Adversarial Domain Adaptation for Variational Neural Language Generation in Dialogue Systems.
International Conference on Computational Linguistics (COLING)(2018)CCF B
Japan Advanced Institute of Science and Technology
Abstract
Domain Adaptation arises when we aim at learning from source domain a model that can per- form acceptably well on a different target domain. It is especially crucial for Natural Language Generation (NLG) in Spoken Dialogue Systems when there are sufficient annotated data in the source domain, but there is a limited labeled data in the target domain. How to effectively utilize as much of existing abilities from source domains is a crucial issue in domain adaptation. In this paper, we propose an adversarial training procedure to train a Variational encoder-decoder based language generator via multiple adaptation steps. In this procedure, a model is first trained on a source domain data and then fine-tuned on a small set of target domain utterances under the guidance of two proposed critics. Experimental results show that the proposed method can effec- tively leverage the existing knowledge in the source domain to adapt to another related domain by using only a small amount of in-domain data.
MoreTranslated text
Key words
Natural Language Generation,Spoken Dialogue Systems,Dialog Management,Language Understanding,Reinforcement Learning
PDF
View via Publisher
AI Read Science
AI Summary
AI Summary is the key point extracted automatically understanding the full text of the paper, including the background, methods, results, conclusions, icons and other key content, so that you can get the outline of the paper at a glance.
Example
Background
Key content
Introduction
Methods
Results
Related work
Fund
Key content
- Pretraining has recently greatly promoted the development of natural language processing (NLP)
- We show that M6 outperforms the baselines in multimodal downstream tasks, and the large M6 with 10 parameters can reach a better performance
- We propose a method called M6 that is able to process information of multiple modalities and perform both single-modal and cross-modal understanding and generation
- The model is scaled to large model with 10 billion parameters with sophisticated deployment, and the 10 -parameter M6-large is the largest pretrained model in Chinese
- Experimental results show that our proposed M6 outperforms the baseline in a number of downstream tasks concerning both single modality and multiple modalities We will continue the pretraining of extremely large models by increasing data to explore the limit of its performance
Try using models to generate summary,it takes about 60s
Must-Reading Tree
Example
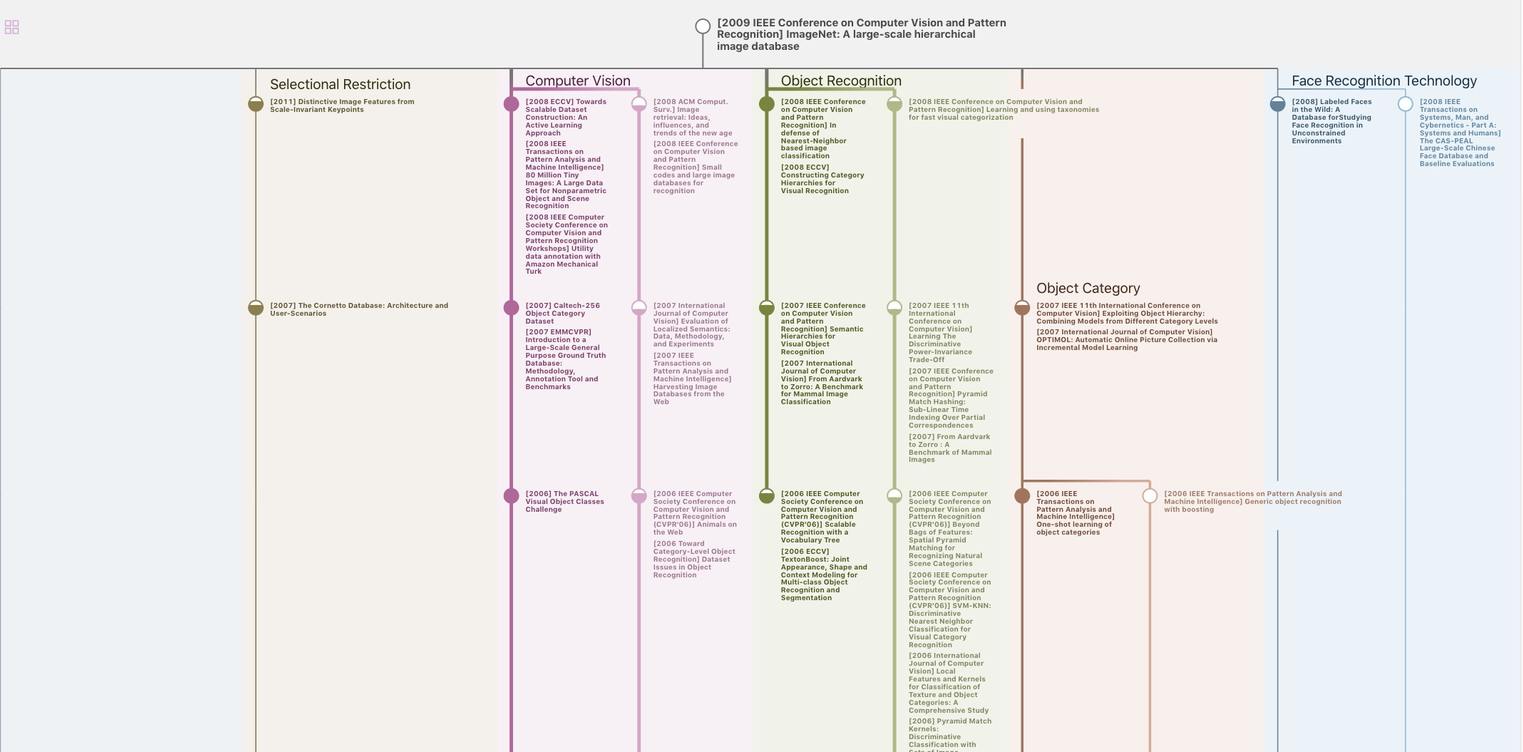
Generate MRT to find the research sequence of this paper
Related Papers
Meta-Learning for Low-resource Natural Language Generation in Task-oriented Dialogue Systems.
IJCAI 2019 2019
被引用114
Domain Adaptive Dialog Generation Via Meta Learning
Annual Meeting of the Association for Computational Linguistics 2019
被引用147
Proceedings of the 20th Annual SIGdial Meeting on Discourse and Dialogue 2019
被引用6
Schema-Guided Natural Language Generation.
International Conference on Natural Language Generation 2020
被引用12
Continual Learning for Natural Language Generation in Task-oriented Dialog Systems
FINDINGS OF THE ASSOCIATION FOR COMPUTATIONAL LINGUISTICS, EMNLP 2020 2020
被引用76
A Survey of Natural Language Generation in Task-Oriented Dialogue System
Journal of Chinese Information Processing 2022
被引用0
The method of hybrid code networks based on time-aware attention mechanism
Journal of Shandong University(Engineering Science) 2022
被引用0
A Method of Extracting Pores from Rock Slices Based on U-Net
Natural Science Journal of Hainan University 2022
被引用1
Towards Human-Like Machine Comprehension: Few-Shot Relational Learning in Visually-Rich Documents
arXiv (Cornell University) 2024
被引用0
Data Disclaimer
The page data are from open Internet sources, cooperative publishers and automatic analysis results through AI technology. We do not make any commitments and guarantees for the validity, accuracy, correctness, reliability, completeness and timeliness of the page data. If you have any questions, please contact us by email: report@aminer.cn
Chat Paper
去 AI 文献库 对话