Supernovae Detection By Using Convolutional Neural Networks
2016 INTERNATIONAL JOINT CONFERENCE ON NEURAL NETWORKS (IJCNN)(2016)
摘要
During the last couple of years Astronomy, as many other fields, has been facing the problem of automatic processing of massive data. This has been primarily driven by large survey instruments, which scan large areas of the sky aiming to catalog everything they find. The High Cadence Transient Survey (HiTS) is one of these surveys that searches for phenomena of limited duration (such as supernovae) called transients. The HiTS pipeline produces transient candidates by subtracting science images from a template. In this paper we present a convolutional neural network (ConvNet) approach to classify sources found in the difference images as real transients or artifacts. We compare our results against a random forest classification model that has been created by the HiTS team during the last two years through feature engineering. Our ConvNet model obtains features automatically and obtains a 99.32% accuracy as compared to the previous 98.89% accuracy from the random forest model. This approach will be very useful when processing data from next generation instruments such as the Large Synoptic Survey Telescope (LSST).
更多查看译文
关键词
supernovae detection,convolutional neural networks,astronomy,High Cadence Transient Survey,HiTS,science images,ConvNet,sources classification,difference images,random forest classification model,feature engineering,large synoptic survey telescope,LSST
AI 理解论文
溯源树
样例
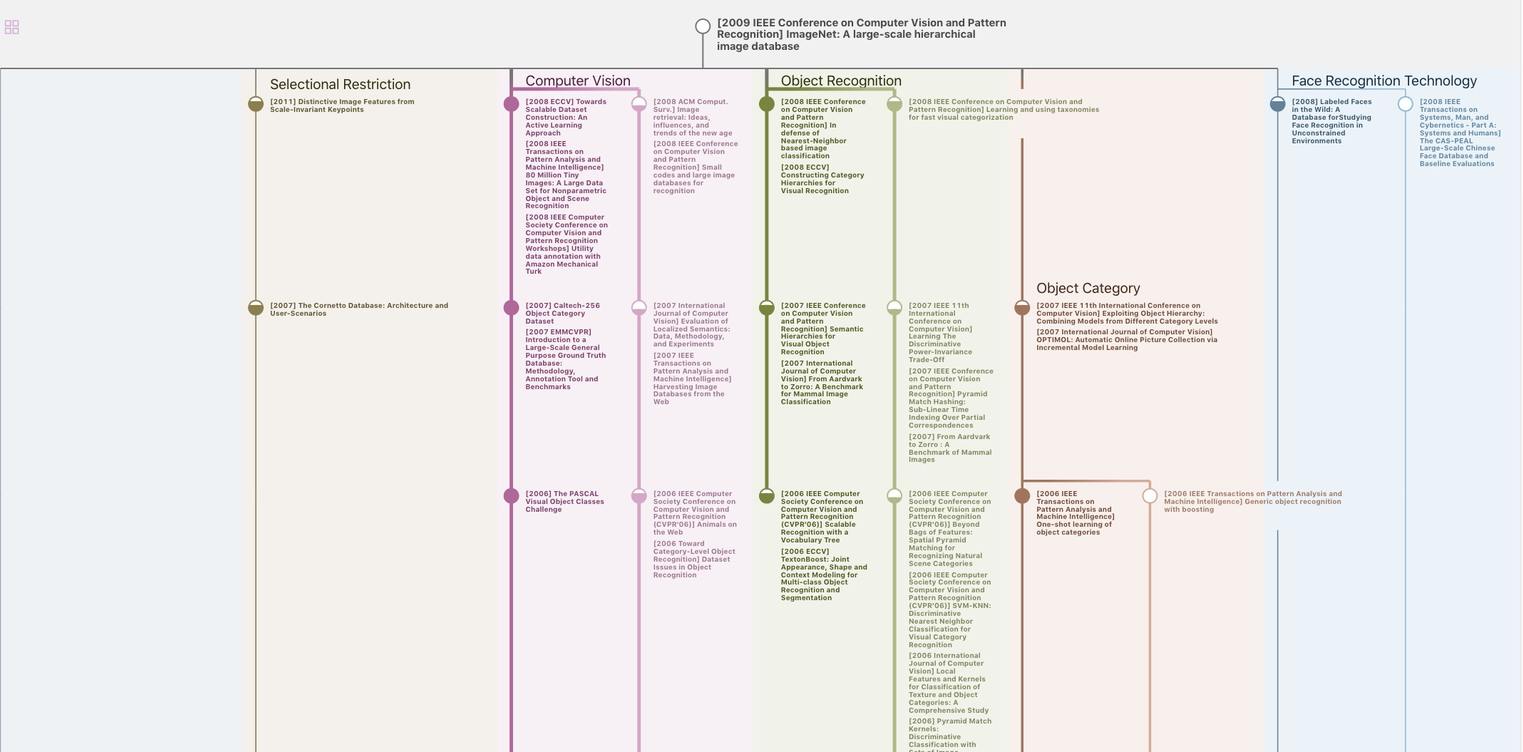
生成溯源树,研究论文发展脉络
Chat Paper
正在生成论文摘要