Improvement of Automatic Scaling of Vertical Incidence Ionograms by Simulated Annealing
Journal of Atmospheric and Solar-Terrestrial Physics(2015)SCI 4区
Wuhan Univ
Abstract
The ionogram autoscaling technique is very important for facilitating the statistical investigation of the ionosphere. Jiang et al. (2013) proposed an autoscaling technique for extracting ionospheric characteristics from vertical incidence ionograms. However, extensive efforts are invested in continuously improving the performance of that. The simulated annealing (SA) is used to improve the autoscaling technique in this paper. To be capable of automatic scaling of ionograms recorded at different locations, the SA is applied instead of Empirical Orthogonal Functions (EOFs) to search the best-fit parameters in the autoscaling technique. In order to validate the improvement of this autoscaling technique, ionograms recorded at Wuhan (30.5°N, 114.3°E), Puer (22.7°N, 101.05°E) and Leshan (29.6°N, 103.75°E) are investigated by comparing the autoscaled results with the values scaled by an operator. Results show that the presented work is efficient for scaling of ionograms recorded at different geographic positions. Moreover, the additional procedure can improve the accuracy of the autoscaling technique compared to results presented by Jiang et al. (2013).
MoreTranslated text
Key words
Ionogram,Automatic scaling,Simulated annealing,Ionosphere
PDF
View via Publisher
AI Read Science
AI Summary
AI Summary is the key point extracted automatically understanding the full text of the paper, including the background, methods, results, conclusions, icons and other key content, so that you can get the outline of the paper at a glance.
Example
Background
Key content
Introduction
Methods
Results
Related work
Fund
Key content
- Pretraining has recently greatly promoted the development of natural language processing (NLP)
- We show that M6 outperforms the baselines in multimodal downstream tasks, and the large M6 with 10 parameters can reach a better performance
- We propose a method called M6 that is able to process information of multiple modalities and perform both single-modal and cross-modal understanding and generation
- The model is scaled to large model with 10 billion parameters with sophisticated deployment, and the 10 -parameter M6-large is the largest pretrained model in Chinese
- Experimental results show that our proposed M6 outperforms the baseline in a number of downstream tasks concerning both single modality and multiple modalities We will continue the pretraining of extremely large models by increasing data to explore the limit of its performance
Try using models to generate summary,it takes about 60s
Must-Reading Tree
Example
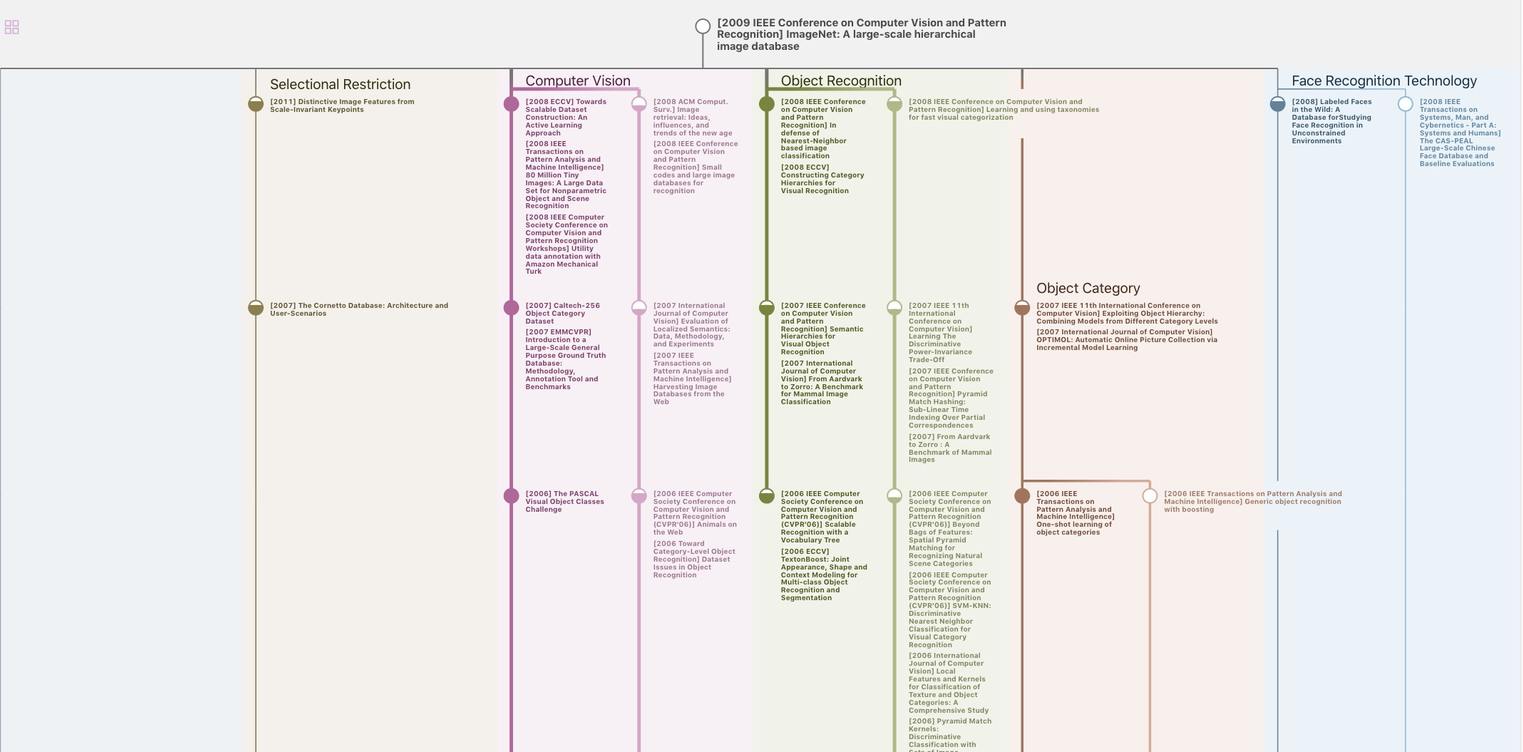
Generate MRT to find the research sequence of this paper
Related Papers
Acta Geophysica 2017
被引用9
Wuhan Ionospheric Oblique Backscattering Sounding System and Its Applications - A Review.
Italian National Conference on Sensors 2017
被引用21
Design and Application of the Digital Multifunctional Ionosonde
IET Radar Sonar & Navigation 2016
被引用4
Advances in Space Research 2016
被引用10
Software for Scaling and Analysis of Vertical Incidence Ionograms-Ionoscaler
Advances in Space Research 2016
被引用29
RADIO SCIENCE 2016
被引用12
Histogram-based Ionogram Displays and Their Application to Autoscaling
Advances in Space Research 2017
被引用8
Radio Science 2020
被引用2
ANNALES GEOPHYSICAE 2020
被引用11
Algorithm for Automatic Scaling of the F-Layer Using Image Processing of Ionograms.
IEEE Transactions on Geoscience and Remote Sensing 2020
被引用4
ACTA ASTRONAUTICA 2021
被引用17
Accuracy Characteristics of Automatic Interpretation of Vertical Sounding Data
26th International Symposium on Atmospheric and Ocean Optics, Atmospheric Physics 2020
被引用0
Advances in Space Research 2022
被引用5
ELECTRONICS 2022
被引用0
Heliyon 2023
被引用0
Frontiers in Astronomy and Space Sciences 2024
被引用0
Data Disclaimer
The page data are from open Internet sources, cooperative publishers and automatic analysis results through AI technology. We do not make any commitments and guarantees for the validity, accuracy, correctness, reliability, completeness and timeliness of the page data. If you have any questions, please contact us by email: report@aminer.cn
Chat Paper
去 AI 文献库 对话