Joint Graph Optimization Towards Crowd Based Mapping
2017 IEEE 20th International Conference on Intelligent Transportation Systems (ITSC)(2017)
摘要
In any autonomous driving system, the map for localization plays a vital part that is often underestimated. The map describes the world around the vehicle outside of the sensor view and is a main input into the decision making process in highly complicated scenarios. Thus there are strict requirements towards the accuracy and timeliness of the map. We present a robust and reliable approach towards crowd based mapping using a GraphSLAM framework based on radar sensors. We show on a parking lot that even in dynamically changing environments, the localization results are very accurate and reliable even in unexplored terrain without any map data. This can be achieved by collaborative map updates from multiple vehicles. To show these claims experimentally, the Joint Graph Optimization is compared to the ground truth on an industrial parking space. Mapping performance is evaluated using a dense map from a total station as reference and localization results are compared with a deeply coupled DGPS/INS system.
更多查看译文
关键词
autonomous driving system,decision making process,robust approach,reliable approach,radar sensors,localization results,collaborative map updates,mapping performance,joint graph optimization,crowd-based mapping
AI 理解论文
溯源树
样例
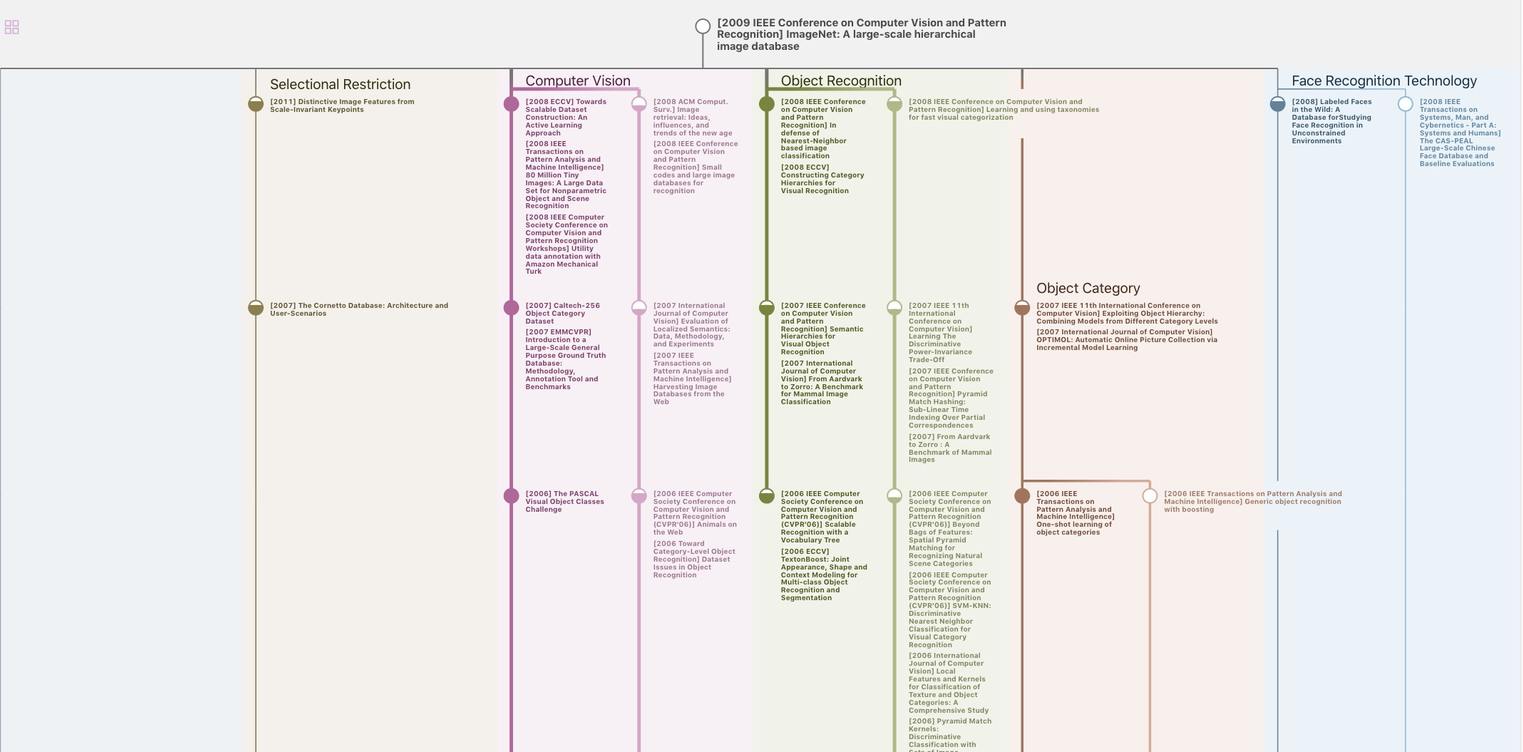
生成溯源树,研究论文发展脉络
Chat Paper
正在生成论文摘要