Estimating Consensus and Associated Uncertainty Between Inherently Different Species Distribution Models
Methods in ecology and evolution(2013)
Abstract
Summary Forecasting shifts in biome and species distribution is crucially needed in the current context of global change. So far, most projections of vegetation distribution rely on correlative species distribution models (SDMs). Yet, process‐based or hybrid models based on explicit physiological description may be more robust to extrapolation under future climatic conditions. Differences between model projections may be wide, leading to scepticism among environmental stakeholders. Here, we propose to combine outputs of several distribution models based on physiological responses, to produce both consensual maps of occurrences and maps of associated uncertainty. The consensus map relies on the conditional projections of each SDM. Because the models used are based on processes, their errors are likely to vary consistently with climate as some processes not implemented in a model might be important under a given set of climatic conditions. Uncertainty of the consensus model is thus assessed through multimodel regression of deviance maps with respect to current climatic conditions, and can be extrapolated to forecast climates. We illustrate this approach using three SDMs, on three widely distributed European trees (Fagus sylvatica L., Quercus robur L. and Pinus sylvestris L.), and project their distributions under two scenarios. The conditional consensus outperforms classical methods of model consensus (i.e. to use the mean, the median or a weighted average of individual SDM outputs) in projecting current occurrences. Consistently, with the results of individual SDMs, the conditional consensus projects that the suitable areas for F. sylvatica and Q. robur will expand towards north‐eastern Europe, while that of P. sylvestris will contract. Projections of future occurrence are most uncertain towards the margins of the distribution (particularly the trailing edge). Our approach can help modellers identify the limitations of each SDM and stakeholders pinpoint the regions of models agreement and highest certainty.
MoreTranslated text
Key words
climate change,correlative distribution models,environmental clustering of model errors,Fagus sylvatica,likelihood,model assessment,Pinus sylvestris,process-based distribution models,Quercus robur
AI Read Science
Must-Reading Tree
Example
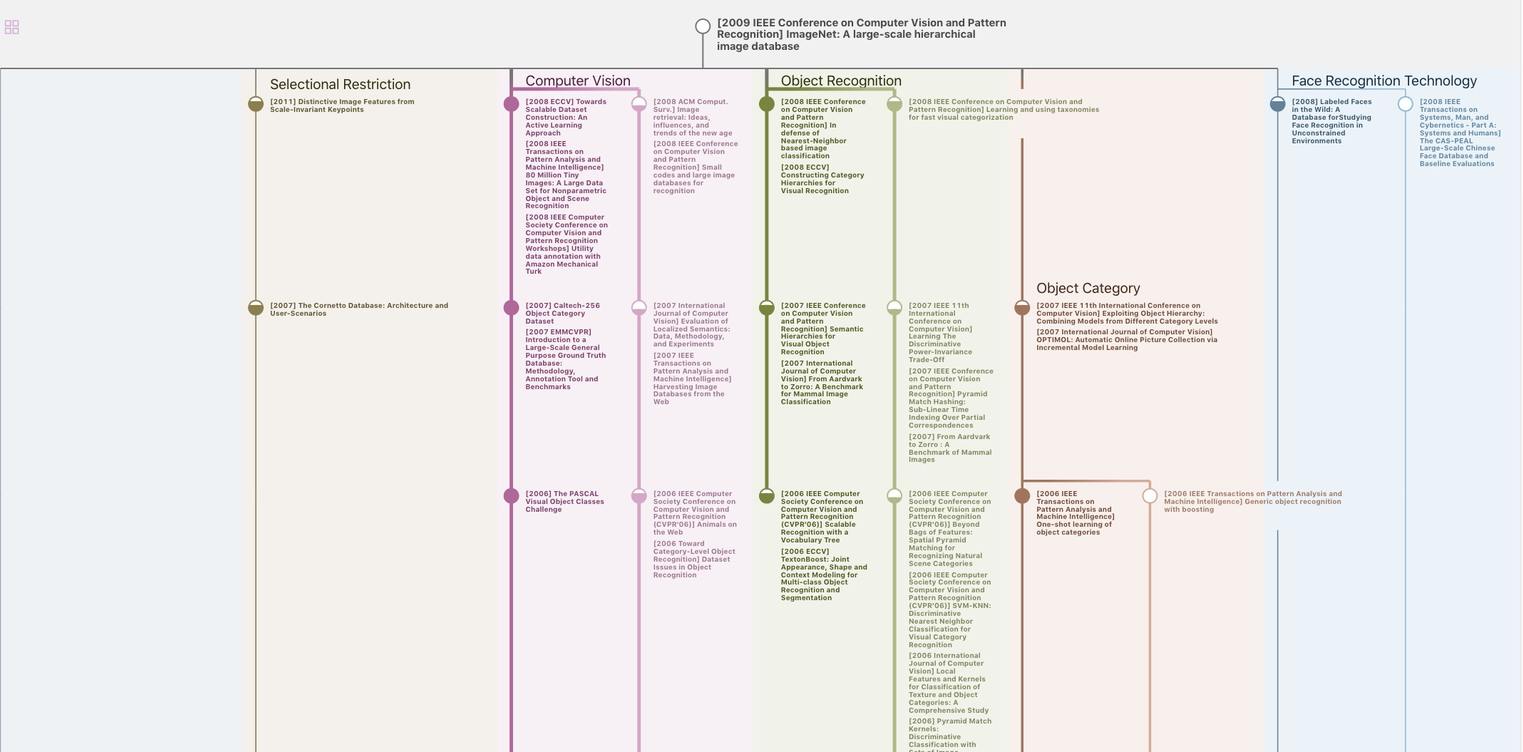
Generate MRT to find the research sequence of this paper
Chat Paper
Summary is being generated by the instructions you defined