Classification-Based Prediction of Effective Connectivity Between Timeseries with a Realistic Cortical Network Model
Frontiers in computational neuroscience(2018)
摘要
Effective connectivity measures the pattern of causal interactions between brain regions. Traditionally, these patterns of causality are inferred from brain recordings using either non-parametric, i.e., model-free, or parametric, i.e., model-based, approaches. The latter approaches, when based on biophysically plausible models, have the advantage that they may facilitate the interpretation of causality in terms of underlying neural mechanisms. Recent biophysically plausible neural network models of recurrent microcircuits have shown the ability to reproduce well the characteristics of real neural activity and can be applied to model interacting cortical circuits. Unfortunately, however, it is challenging to invert these models in order to estimate effective connectivity from observed data. Here, we propose to use a classification-based method to approximate the result of such complex model inversion. The classifier predicts the pattern of causal interactions given a multivariate timeseries as input. The classifier is trained on a large number of pairs of multivariate timeseries and the respective pattern of causal interactions, which are generated by simulation from the neural network model. In simulated experiments, we show that the proposed method is much more accurate in detecting the causal structure of timeseries than current best practice methods. Additionally, we present further results to characterize the validity of the neural network model and the ability of the classifier to adapt to the generative model of the data.
更多查看译文
关键词
causality,classification and prediction,cortical network model,neural networks,connectivity,effective connectivity,timeseries analysis
AI 理解论文
溯源树
样例
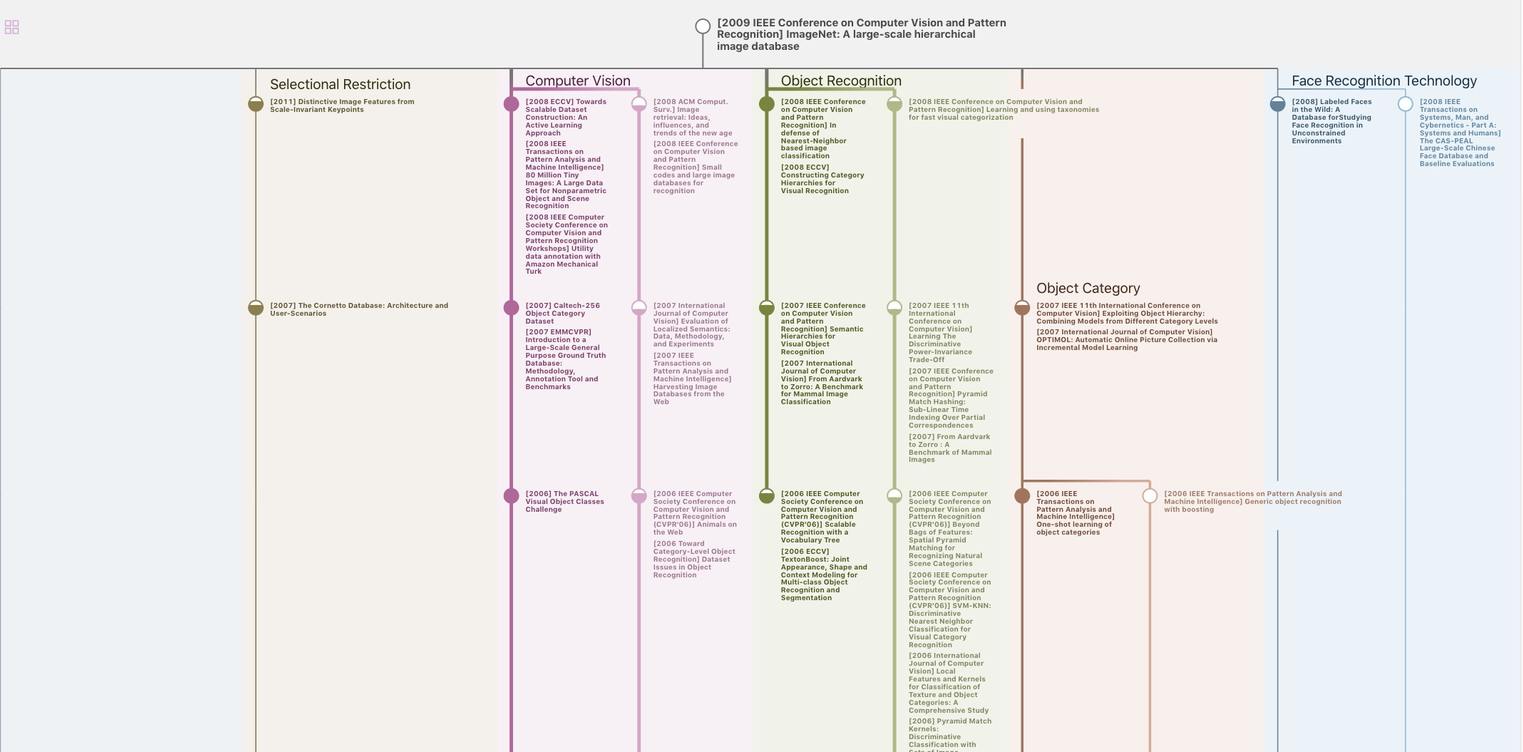
生成溯源树,研究论文发展脉络
Chat Paper
正在生成论文摘要