Deep Learning for Human Part Discovery in Images.
IEEE International Conference on Robotics and Automation(2016)
摘要
This paper addresses the problem of human body part segmentation in conventional RGB images, which has several applications in robotics, such as learning from demonstration and human-robot handovers. The proposed solution is based on Convolutional Neural Networks (CNNs). We present a network architecture that assigns each pixel to one of a predefined set of human body part classes, such as head, torso, arms, legs. After initializing weights with a very deep convolutional network for image classification, the network can be trained end-to-end and yields precise class predictions at the original input resolution. Our architecture particularly improves on over-fitting issues in the up-convolutional part of the network. Relying only on RGB rather than RGB-D images also allows us to apply the approach outdoors. The network achieves state-of-the-art performance on the PASCAL Parts dataset. Moreover, we introduce two new part segmentation datasets, the Freiburg sitting people dataset and the Freiburg people in disaster dataset. We also present results obtained with a ground robot and an unmanned aerial vehicle.
更多查看译文
关键词
deep learning,human part discovery,human body part segmentation,RGB images,convolutional neural networks,CNN,network architecture,deep convolutional network,image classification,PASCAL parts dataset,part segmentation datasets,Freiburg sitting people dataset,disaster dataset,unmanned aerial vehicle
AI 理解论文
溯源树
样例
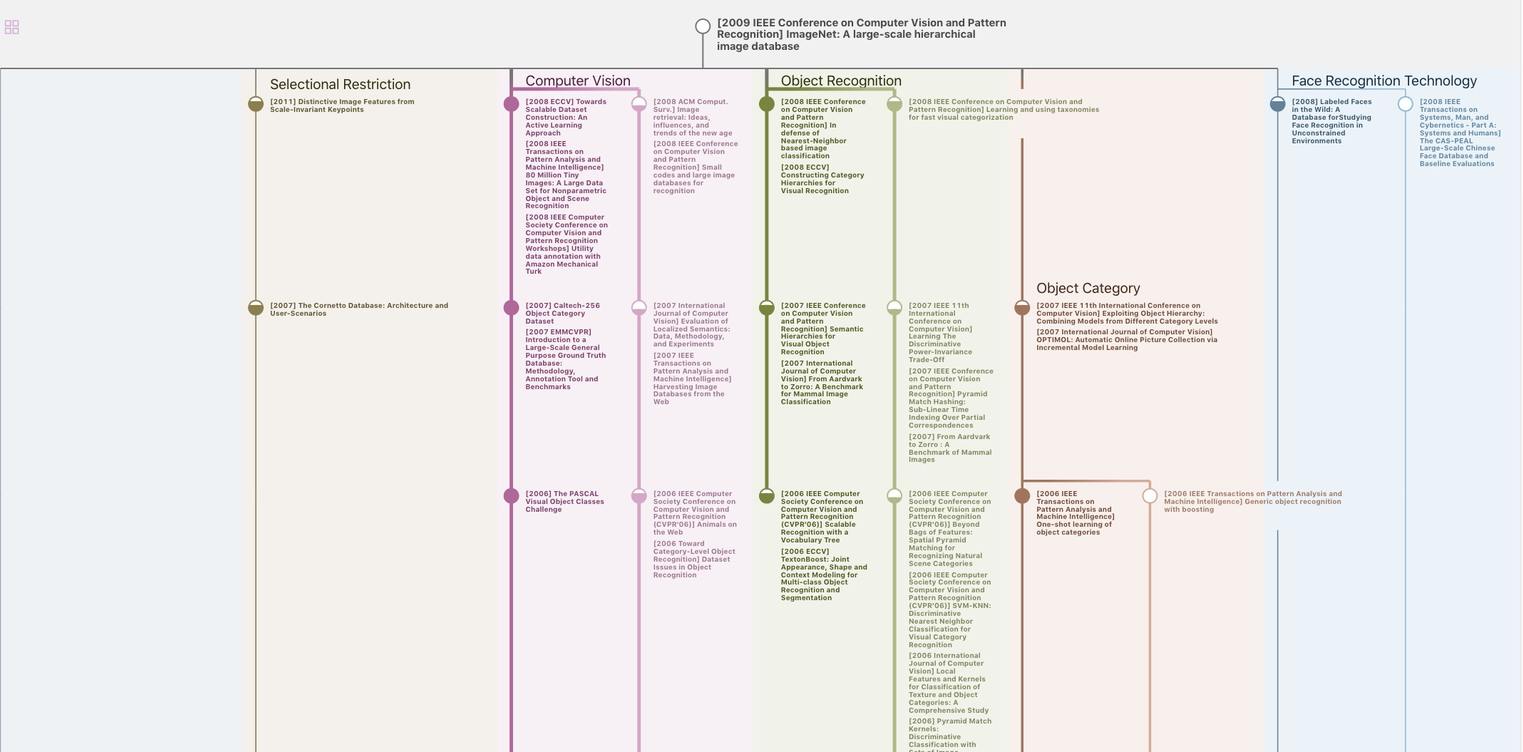
生成溯源树,研究论文发展脉络
Chat Paper
正在生成论文摘要