Multitask learning for Laban movement analysis
MOCO(2015)
摘要
This paper presents the results of a multitask learning method for recognition of Laban Movement Analysis (LMA) qualities from a markerless motion capture camera. LMA is a well-accepted method for describing, interpreting and documenting human movement which can be advantageous over kinematic description for capturing qualitative aspects as well as quantitative ones. Its specific language can be understood across disciplines. Thus, in recent years, LMA is increasingly becoming the preferred method for movement analysis. Many applications that use motion capture data might be significantly leveraged by automatic recognition of Laban Movement qualities. A data set of 550 video clips of different combinations of LMA qualities were recorded from markerless motion capture skeletal recordings demonstrated on the output of Microsoft's Kinect V2 sensor and on video. A sample of these clips were tagged by 2 Certified Movement Analysts as a multi-label training set to develop the Machine Learning (ML) algorithms. This approach obtained an improvement in recall and precision rate of about 60%--- 4% more than single-task machine learning previous approach by Bertstein et al. on single-task learning, was validated by analysis of non trained people moving general actions. Results show improved handling of noisy sensory data with an in-home setup, a method for automatic recognition of markerless movement in different situations, postures and tasks, and moderate improvements in quantification of subtle qualities for which a well defined quantification had previously not been found.
更多查看译文
AI 理解论文
溯源树
样例
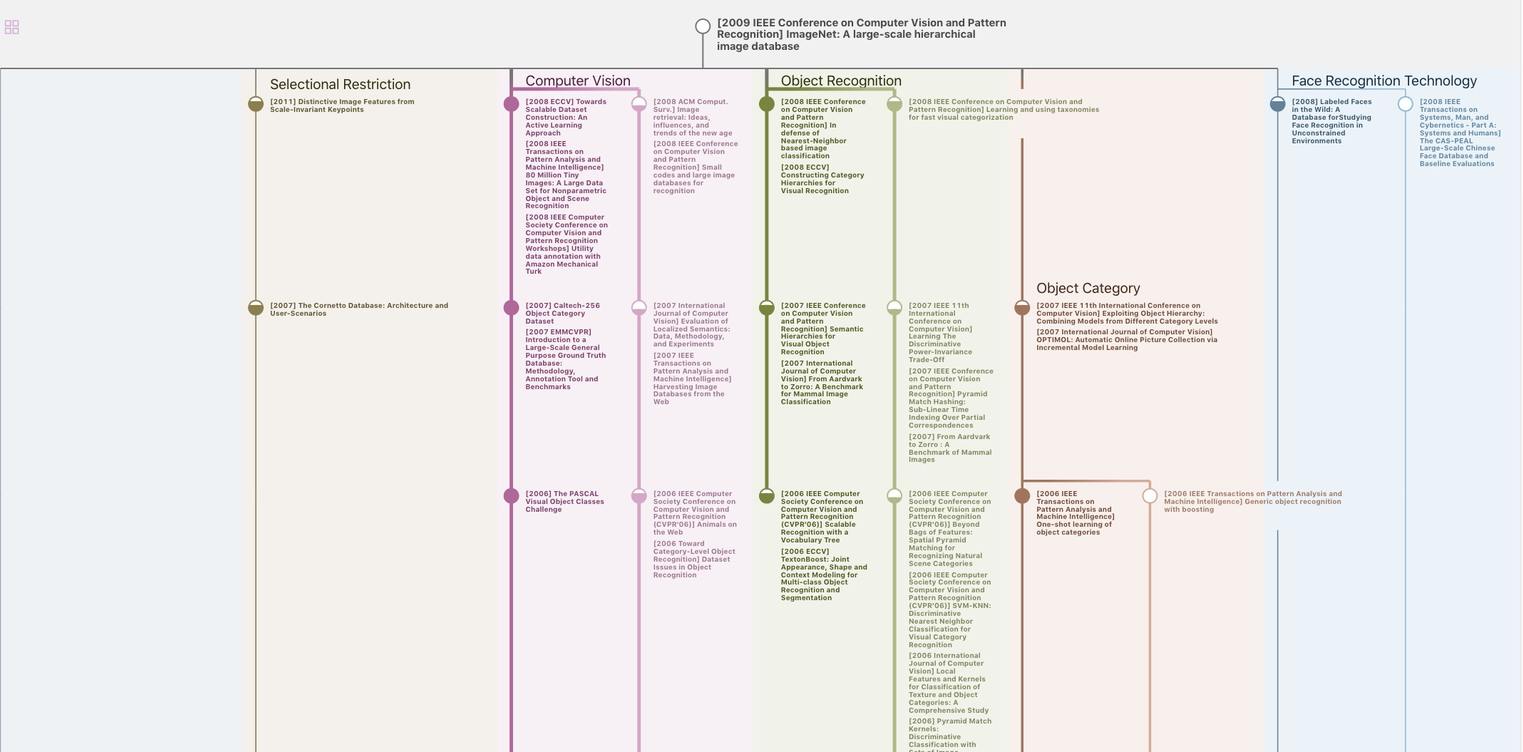
生成溯源树,研究论文发展脉络
Chat Paper
正在生成论文摘要