Large-scale Distance Metric Learning with Uncertainty
2018 IEEE/CVF Conference on Computer Vision and Pattern Recognition(2018)
摘要
Distance metric learning (DML) has been studied extensively in the past decades for its superior performance with distance-based algorithms. Most of the existing methods propose to learn a distance metric with pairwise or triplet constraints. However, the number of constraints is quadratic or even cubic in the number of the original examples, which makes it challenging for DML to handle the large-scale data set. Besides, the real-world data may contain various uncertainty, especially for the image data. The uncertainty can mislead the learning procedure and cause the performance degradation. By investigating the image data, we find that the original data can be observed from a small set of clean latent examples with different distortions. In this work, we propose the margin preserving metric learning framework to learn the distance metric and latent examples simultaneously. By leveraging the ideal properties of latent examples, the training efficiency can be improved significantly while the learned metric also becomes robust to the uncertainty in the original data. Furthermore, we can show that the metric is learned from latent examples only, but it can preserve the large margin property even for the original data. The empirical study on the benchmark image data sets demonstrates the efficacy and efficiency of the proposed method.
更多查看译文
关键词
DML,distance-based algorithms,learning procedure,performance degradation,metric learning framework,benchmark image datasets,distance metric learning,triplet constraints,pairwise constraints
AI 理解论文
溯源树
样例
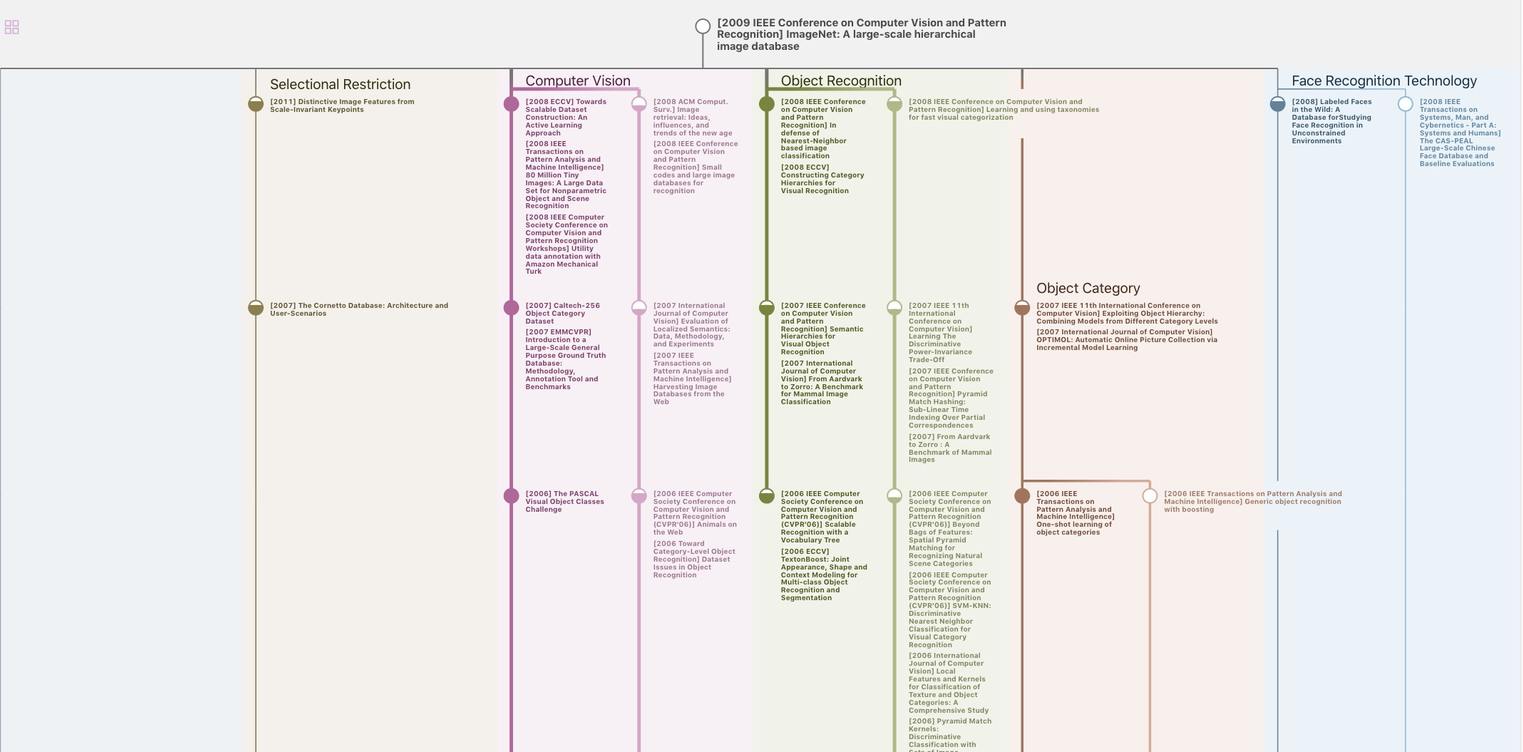
生成溯源树,研究论文发展脉络
Chat Paper
正在生成论文摘要