Cause-Effect Knowledge Acquisition and Neural Association Model for Solving A Set of Winograd Schema Problems
IJCAI 2017(2017)
摘要
This paper focuses on the investigations in Winograd Schema (WS), a challenging problem which has been proposed for measuring progress in commonsense reasoning.Due to the lack of commonsense knowledge and training data, very little work has been found on the WS problems in recent years.Actually, there is no shortcut to solve this problem except to collect more commonsense knowledge and design suitable models.Therefore, this paper addresses a set of WS problems by proposing a knowledge acquisition method and a general neural association model.To avoid the sparseness issue, the knowledge we aim to collect is the cause-effect relationships between thousands of commonly used words.The knowledge acquisition method supports us to extract hundreds of thousands of cause-effect pairs from large text corpus automatically.Meanwhile, a neural association model (NAM) is proposed to encode the association relationships between any two discrete events.Based on the extracted knowledge and the NAM models, in this paper, we successfully build a system for solving WS problems from scratch and achieve 70.0% accuracy.Most importantly, this paper provides a flexible framework to solve WS problems based on event association and neural network methods.
更多查看译文
关键词
Machine Learning: Machine Learning,Machine Learning: Deep Learning
AI 理解论文
溯源树
样例
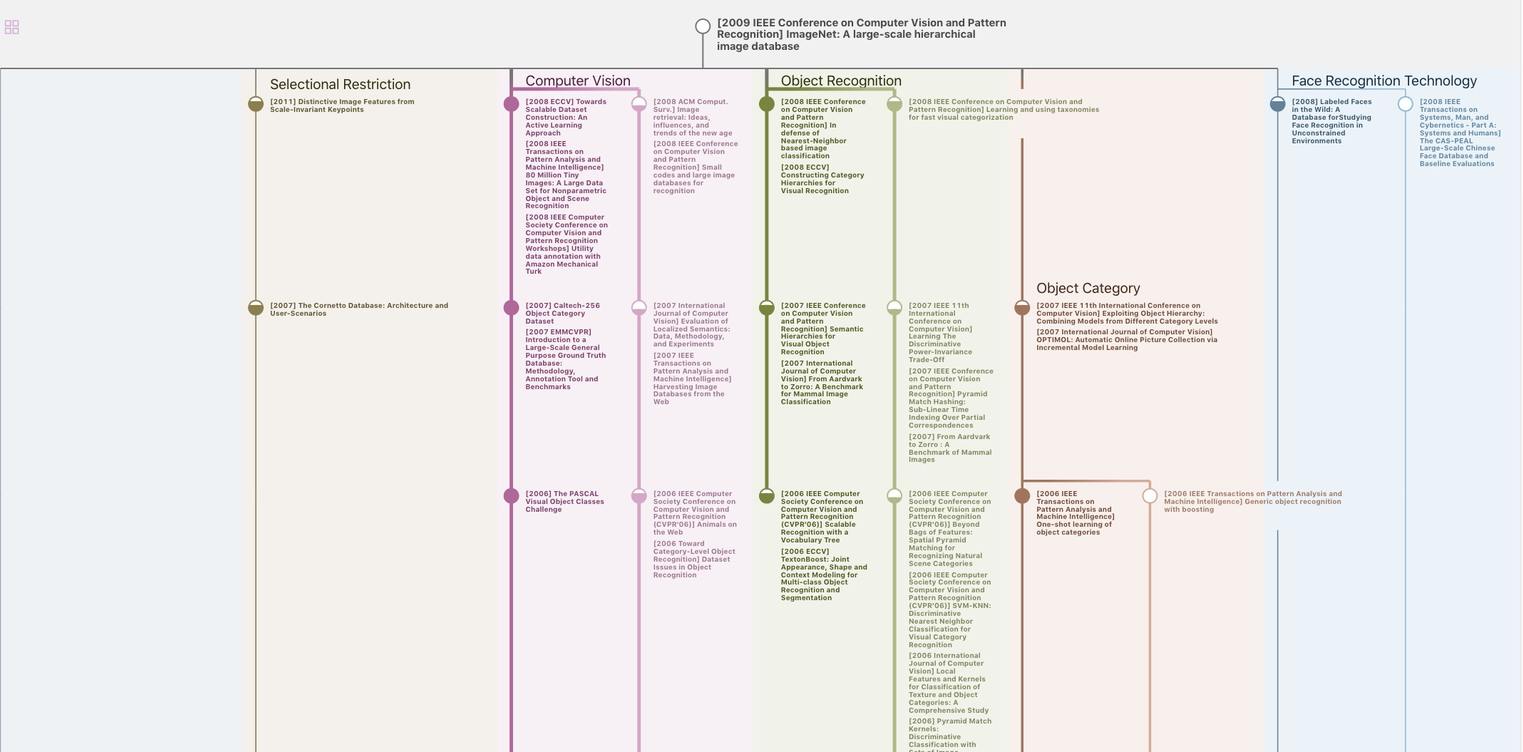
生成溯源树,研究论文发展脉络
Chat Paper
正在生成论文摘要