Efficient Unconditionally Secure Comparison and Privacy Preserving Machine Learning Classification Protocols.
ProvSec(2015)
摘要
We propose an efficient unconditionally secure protocol for privacy preserving comparison of $$\\ell $$ℓ-bit integers when both integers are shared between two semi-honest parties. Using our comparison protocol as a building block, we construct two-party generic private machine learning classifiers. In this scenario, one party holds an input while the other holds a model and they wish to classify the input according to the model without revealing their private information to each other. Our constructions are based on the setup assumption that there exists pre-distributed correlated randomness available to the computing parties, the so-called commodity-based model. The protocols are storage and computationally efficient, consisting only of additions and multiplications of integers.
更多查看译文
关键词
Secure comparison, Private machine learning, Unconditional security, Commodity based model
AI 理解论文
溯源树
样例
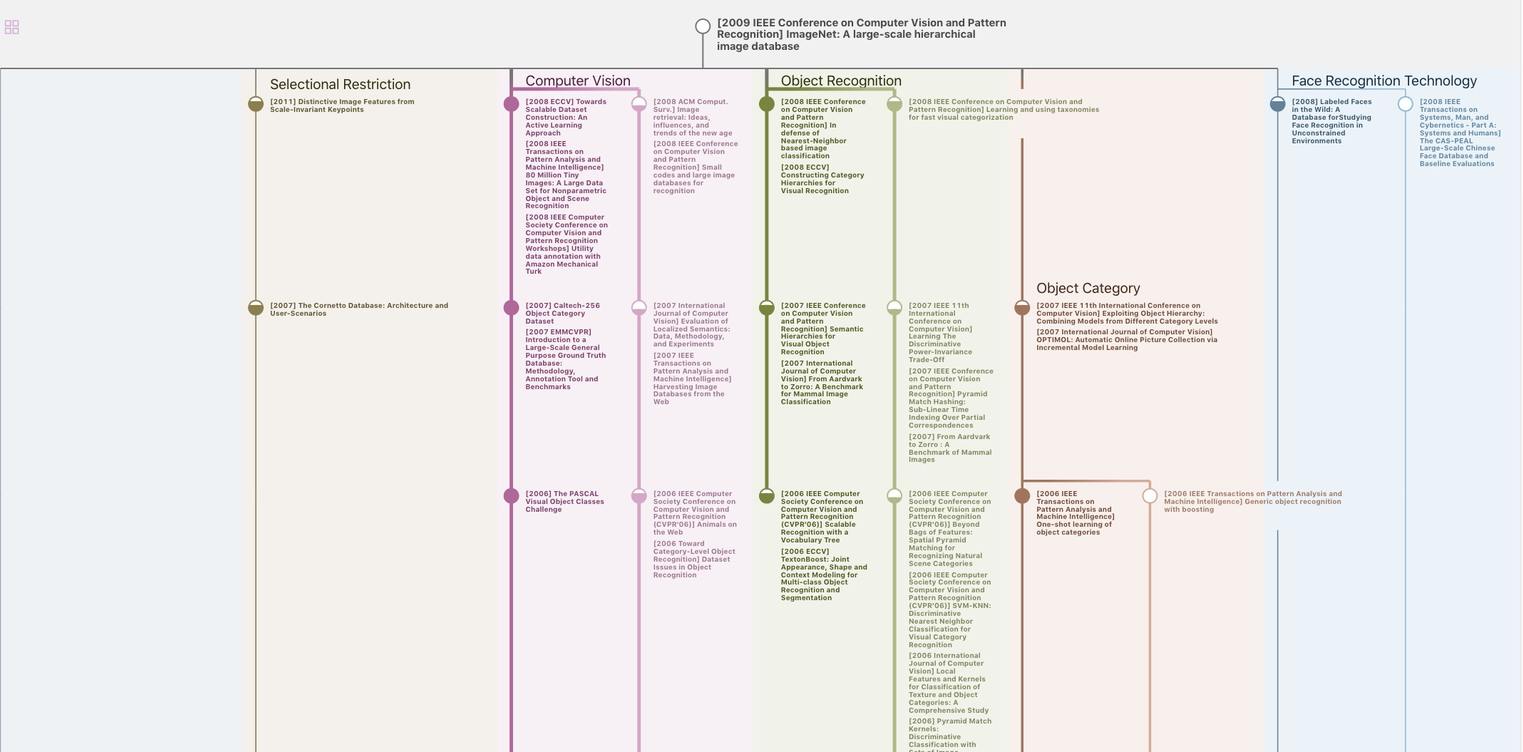
生成溯源树,研究论文发展脉络
Chat Paper
正在生成论文摘要