Thinning Deep Neural Networks for Sketch Recognition
IEEE Conference Proceedings(2016)
摘要
In this paper, we propose that deep neural networks that are thinner than typical image recognition networks can perform sketch recognition effectively. As in other computer vision problems, convolutional neural networks (CNNs) outperform other feature extraction methods significantly in sketch recognition. To date, two CNN structures have been proposed for sketch recognition, as described in [4] and [5], achieving classification accuracies of 74.9% and 75.42%, respectively. Although an easy way to improve performance is to design a deeper network, researchers currently tend to avoid an increase in the number of parameters. We attempted to find a more efficient network structure in terms of trade-off between memory usage and performance. Our experiments suggest that making convolutional layers thinner does not result in a significant drop in performance, which correspond to our expectation that low level features are simpler in sketches than those extracted from images.
更多查看译文
关键词
deep neural networks,sketch recognition,image recognition,computer vision,convolutional neural networks,feature extraction methods
AI 理解论文
溯源树
样例
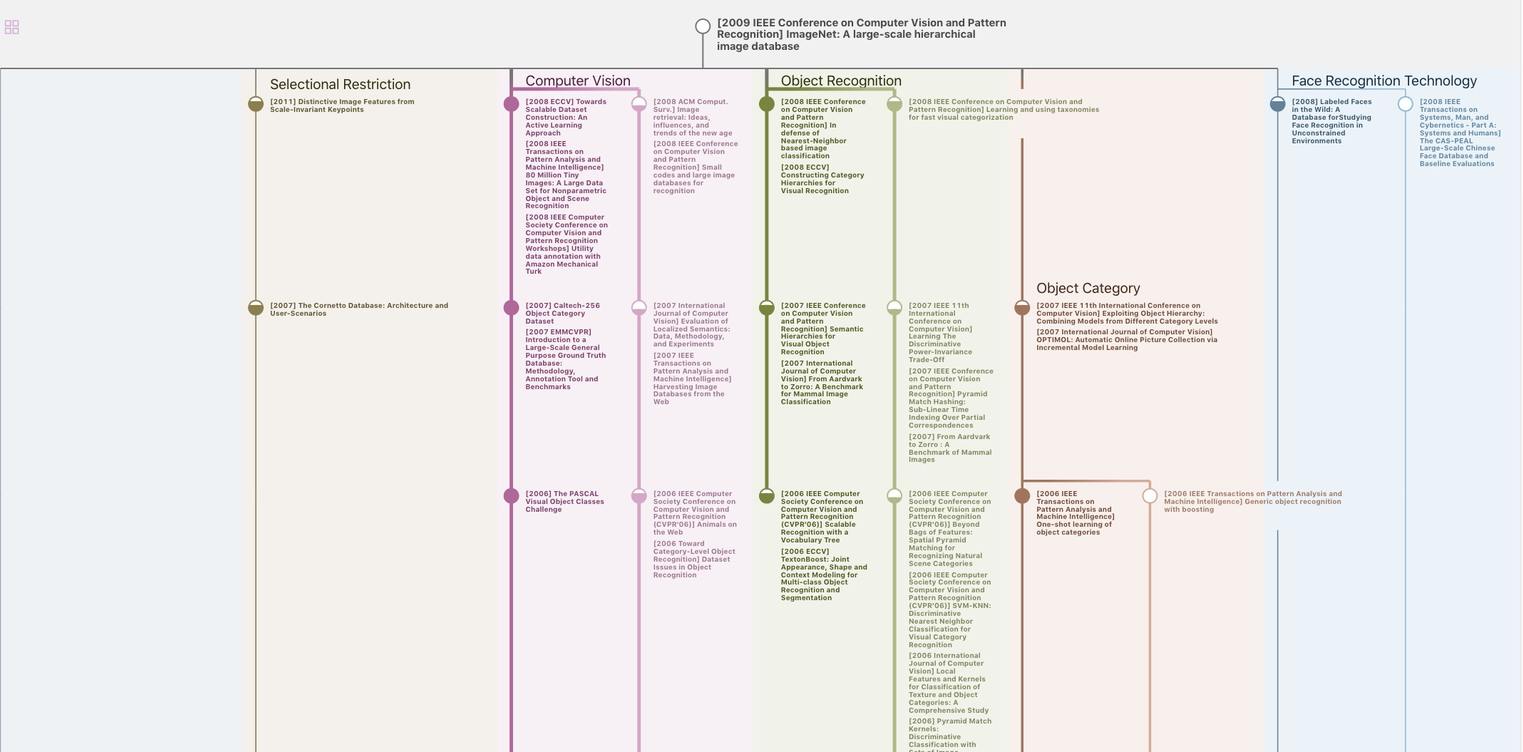
生成溯源树,研究论文发展脉络
Chat Paper
正在生成论文摘要