A Cluster Finding Algorithm Based on the Multiband Identification of Red Sequence Galaxies
Monthly Notices of the Royal Astronomical Society(2014)SCI 2区
Abstract
We present a new algorithm, CAMIRA, to identify clusters of galaxies in wide-field imaging survey data. We base our algorithm on the stellar population synthesis model to predict colours of red sequence galaxies at a given redshift for an arbitrary set of bandpass filters, with additional calibration using a sample of spectroscopic galaxies to improve the accuracy of the model prediction. We run the algorithm on similar to 11 960 deg(2) of imaging data from the Sloan Digital Sky Survey ( SDSS) Data Release 8 to construct a catalogue of 71 743 clusters in the redshift range 0.1 < z < 0.6 with richness after correcting for the incompleteness of the richness estimate greater than 20. We cross-match the cluster catalogue with external cluster catalogues to find that our photometric cluster redshift estimates are accurate with low bias and scatter, and that the corrected richness correlates well with X-ray luminosities and temperatures. We use the publicly available Canada-France-Hawaii Telescope Lensing Survey shear catalogue to calibrate the mass-richness relation from stacked weak lensing analysis. Stacked weak lensing signals are detected significantly for eight subsamples of the SDSS clusters divided by redshift and richness bins, which are then compared with model predictions including miscentring effects to constrain mean halo masses of individual bins. We find the richness correlates well with the halo mass, such that the corrected richness limit of 20 corresponds to the cluster virial mass limit of about 1 x 10(14) h(-1) M-circle dot for the SDSS DR8 cluster sample.
MoreTranslated text
Key words
galaxies: clusters: general
PDF
View via Publisher
AI Read Science
AI Summary
AI Summary is the key point extracted automatically understanding the full text of the paper, including the background, methods, results, conclusions, icons and other key content, so that you can get the outline of the paper at a glance.
Example
Background
Key content
Introduction
Methods
Results
Related work
Fund
Key content
- Pretraining has recently greatly promoted the development of natural language processing (NLP)
- We show that M6 outperforms the baselines in multimodal downstream tasks, and the large M6 with 10 parameters can reach a better performance
- We propose a method called M6 that is able to process information of multiple modalities and perform both single-modal and cross-modal understanding and generation
- The model is scaled to large model with 10 billion parameters with sophisticated deployment, and the 10 -parameter M6-large is the largest pretrained model in Chinese
- Experimental results show that our proposed M6 outperforms the baseline in a number of downstream tasks concerning both single modality and multiple modalities We will continue the pretraining of extremely large models by increasing data to explore the limit of its performance
Try using models to generate summary,it takes about 60s
Must-Reading Tree
Example
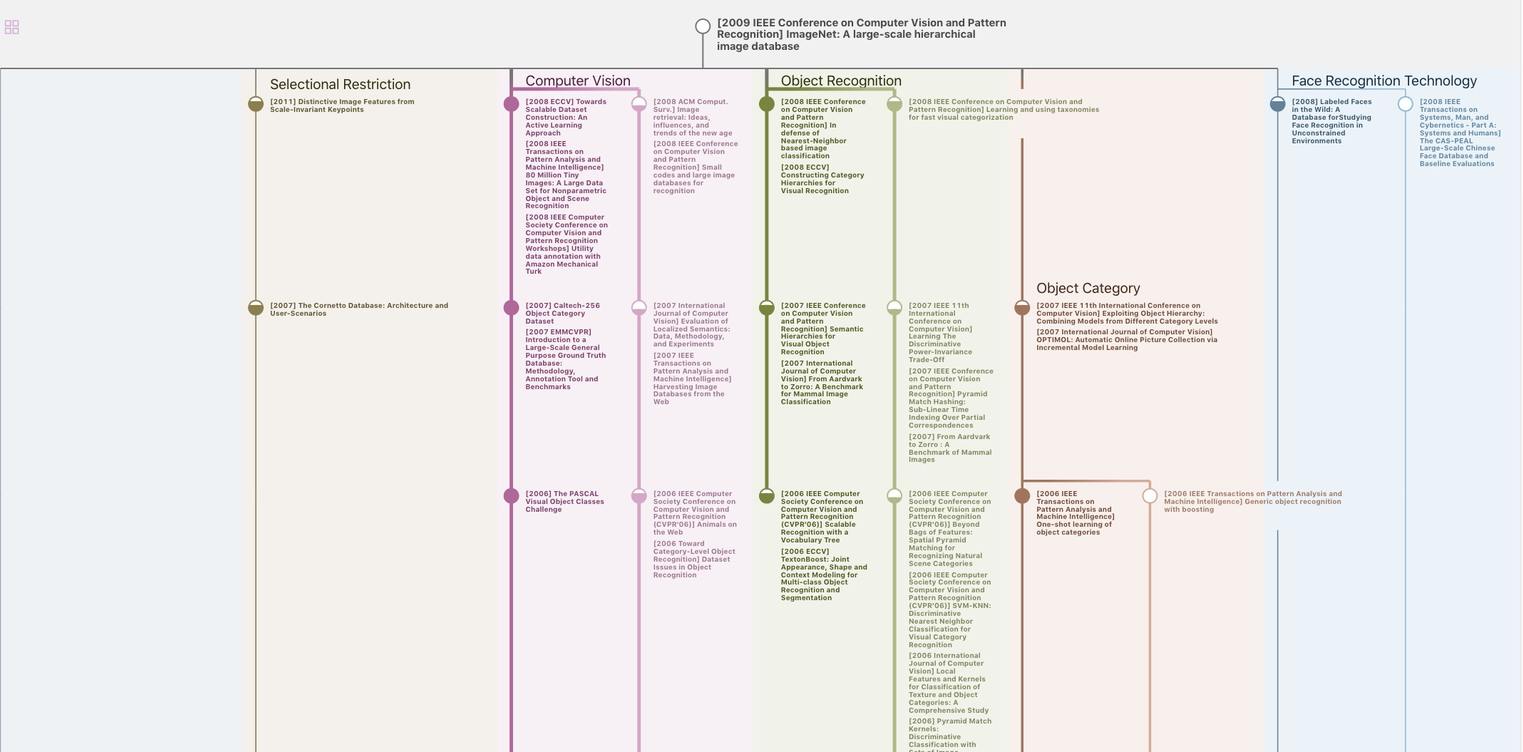
Generate MRT to find the research sequence of this paper
Related Papers
2002
被引用1945 | 浏览
2007
被引用89 | 浏览
1998
被引用17891 | 浏览
2008
被引用768 | 浏览
2009
被引用477 | 浏览
2009
被引用42 | 浏览
2008
被引用43 | 浏览
2012
被引用302 | 浏览
2013
被引用355 | 浏览
2013
被引用317 | 浏览
2011
被引用134 | 浏览
Data Disclaimer
The page data are from open Internet sources, cooperative publishers and automatic analysis results through AI technology. We do not make any commitments and guarantees for the validity, accuracy, correctness, reliability, completeness and timeliness of the page data. If you have any questions, please contact us by email: report@aminer.cn
Chat Paper
去 AI 文献库 对话