Learning Deep Classifiers With Deep Features
2016 IEEE INTERNATIONAL CONFERENCE ON MULTIMEDIA & EXPO (ICME)(2016)
摘要
Visual separability between different objects in various image classification tasks is highly uneven. As a consequence, humans need different levels of detailed descriptions to separate objects in multi-granularity similarities. Meanwhile, deep networks, such as convolutional neural networks (CNNs) have demonstrated great ability in multilevel representations for an object. Unfortunately, existing methods with deep networks in classification typically use the output of the last layer as the only feature to train flat N-way classifiers, which fail to fit the multi-granularity character. In this paper, by regarding different CNN layers as multiple levels of abstraction, we propose a deep decision tree (DDT) to distinguish objects sharing great appearance similarities with utilizing features in all layers. First, deep features in multiple layers are extracted from deep networks as the input for building a DDT. Next, in the training phase, the features from earlier layers are selected for splitting on a deeper node. Finally, multiple DDTs are bagged to make the final prediction by taking the majority vote. The experimental results in two datasets show DDT can greatly improve the classification accuracy in multi-grained tasks than flat models.
更多查看译文
关键词
Convolutional neural networks,deep classifier,deep decision tree,multi-grained classification
AI 理解论文
溯源树
样例
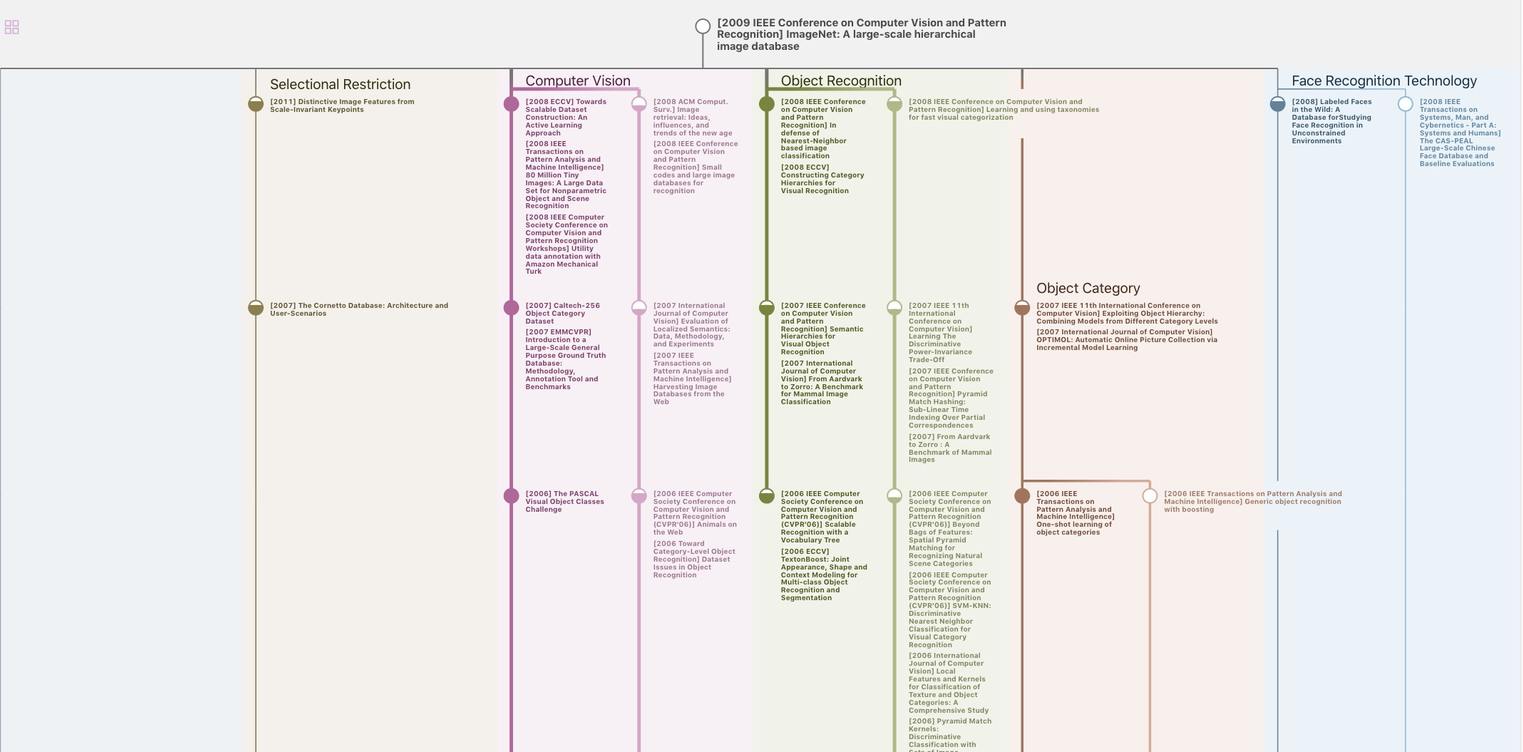
生成溯源树,研究论文发展脉络
Chat Paper
正在生成论文摘要