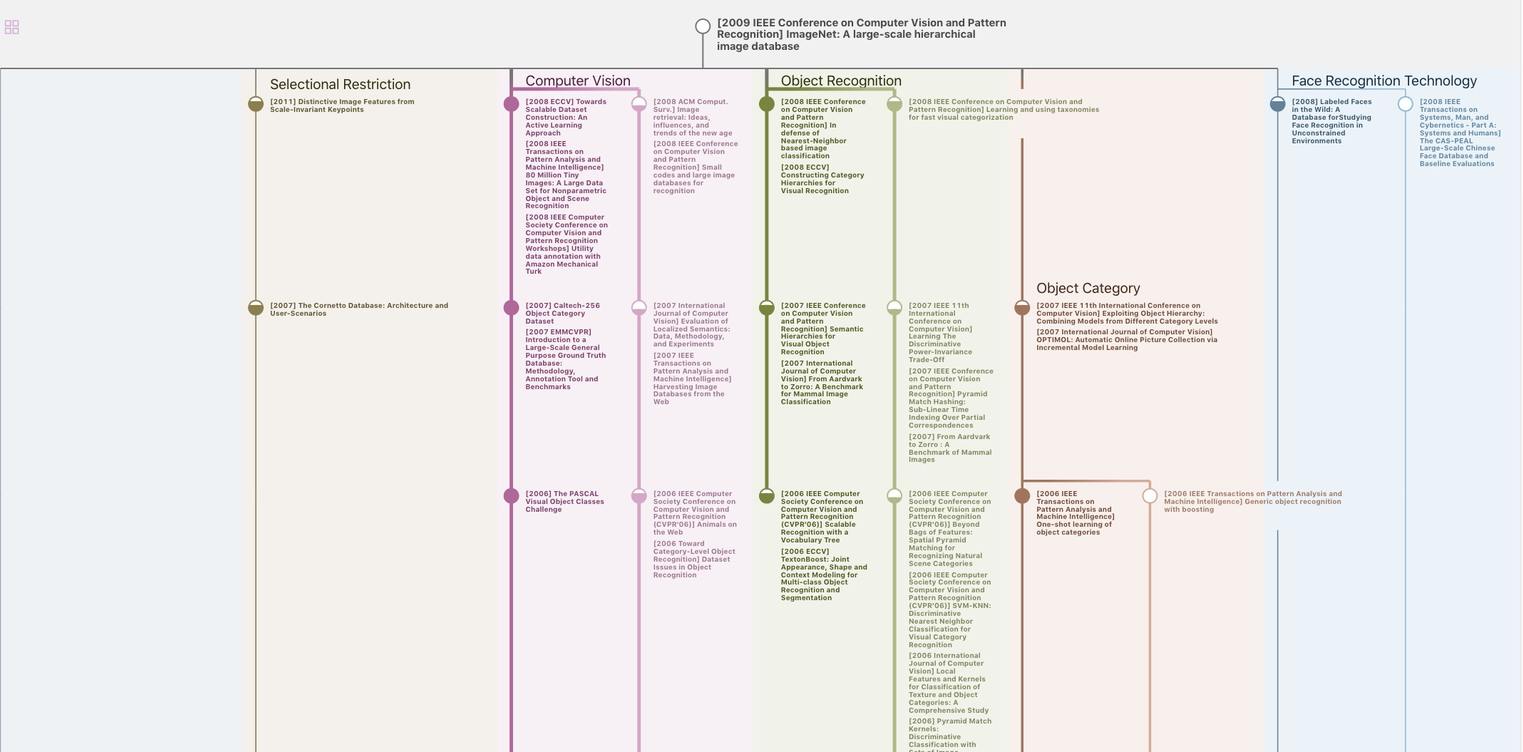
生成溯源树,研究论文发展脉络
Chat Paper
正在生成论文摘要