Sparse representation for colors of 3d point cloud via virtual adaptive sampling
2017 IEEE INTERNATIONAL CONFERENCE ON ACOUSTICS, SPEECH AND SIGNAL PROCESSING (ICASSP)(2017)
摘要
Sparse signal representation has proven to be an extremely powerful tool in a wide range of engineering applications. However, most of the existing techniques are designed for regular data (such as audio signals and images/videos) that uniformly lies in regular Euclidian spaces. This paper aims at extending sparse representation for irregular data (such as colors of 3D point clouds) that is defined on irregular domains embedded in Euclidean spaces. Dealing with the irregular structure of such data via a virtual adaptive sampling process, we formulate sparse representation as an eo-norm regularized optimization problem. Experimental results show that the proposed algorithm outperforms the state-of-the-art algorithm to a large extent: with the same number of nonzero coefficients, we improve the reconstruction quality up to 5 dB; conversely, fixing the reconstruction quality, our method uses only 55% coefficients. Using compressive sensing theory, we provide an intuitive explanation on how and why our algorithm works well in practice.
更多查看译文
关键词
3D point cloud,voxelization,sparse representation,compressive sensing,compression,reconstruction
AI 理解论文
溯源树
样例
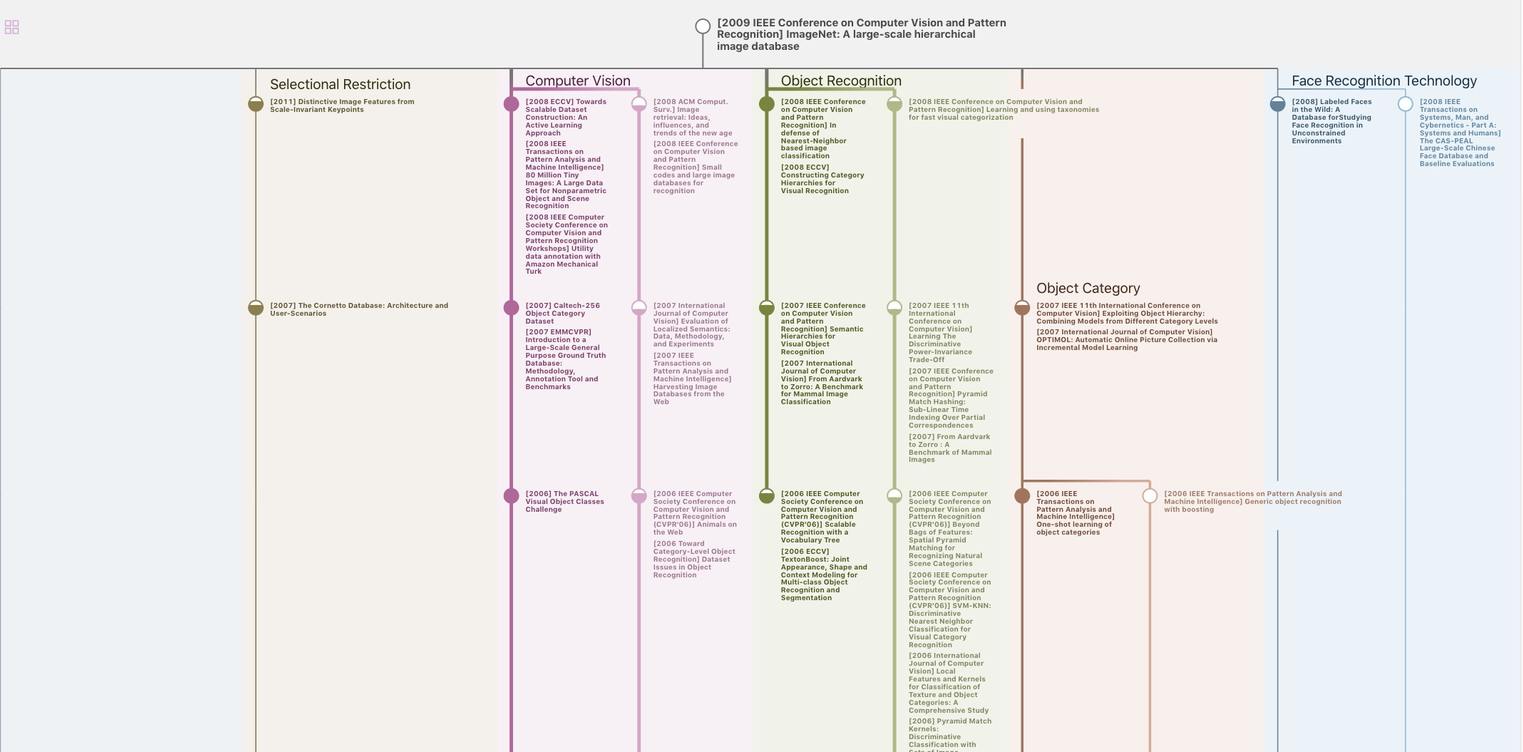
生成溯源树,研究论文发展脉络
Chat Paper
正在生成论文摘要