Improving Tuberculosis Diagnostics Using Deep Learning and Mobile Health Technologies among Resource-Poor and Marginalized Communities.
IEEE/ACM International Conference on Connected Health Applications, Systems and Engineering Technologies(2016)
摘要
Tuberculosis (TB) is a chronic infectious disease worldwide and remains a major cause of death globally. Of the estimated 9 million people who developed TB in 2013, over 80% were in South-East Asia, Western Pacific, and African. The majority of the infected populations was from resource-poor and marginalized communities with weak healthcare infrastructure. Reducing TB diagnosis delay is critical in mitigating disease transmission and minimizing the reproductive rate of the tuberculosis epidemic. The combination of machine learning and mobile computing techniques offers a unique opportunity to accelerate the TB diagnosis among these communities. The ultimate goal of our research is to reduce patient wait times for being diagnosed with this infectious disease by developing new machine learning and mobile health techniques to the TB diagnosis problem. In this paper, we first introduce major technique barriers and proposed system architecture. Then we report two major progresses we recently made. The first activity aims to develop large-scale, real-world and well-annotated X-ray image database dedicated for automated TB screening. The second research activity focus on developing effective and efficient computational models (in particularly, deep convolutional neural networks (CNN)-based models) to classify the image into different category of TB manifestations. Experimental results have demonstrated the effectiveness of our approach. Our future work includes: (1) to further improve the performance of the algorithms, and (2) to deploy our system in the city of Carabayllo in Perú, a densely occupied urban community and high-burden TB.
更多查看译文
关键词
tuberculosis,diagnosis,deep learning,deep convolutional neural networks,mHealth,mobile computing,Peru
AI 理解论文
溯源树
样例
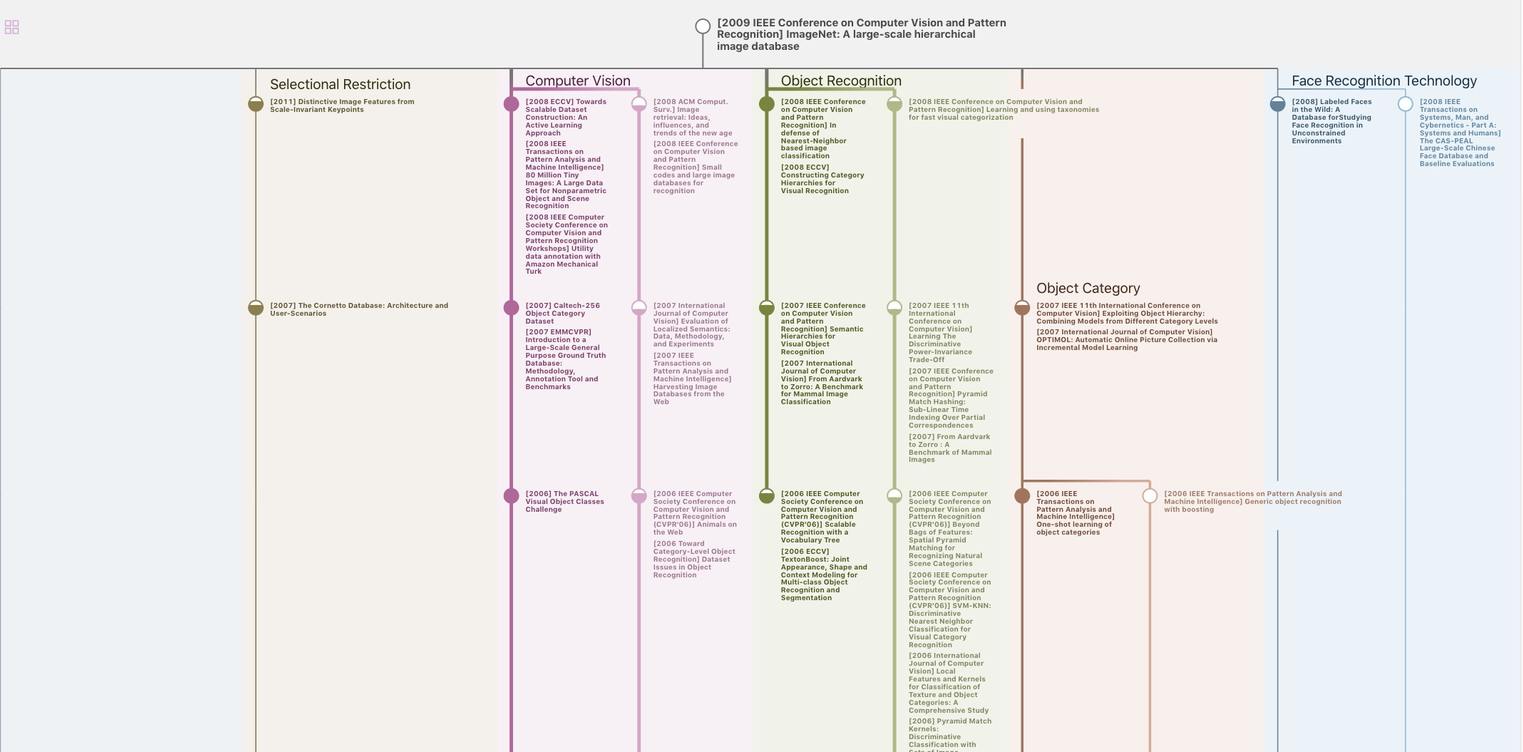
生成溯源树,研究论文发展脉络
Chat Paper
正在生成论文摘要