Diagnosis of Electric Vehicle Batteries Using Recurrent Neural Networks
IEEE transactions on industrial electronics(2017)
Abstract
In electric vehicle (EV) systems, diagnosing battery states, such as state of health (SOH), is crucial to guarantee safety. Such a diagnosis can be used to determine the replacement time for a battery or to assess the driving mileage. The majority of studies in recent decades have defined SOH under fairly constrained assumptions, such as cycling within a fixed range and in fixed temperature. Unfortunately, the assumptions cannot be applied to real-life EV batteries, which for the most part are cycled arbitrarily. In clear contrast, this paper studies how SOH can be estimated in more practical environments, in which batteries must support real-world driving patterns. This paper proposes a data-driven approach to trace SOH using measurable EV data, such as current (I) and voltage (V). While the existing data-driven approaches are mostly based on historical I/V distributions, our approach utilizes time-varying I/V instantiations, each portraying a snapshot during a short period. In particular, we design a model based on a recurrent neural network, which is highly suited to handling the sequential data. Our validation provides very robust and flexible results under various EV driving conditions, with the average error lower than 0.0765 Ah (2.46%) over all of our experiments.
MoreTranslated text
Key words
Battery management systems,electric vehicles (EVs),recurrent neural networks (RNNs)
AI Read Science
Must-Reading Tree
Example
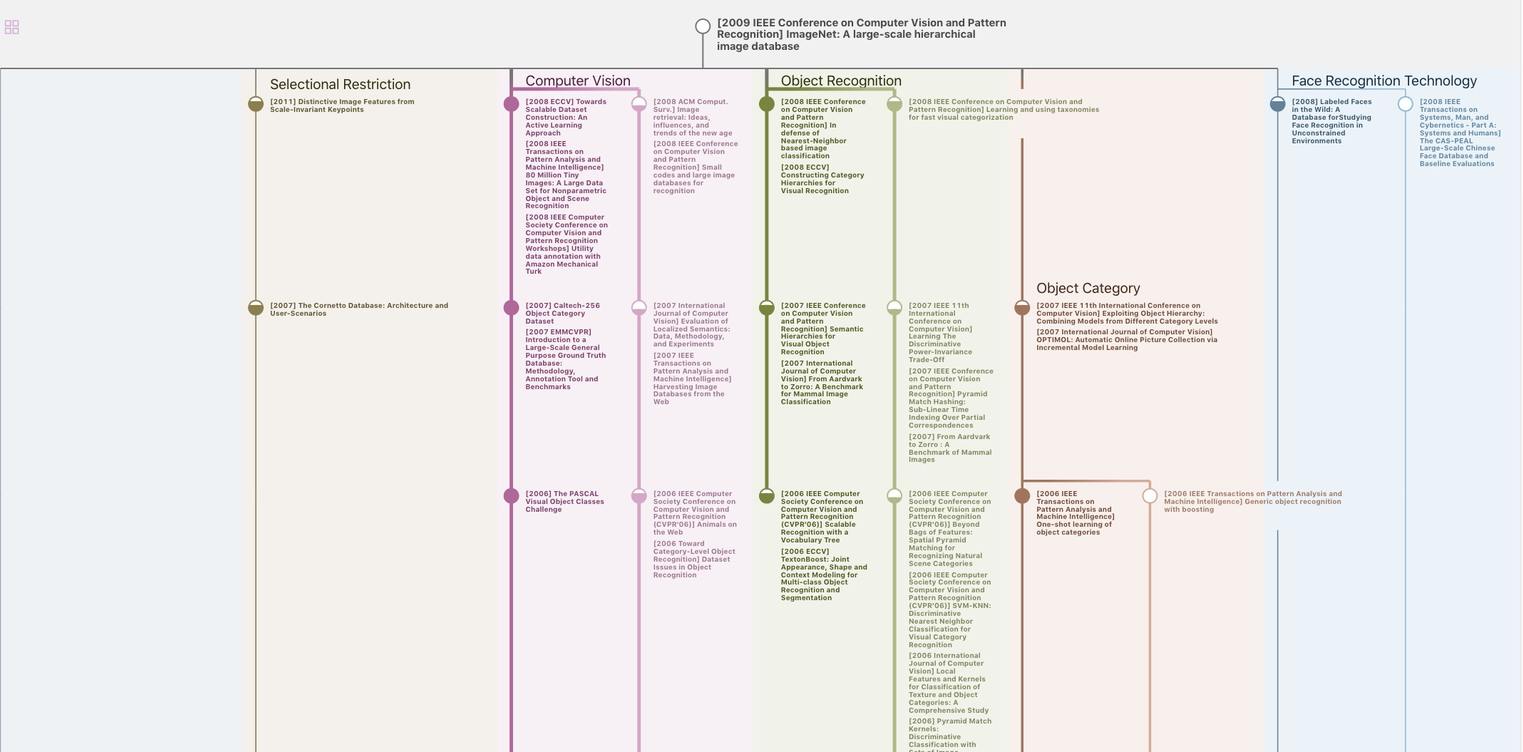
Generate MRT to find the research sequence of this paper
Chat Paper
Summary is being generated by the instructions you defined