Plug-and-Play ADMM for Image Restoration: Fixed-Point Convergence and Applications
IEEE transactions on computational imaging(2017)
摘要
Alternating direction method of multiplier (ADMM) is a widely used algorithm for solving constrained optimization problems in image restoration. Among many useful features, one critical feature of the ADMM algorithm is its modular structure which allows one to plug in any off-the-shelf image denoising algorithm for a subproblem in the ADMM algorithm. Because of the plug-in nature, this type of ADMM algorithms is coined the name "Plug-and-Play ADMM". Plug-and-Play ADMM has demonstrated promising empirical results in a number of recent papers. However, it is unclear under what conditions and by using what denoising algorithms would it guarantee convergence. Also, since Plug-and-Play ADMM uses a specific way to split the variables, it is unclear if fast implementation can be made for common Gaussian and Poissonian image restoration problems. In this paper, we propose a Plug-and-Play ADMM algorithm with provable fixed point convergence. We show that for any denoising algorithm satisfying an asymptotic criteria, called bounded denoisers, Plug-and-Play ADMM converges to a fixed point under a continuation scheme. We also present fast implementations for two image restoration problems on super-resolution and single-photon imaging. We compare Plug-and-Play ADMM with state-of-the-art algorithms in each problem type, and demonstrate promising experimental results of the algorithm.
更多查看译文
关键词
ADMM,deblurring,denoising,image restoration,inpainting,Plug-and-Play,Poisson noise,single photon imaging,super-resolution
AI 理解论文
溯源树
样例
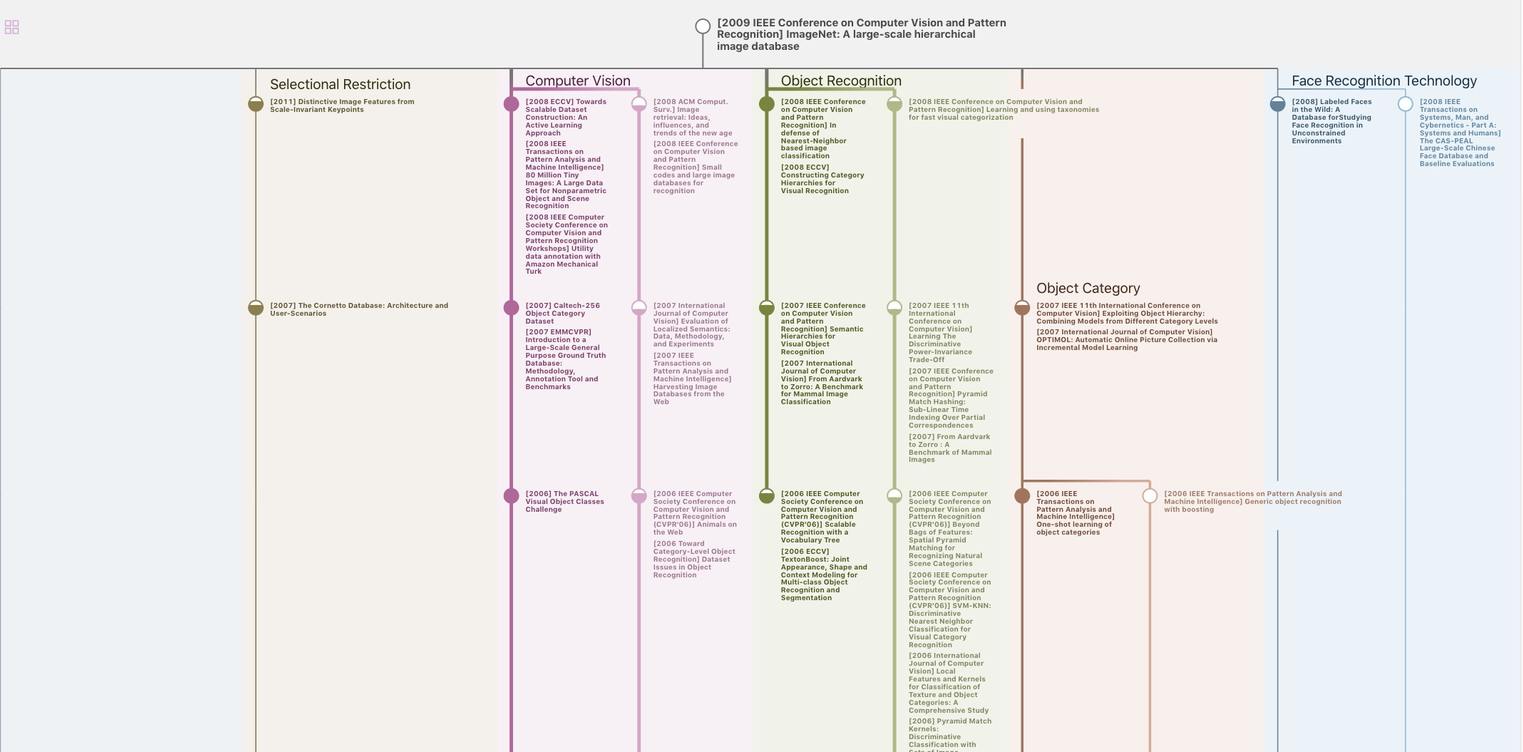
生成溯源树,研究论文发展脉络
Chat Paper
正在生成论文摘要