The Precision-Recall Plot is More Informative Than the ROC Plot when Evaluating Binary Classifiers on Imbalanced Datasets.
PLoS ONE(2015)SCI 3区
- Pretraining has recently greatly promoted the development of natural language processing (NLP)
- We show that M6 outperforms the baselines in multimodal downstream tasks, and the large M6 with 10 parameters can reach a better performance
- We propose a method called M6 that is able to process information of multiple modalities and perform both single-modal and cross-modal understanding and generation
- The model is scaled to large model with 10 billion parameters with sophisticated deployment, and the 10 -parameter M6-large is the largest pretrained model in Chinese
- Experimental results show that our proposed M6 outperforms the baseline in a number of downstream tasks concerning both single modality and multiple modalities We will continue the pretraining of extremely large models by increasing data to explore the limit of its performance
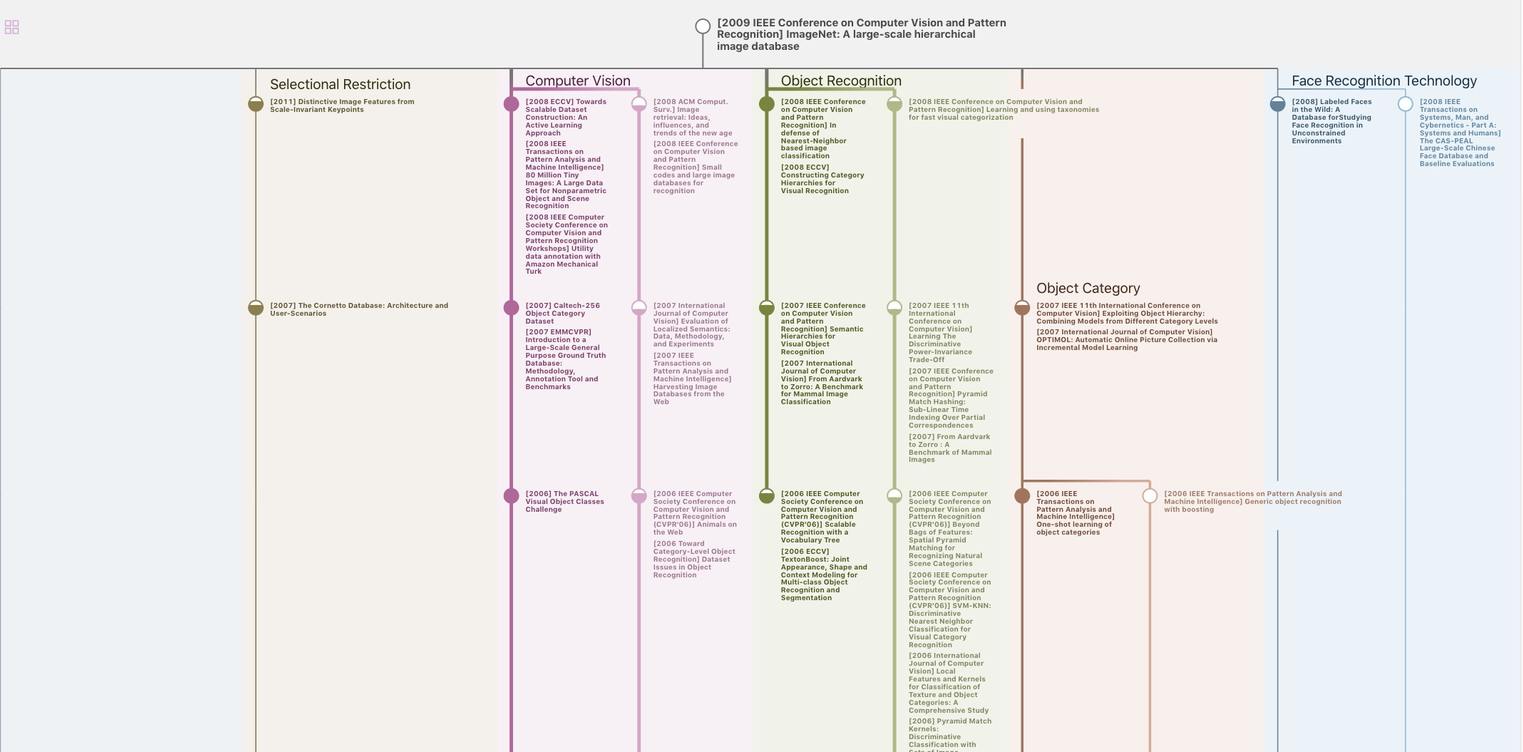
Crafting Adversarial Input Sequences for Recurrent Neural Networks
被引用301
Adversarial Examples in the Physical World.
被引用7261
Safety Verification of Deep Neural Networks.
被引用1195
Assessing Threat of Adversarial Examples on Deep Neural Networks.
被引用58
Universal Adversarial Perturbations Against Semantic Image Segmentation.
被引用378
Adversarial Image Perturbation for Privacy Protection - A Game Theory Perspective.
被引用96
被引用11
Adversarial Examples for Evaluating Reading Comprehension Systems.
被引用1848
Foolbox v0.8.0: A Python toolbox to benchmark the robustness of machine learning models.
被引用253
被引用24
Certifying Some Distributional Robustness with Principled Adversarial Training.
被引用1224
Feature-Guided Black-Box Safety Testing of Deep Neural Networks.
被引用137
Attack and Defense of Dynamic Analysis-Based, Adversarial Neural Malware Detection Models.
被引用25
Deflecting Adversarial Attacks with Pixel Deflection
被引用409
TwinNet: A Double Sub-Network Framework for Detecting Universal Adversarial Perturbations
被引用9
Learning to Attack: Adversarial Transformation Networks
被引用206
Deep Networks with RBF Layers to Prevent Adversarial Examples
被引用10
被引用61
Practical Black-Box Attacks on Deep Neural Networks Using Efficient Query Mechanisms
被引用316
How to Steal a Machine Learning Classifier with Deep Learning
被引用60