Dynamic Frame Skipping For Fast Speech Recognition In Recurrent Neural Network Based Acoustic Models
2018 IEEE INTERNATIONAL CONFERENCE ON ACOUSTICS, SPEECH AND SIGNAL PROCESSING (ICASSP)(2018)
摘要
A recurrent neural network is a powerful tool for modeling sequential data such as text and speech. While recurrent neural networks have achieved record-breaking results in speech recognition, one remaining challenge is their slow processing speed. The main cause comes from the nature of recurrent neural networks that read only one frame at each time step. Therefore, reducing the number of reads is an effective approach to reducing processing time. In this paper, we propose a novel recurrent neural network architecture called Skip-RNN, which dynamically skips speech frames that are less important. The Skip-RNN consists of an acoustic model network and skip-policy network that are jointly trained to classify speech frames and determine how many frames to skip. We evaluate our proposed approach on the Wall Street Journal corpus and show that it can accelerate acoustic model computation by up to 2.4 times without any noticeable degradation in transcription accuracy.
更多查看译文
关键词
recurrent neural networks, neural acoustic models, dynamic frame skipping, policy gradient methods
AI 理解论文
溯源树
样例
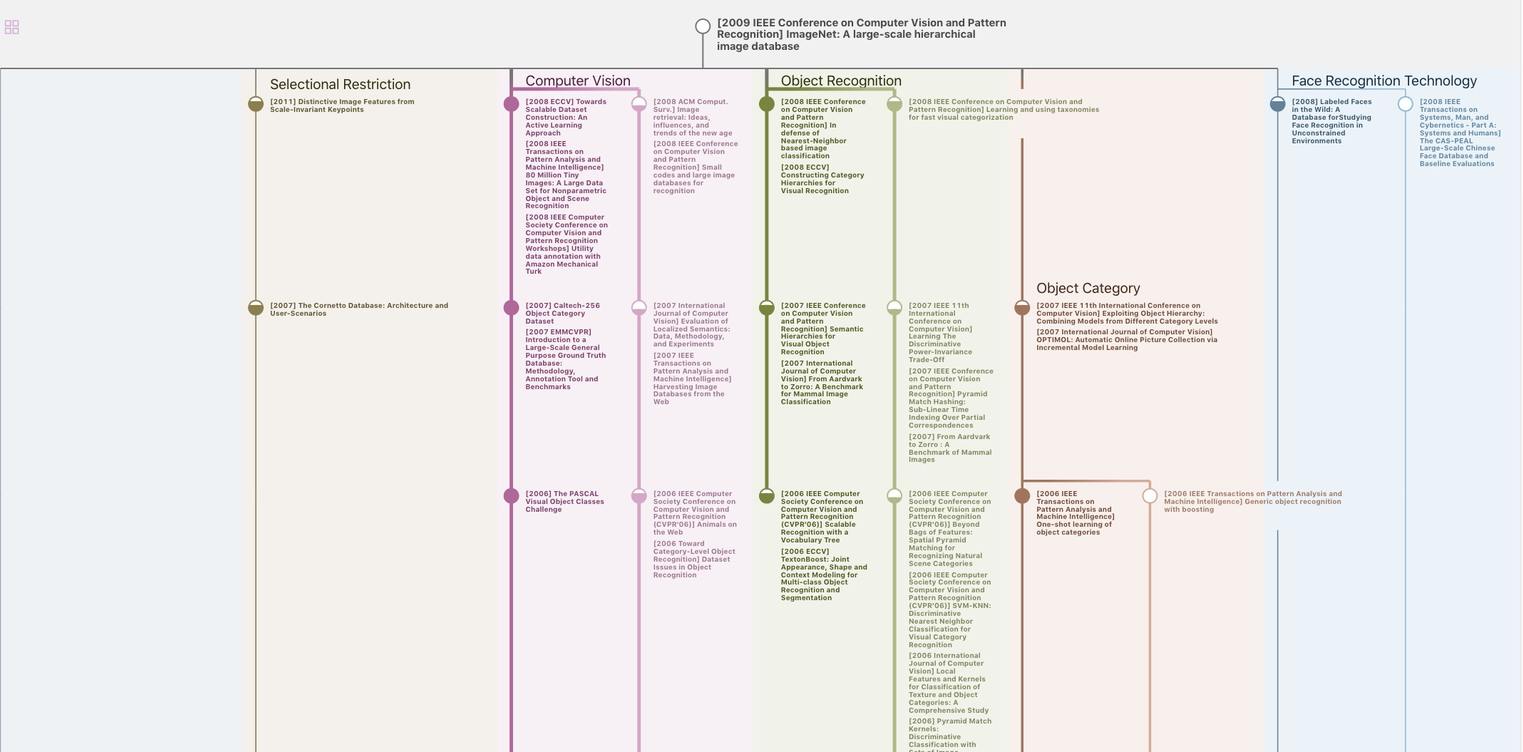
生成溯源树,研究论文发展脉络
Chat Paper
正在生成论文摘要