Entropy-based Detection of Botnet Command and Control
Proceedings of the Australasian Computer Science Week Multiconference(2017)
摘要
Any malware designed to be controlled by a remote system (as required for a botnet) must establish command and control (C&C) with that system. As part of establishing and maintaining C&C, botnet malware will often send regular messages to the locations of potential C&C servers, a practice known as beaconing. To avoid detection of these beacons, this traffic will be obfuscated and encrypted. Security researchers have had success with detecting beaconing by looking for traffic at regular time intervals, but malware authors are now introducing variation in these time intervals to foil this. Our research proposes that the regularity of both communication time intervals and data size are a useful feature set for detecting these C&C channels. To quantify the regularity of these streams of data, we use a nearest-neighbour estimate of entropy that is simple and quick to calculate. We then measure mean, standard deviation and entropy for time intervals and data sizes of a series of connections between a source and a destination. These features are then used as input to a support vector machine which is trained to detect botnet C&C activity. This approach was tested on the ISOT dataset---a dataset containing a mix of both benign and malicious real-world traffic. Using these features, we were able to train this classifier to accurately detect a large proportion of the botnet traffic.
更多查看译文
AI 理解论文
溯源树
样例
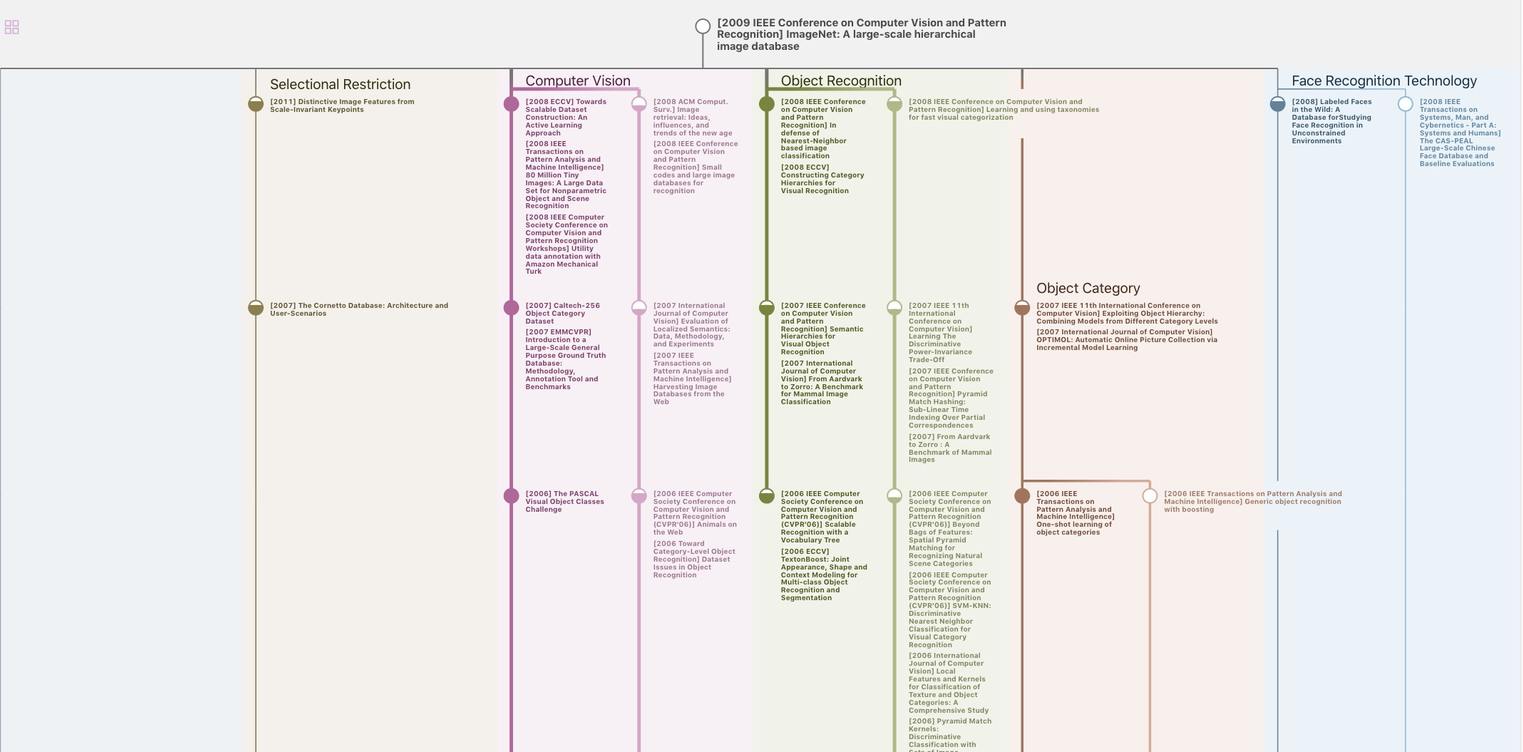
生成溯源树,研究论文发展脉络
Chat Paper
正在生成论文摘要