Nonnegative Mixture for Underdetermined Blind Source Separation Based on a Tensor Algorithm
Circuits, Systems, and Signal Processing(2015)
Abstract
In this study, a tensor algorithm is proposed to blindly separate an instantaneous linear underdetermined mixture with non-stationary sources and nonnegative mixing matrix. It proceeds in two steps: 1) estimating the mixing matrix and 2) recovering the source signals. First, a canonical tensor model is constructed using a fourth-order cumulant tensor of the observed signals to estimate the mixing matrix. Then, an improved hierarchical alternating least squares algorithm is used to decompose the canonical tensor model, which ensures that all elements of the mixing matrix are positive. Finally, the sources are recovered using a minimum mean-squared error beamformer approach without any hypothetical limitation. We apply two classes of data (speech signals and biomedical signals) to substantiate the effectiveness of the proposed algorithm for underdetermined blind source separation.
MoreTranslated text
Key words
Underdetermined blind source separation,Hierarchical alternating least squares,Canonical decomposition,Minimum mean-squared error beamformer
AI Read Science
Must-Reading Tree
Example
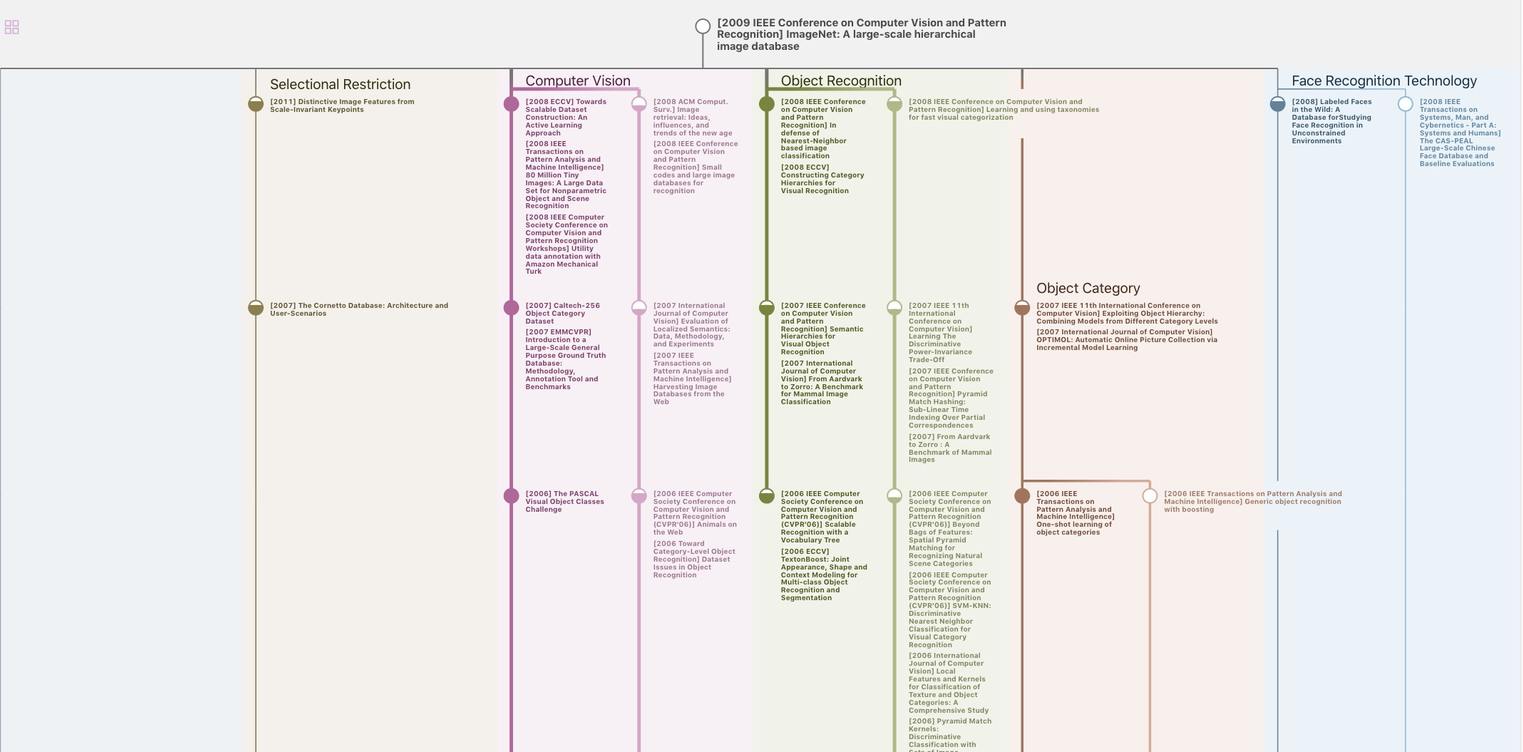
Generate MRT to find the research sequence of this paper
Chat Paper
Summary is being generated by the instructions you defined