Comparative evaluation of big-data systems on scientific image analytics workloads
Hosted Content(2017)
摘要
AbstractScientific discoveries are increasingly driven by analyzing large volumes of image data. Many new libraries and specialized database management systems (DBMSs) have emerged to support such tasks. It is unclear how well these systems support real-world image analysis use cases, and how performant the image analytics tasks implemented on top of such systems are. In this paper, we present the first comprehensive evaluation of large-scale image analysis systems using two real-world scientific image data processing use cases. We evaluate five representative systems (SciDB, Myria, Spark, Dask, and TensorFlow) and find that each of them has shortcomings that complicate implementation or hurt performance. Such shortcomings lead to new research opportunities in making large-scale image analysis both efficient and easy to use.
更多查看译文
AI 理解论文
溯源树
样例
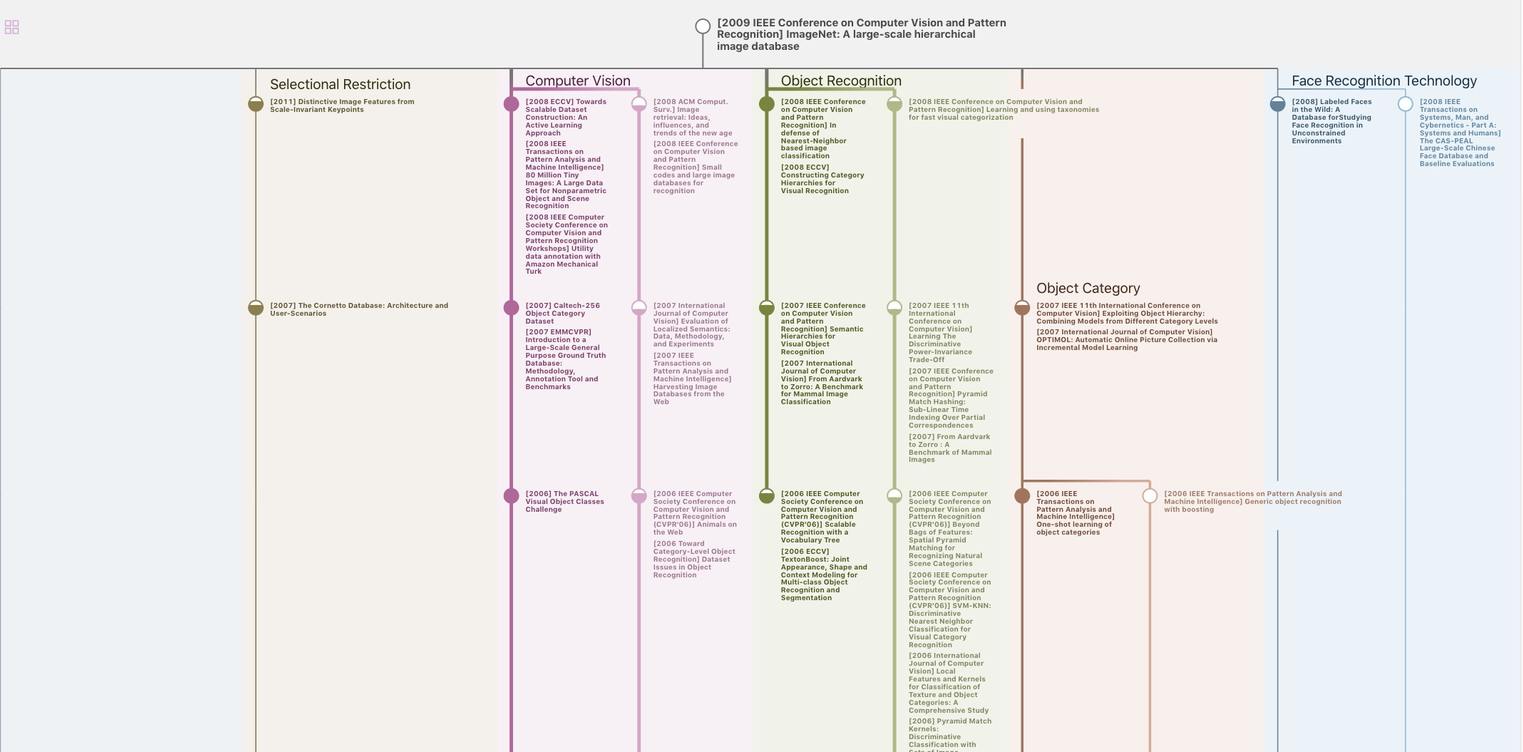
生成溯源树,研究论文发展脉络
Chat Paper
正在生成论文摘要