Phase Transitions in Parameter Rich Optimization Problems.
ANALCO(2017)
摘要
Most real world combinatorial optimization problems are affected by noise in the input data. Search algorithms to identify “good” solutions with low costs behave like the dynamics of large disordered particle systems, e.g., random networks or spin glasses. Such solutions to noise perturbed optimization problems are characterized by Gibbs distributions when the optimization algorithm searches for typical solutions by stochastically minimizing costs. The free energy that determines the normalization of the Gibbs distribution balances cost minimization relative to entropy maximization.The problem to analytically compute the free energy of disordered systems has been known as a notoriously difficult mathematical challenge for at least half a century (Talagrand, 2003). We provide rigorous, matching upper and lower bounds on the free energy for two disordered combinatorial optimization problems of random graph instances, the sparse Minimum Bisection Problem (sMBP) and Lawler's Quadratic Assignment Problem (LQAP). These two problems exhibit phase transitions that are equivalent to the statistical behavior of Derrida's Random Energy Model (REM). Both optimization problems can be characterized as parameter rich since individual solutions depend on more parameters than the logarithm of the solution space cardinality would suggest for e.g. a coordinate representation.
更多查看译文
关键词
Gathering Algorithms,Geometric Optimization,Approximation Algorithms,Online Algorithms
AI 理解论文
溯源树
样例
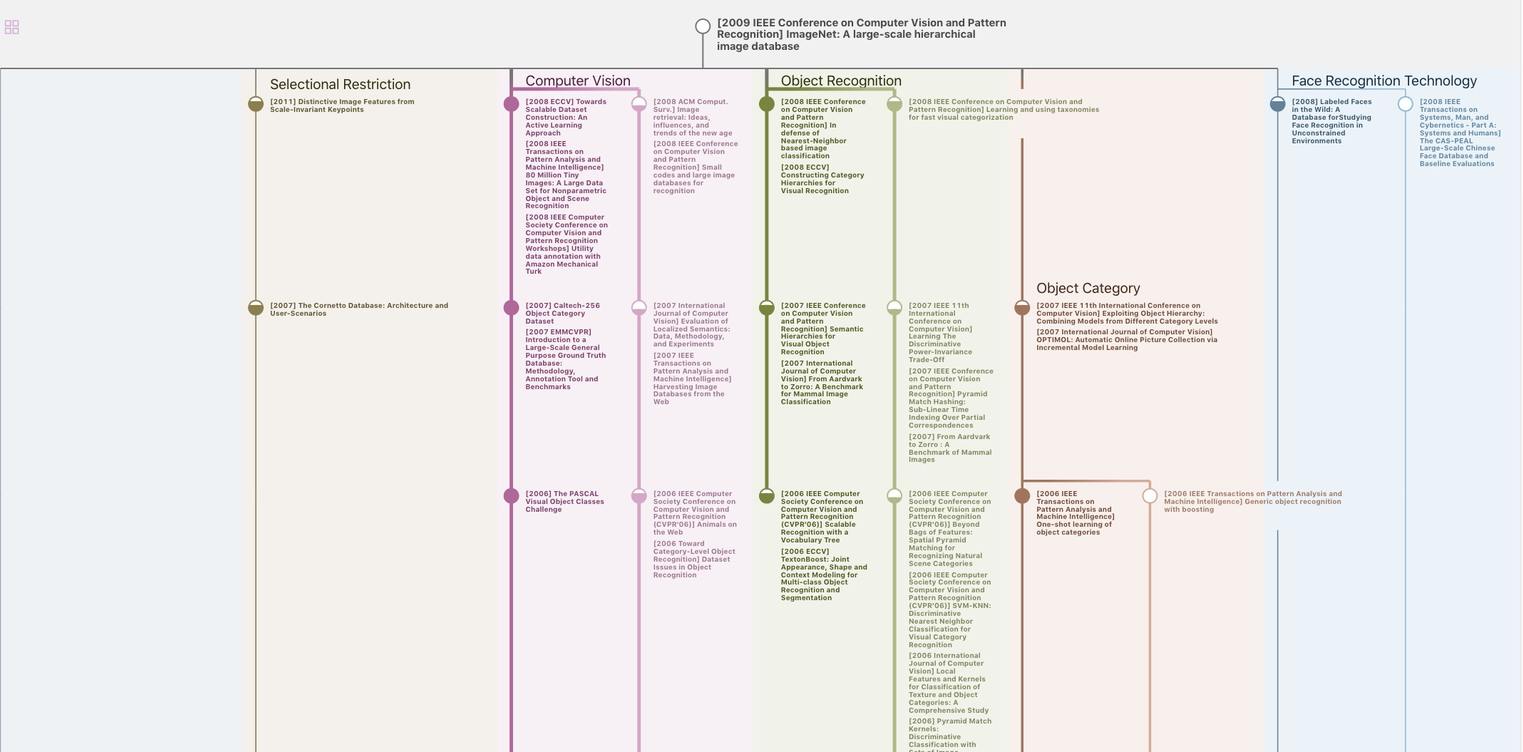
生成溯源树,研究论文发展脉络
Chat Paper
正在生成论文摘要